Manufacturing has gone through a series of major evolutions in the past few years. Subtractive processes (drilling, cutting, CNC milling and turning) and standard formative techniques (injecting, foraging, stamping, casting, molding) have been challenged by new, rapidly growing disruptive technologies, including 3D printing, laser surface texturing and additive manufacturing, among others.
This article discusses some of these new technologies versus legacy approaches for understanding the underlying parameters and challenges. How Bruker 3D optical profiling technologies fit into new and evolving manufacturing approaches are also discussed. Lastly, several case studies illustrate how optical profiling can help to optimize these new manufacturing processes.
New Manufacturing Processes
Although subtractive and formative techniques have existed for a long time, the need for flexibility in design, conception and customization has meant that manufacturing is always looking for new, improved solutions. For example, formative manufacturing requires the conception and manufacture of stamps or molds that do not require further change or customization.
This typically does reduce the cost for high-volume production. Similarly, in subtractive processes, ultra-high speed machining and advances in computing and 5-axis freedom have boosted both the usage and capability of complex part production. However, sometimes it has actually led to higher manufacturing costs due to the loss of material through subtractive techniques, that is, starting from a large block and then removing matter to design a part. Moreover, some designs are still unachievable with these methods, for example re-entrant or crossed features. This can be due, for example, to physical access limitations for a drilling bit.
.jpg)
Figure 1. Illustrations of manufacturing principles.
Certain markets, including aerospace and automotive, have triggered new manufacturing techniques that require lighter and more mechanically resistant parts with inherent flexibility of design or customization. Therefore, techniques like 3D printing, laser texturing and additive manufacturing have become populare new avenues of industrial production. 3D printing and additive manufacturing come from the same idea of creating an object one layer at a time from a CAD file (usually in STL format).
More specifically, additive manufacturing encompasses a process where metallic powder is set into a solid by sintering or melting. The standard powders are made through the atomization process of pure metals (e.g., nickel, copper, aluminium, titanium) or alloys (e.g., metallic ceramic, Inconel and steel). Most common melting techniques involve an electron beam (EBM) or a scanning laser (direct metal laser sintering) on powder beds, which can be refreshed after each pass. This technique is also known as LPBF or powder bed fusion.
3D printing however, arises from a slightly different principle. In this case, material is deposited directly one layer at a time though a nozzle via binder (binder jetting) or solid wire extrusion (fused deposition modelling). A broad variety of polymers is applicable in 3D printing manufacturing, ranging from commodity polymers (ABS, PE, PP, PVC) to high-performance ones (PEI, PEEK).
Although both 3D printing and additive manufacturing allow for flexibility and rapid customization, they share the same technical challenges. Due to columnar gain growth, anisotropic parts resistance is inherent to the layer-to-layer process. This is advantageous for specific applications where strain is exerted in a specific direction. To improve isotropy, strategies based on a crosshatch pattern for the laser scanning path or for proper overlapping between two laser paths have been proposed.
In addition, successive heating/cooling cycles and the subsequent thermal expansion/contraction for metals or recrystallization for polymers induces layer-to-layer stress. The main consequences of this include part deformation, which causes deviation from desired dimensional tolerances, as well as crack propagation or limitation to sustain fatigue. Solutions that are currently used are based on post-process annealing phase or proper stimulation of deformations to include them during the manufacturing process.
Ultimately, an optimum balance needs to be found between laser power (extrusion speed and temperature for wire), bed thickness and scanning speed for any additive manufacturing processes based on powder bed fusion and also, to a lesser extent, for material extrusion techniques. Figure 2 illustrates effects of laser power and scanning speed. To ensure high mechanical performance, these parameters are crucial. Porosity or voids formation, as well as non-cohesive materials are a direct result of poor process settings.
.jpg)
Figure 2. Illustration of the impact of laser power and scanning speed in laser selective melting on powder beds.
Characterization Techniques
As previously mentioned, 3D printing and additive technologies rely on proper manufacturing settings to be successful. As a result, characterization techniques play a role in driving process optimization by providing information on how the process went and by identifying the root causes of any failures. Bruker has a unique range of solutions with a broad variety of applicable techniques:
- For grain texture and residual stress, X-ray diffraction provides global information while an EDX sensor on an electron microscope acquires local information.
- Surface and volume can be accessed via micro-computed tomography (µCT) and optical profilometry. Critical dimensions (CD), void percentage, deviation from CAD models, waviness, and roughness are all important outputs.
- For particle chemistry, X-ray fluorescence provides elemental composition while combustion gas analysis and inert gas fusion mass spectrometry allows trace quantification of argon, carbon, oxygen, sulfur, hydrogen and nitrogen impacting mechanical properties.
- Mechanical properties can be characterized through nano (single particle/high-resolution mapping) or micro indentation (Vickers hardness). Tribology properties can also be assessed through wear (pin on desk, linear reciprocating) and fatigue testing.
.jpg)
Figure 3. Summary of Bruker characterization techniques for additive manufacturing.
This article concentrates on how 3D optical profilers turn topography measurements into quantified roughness parameters that directly link to the manufacturing process. The three case studies below cover bed fusion additive manufacturing through selective laser melting (SLM) and polymer 3D printing.
Case Study: Process Influence for Stainless Steel Parts
Compared to standard subtractive techniques, the additive manufacturing process results in different surface roughness. This case study discusses how 3D optical profiling can assess the roughness differences between processes and allows the quantification of post-process efficiency. Multiple stainless steel bars were manufactured for mechanical testing using different processes, from regular turning machine up to additive manufacturing. Additive manufacturing was performed via an EOSIn M270 machine in conjunction with EOS Stainless Steel GP1 powder along two different directions (transversal or longitudinal from bar direction). To emphasise differences, topography was captured by a white light interferometry (WLI) 3D optical profiler with a 20x objective along a 1mm2 area. Figure 4 summarizes the different processes.
.jpg)
Figure 4. Summary tab for processes and visual aspects for metallic bars. Samples courtesy University of Sydney, Australia.
Figure 5 shows the 3D topographies and confirms the differences with respect to process type. The lowest roughness is a result of a turning machined surface whilst raw additive manufactured surfaces show a large vertical range. Post-process is situated in between and shows clearly that overall roughness has been successfully reduced.
![Topography results from the four different processes. Area and vertical scales are identical for comparison (1 mm² and [-60 µm, +80 µm] respectively). Turning machined is represented in A while post-processed AM bar is shown in B. C and D show vertical and horizontal grown bars, respectively.](https://d12oja0ew7x0i8.cloudfront.net/images/Article_Images/ImageForArticle_17901(5).jpg)
Figure 5. Topography results from the four different processes. Area and vertical scales are identical for comparison (1 mm² and [-60 μm, +80 μm] respectively). Turning machined is represented in A while post-processed AM bar is shown in B. C and D show vertical and horizontal grown bars, respectively.
The mean roughness parameter Sa can be used to easily gauge the efficiency and effectiveness of roughness reduction (see Figure 6). It is a well-accepted and convenient parameter and can rank different post-processes to find the one that is most cost effective.
.jpg)
Figure 6. Graphic summary of mean roughness versus processes (left) and appearance versus shininess (right).
Furthermore, each bar has very different aspects that vary from shiny to matte surfaces. Aesthetics may play a significant role in consumer perception, depending on the market served by manufacturing, and this can make it a critical concern in final quality checks. Often this control is manual and based solely on the visual perception of the operator. The mean slope parameter Sdq can be one possible selection to reduce operator time and achieve higher consistency for the production line. There is a strong dependence and nice correlation between the mean slope and shininess.
Lastly, additive manufactured parts commonly require a second step process to optimize surface functionality performance, for example wear and mechanical resistance or the ability to strongly anchor paint/coating. Therefore, additive manufacturing needs dedicated parameters that can rank how good the initial manufactured surface is and how much it is improved by post-processing. Functional areal roughness parameters can easily work out the initial volume of matter in contact (Sm), which can inversely link with porosity volume (Sv) and abrasion resistance to impact lubricant/paint retention or mechanical strength with a high probability of cracks.
.jpg)
Figure 7. Summary of peak material and void densities for various processes.
In this study, the subtractive process leaves a smooth surface with a low surface porosity and a high load capability. This is shown by both low peak and void volume parameters. At the same time, these parameters differentiate additive manufacturing paths nicely: vertical manufacturing gets less peak volume but a higher porosity volume than horizontal manufacturing. There is a preference for horizontal layer growth due to the fact that mechanical strength and wear resistance are highly impacted in the vertical. This value, and other such parameters, can be used to gauge the effectiveness of different building strategies because it is user-independent and quantified. Finally, functional parameters emphasize how post-process operates by reducing peak volumes and porosity for improved bearing and higher mechanical strength. The effectiveness of different post-processing methods can therefore be ranked and background on inherent reasons for failures and/or underperforming products can be elicited.
Non-contact 3D profiling has clearly differentiated each process in this study and is in the position to deliver quantified normative values that can be linked to functionalities. These parameters can be used both in the development phase and in production-line quality control.
Case Study: Powder Influence for Aluminum Alloy Parts
All bed fusion-based additive manufacturing processes are so sensitive to powder selection that changing powder requires the fine tuning of production parameters. This case study illustrates the impact of changing to pure aluminum powder from Inconel 718 to build exactly the same part. Recording topography with a focus variation-based 3D optical profiler was used to quantify effects.
To achieve the best balance between vertical/lateral resolutions and field of view to capture important characteristics of both parts, a 20x objective was utilized. In addition to being able to operate on very rough surfaces, the focus variation technique gives a color image at the focus point for every pixel, on top of topography. The color gives additional information about possible alloy formation or oxidation. An example for both Inconel- and aluminum- based surfaces is shown in Figure 8.
.jpg)
Figure 8. True color image of a manufactured metal part (left), courtesy of Volum-E, France; and 3D rendering of topographies (0.7x0.7 mm²) with color map overlays of an aluminum powder (top right) and an Inconel powder (bottom right).
Figure 9 shows systematic measurements performed at identical locations. Upon initial inspection, parts manufactured with aluminum clearly have higher corrugation as well as the presence of round particles or aggregates. Conversely, a smoother surface that reveals the typical ridges left by the movement of the melting front is seen with the Inconel-based process. On occasion, round particles that were smaller and well spread apart compared to the aluminum powder were observed. This study suggests that production parameters are more suited to Inconel powder, as this produces better fusion completeness and less spatter. However at certain locations, porosity can be observed because the laser is too fast (see Figure 9, bottom row).
![Summary table showing topographies for both powders and different locations on sample. All topographies are displayed with same area and same vertical scale (0.7 by 0.7 mm², Z scale [-140 µm; +140 µm] respectively).](https://d12oja0ew7x0i8.cloudfront.net/images/Article_Images/ImageForArticle_17901(9).jpg)
Figure 9. Summary table showing topographies for both powders and different locations on sample. All topographies are displayed with same area and same vertical scale (0.7 by 0.7 mm², Z scale [-140 μm; +140 μm] respectively).
Although 3D topography provides qualitative data on how successful the process was, areal roughness can add the necessary quantitative data for correct feedback to production and subsequent fine tuning of manufacturing parameters. In this case study, invert of mean summit radius and appropriate special filtering to reveal the particles can emphazise the presence of remaining un-sintered particles and/or spatter. This is shown in Figure 10. Under these conditions, there appears to be a significant difference between aluminum- and Inconel-based parts, and this can be used to check parameters and fine tune the process or for quality control.
.jpg)
Figure 10. Differentiation between aluminum-based and Inconel-based parts through roughness parameters. Graph on left shows average value (solid bars) with 1σ dispersion (vertical lines). Topographies (aluminum-middle; Inconel-right) are shown after high-pass robust Gaussian, 2nd order and 0.25 mm cut-off. Vertical scales are optimized for particle rendering.
Through segmentation, further quantification of the number and size of the particles can be calculated. The initial analysis is strengthened by giving a clear indication of the average diameter of the particles, and this suggests possible root causes of failure by comparison to native particle diameters. An example where particles are ranked from largest to smallest average diameter is shown in Figure 11.
.jpg)
Figure 11. Left, automatic segmentation of filtered topography image from Inconel part. Right, summary of all detected particle with average diameter, total height (Rp%), and volume.
It is clearly illustrated in this study that a 3D optical profiler using focus variation can reliably characterize the impact of powder type on the additive manufacturing process. Key information, such as presence of spatter and/or remaining particle, is also captured by this technique and can be used to further track and improve manufacturing processes.
Case Study: Temperature Influence on 3D Printing of PEEK Material
PEEK is an important material and has notable inert behavior across a wide variety of environments. The pharmaceutical industry uses it to deliver aerosol drugs with adequate mixing of drug components and without anchorage of foreign particles (cleanness). This case study focused on designing a mixer using hot extrusion 3D printing. A PEEK filament was melted and then pushed out by a scanning nozzle to create the mixer one layer at a time. The primary aim was to check the influence that extrusion temperatures had on the inner channel topology. Therefore, two samples were prepared: one under too high a temperature and another under the correct temperature. After they were built, the parts were cleaved to access the inner channel for 3D optical measurement. Another non-destructive solution would have been micro-computed tomography.
.jpg)
Figure 12. Channel topography measured with a 10x objective and stitching: channel with proper temperature (6.9x3.5x0.7 mm3) on left; channel with inappropriate temperature (9.0x2.4x0.6 mm3) on right. The mean roughness (Sa) was 7.8 μm and 7.5 μm, respectively, after a high-pass Gaussian regression filter, 1st order, 0.25 mm cut-off. Samples courtesy of I-Form, Dublin, Ireland.
The 3D topography measurement clearly emphasizes the impact of temperature. Reservoirs of fluid along the channel were created by depressions/pores that were triggered by the too high temperature. This suggests a possible mixing issue and/or contamination that excludes such structures for demanding pharmaceutical applications. However, the mean roughness parameter (Sa) cannot be used as a pass/fail criteria. Surprisingly, aside from the pores, the defective channel design has a smoother appearance (see Figure 12). Furthermore, mean roughness does not provide any indication of how to drive process improvement, nor does it indicate the reason for failure.
An areal roughness parameter from the spatial section is a better indicator because 3D printing leaves a well-defined layer-to-layer pattern. For example, Figure 13 shows that the auto-correlation length (Sal) differentiates processes nicely. As well as differentiation, Sal also provides information directly on layer-to-layer periodicity and this relates directly to the manufacturing process. This is also massively advantageous because it checks the agreement between expected layer spacing and actual results simultaneously.
Sal reaches 89 mm in a proper process, which corresponds to the nozzle spacing between layers. However, for defective parts, Sal is smaller, and this indicates a mixing/merging between the layers. Therefore, the Sal roughness parameter becomes an imperative feedback parameter while changing the extrusion temperature. A defective process is implied by a higher or lower value compared to the expected layer spacing. A user can reliably and quickly converge toward the best set of parameters for optimum production using this process.
.jpg)
Figure 13. Graph showing auto-correlation length differences between parts that will work and ones that will not (left). The topography after shape removal and masking on smooth areas of the properly processed part (top right) is shown versus the topography of the defective parts (bottom right).
Conclusions
This article has discussed how important 3D optical profiling can be for process optimization, process optimization and quality control in additive manufacturing . The vertical and lateral accuracy of optical profiler metrology, combined with non-contact and areal measurements, leads to reliable diagnostics and actionable assessments on how good a manufacturing process is across different powders, different temperatures or different processes. Therefore, 3D optical profiling has become a key characterization technique for supporting the development and fine tuning of additive manufacturing techniques and 3D printing.
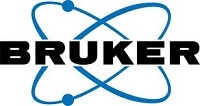
This information has been sourced, reviewed and adapted from materials provided by Bruker Nano Surfaces.
For more information on this source, please visit Bruker Nano Surfaces.