The widespread progress in the field of energy-dispersive spectrometry (EDS) provides plenty of opportunities for sophisticated analysis of low energy X-ray lines at high spatial resolution.
This is shown for cobalt nickel arsenic sulfide grains in samples from the Offset Dike of the Sudbury Igneous Complex (SIC) (Hecht et al. 2010). The Bruker M4 TORNADO Micro-XRF spectrometer was used to identify minerals of economic interest and the same were located with the aid of automated feature analysis using SEM-EDS.
Methods
A FE-SEM fitted with a QUANTAX EDS system, which also has an XFlash® 6 | 10 silicon drift detector (SDD), was used for analyzing element composition in bulk samples at high spatial resolution (pixel size < 50nm). It was possible to acquire spectrum images (HyperMap) with parameters as listed in Table 1.
The EDS databases have the entire spectra for each pixel, which benefits data mining. The element identification can be enhanced by using the maximum pixel spectrum function (Bright and Newbury, 2004).
This operation synthesizes a spectrum comprising the maximum count level located in each spectrum energy channel. It is possible to identify even elements that are present in a few or just one pixel of an element map.
Table 1. Analytical conditions for spectrum imaging (HyperMap)
tet |
Worthington dike |
Parkin dike |
Accelerating voltage / kV |
7 |
7 |
Beam current / pA |
22 |
22 |
Input count rate / kcps |
~97 |
~97 |
Map resolution / pixels2 |
640 x 360 |
1024 x 768 |
Pixel resolution / nm |
45 |
50 |
Acquisition time / min |
20 |
187 |
.jpg)
.jpg)
.jpg)
.jpg)
.jpg)
.jpg)
Figure 1. Analysis of a mineral sample from the Worthington dike
.jpg)
.jpg)
.jpg)
.jpg)
.jpg)
Figure 2. Analysis of a mineral sample from the Parkin dike
Chemical phase mapping (Autophase) identifies equally composed spectra with the aid of mathematical techniques or user defined areas.
In order to divide overlapping peaks, line deconvolution algorithms are a very important feature in the analysis software. Element identification can be enhanced greatly by applying a comprehensive atomic database with more than 300 additional L, M and N lines in the low energy range of 0–6keV (Aßmann and Wendt, 2003). The element distribution with strong overlapping peaks can be shown using net intensity maps during or after map acquisition.
Results
Cobalt nickel arsenic sulfide grain in Figure 1a reveals the element distribution of low energy X-ray lines (Figure 1b) and mineral phases (Figure 1c). Some elements like palladium, bismuth, and tellurium were not identified by the sum spectrum of the tested area, but in the maximum pixel spectrum function (Figure 1d).
Figure 1e shows the strong overlapping peaks of element lines at the lowermost energy area (iron L, nickel L, cobalt L and copper L) and Figure 1f shows the intermediate energy area (sulfur K, bismuth M, palladium L, and silver L,) can be divided by peak deconvolution.
The second example (Figure 2) shows a partial modification of pentlandite (iron nickel sulfide). It is possible to recognize the compositional variation of a cobaltite-gersdorffite solid solution with cobalt being substituted with nickel at the rim.
Conclusion
The above element study at high spatial resolution reveals that it is possible to use low energy X-ray lines for the purpose of qualitative composition establishment. The various technological advancements in research concerning atomic data, silicon drift detectors, and modern data processing have enabled elemental study at high spatial resolution.
The feature study at sub-micron scale via SEM-EDS helps to provide new understanding of telluride, sulfide, and arsenide deposit models, as plenty of detailed data can be gathered over a short span of time.
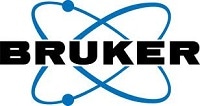
This information has been sourced, reviewed and adapted from materials provided by Bruker Nano Analytics.
For more information on this source, please visit Bruker Nano Analytics.