When we were at school we probably believed that science and mathematics were primarily concerned with offering definitive answers. But grown-up scientists and engineers know that this is rarely the case.
Uncertainty is inherent in almost every system studied and every experiment conducted. It might come from natural scatter in the experimental results, limitations in the precision of measurement methods, errors made when performing measurements, or the need to extrapolate from incomplete data.
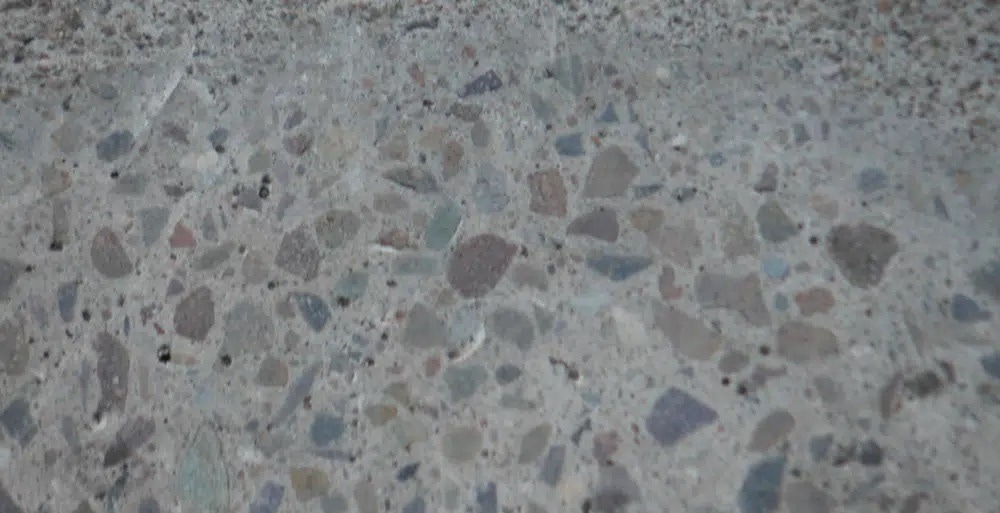
Image Credit: Intellegens Limited
We also need to deal with uncertainty in machine learning (ML). Predictions from ML models will be uncertain partly due to inherent scatter in the training data, and partly because models are built from limited data.
It is important to embrace this uncertainty, rather than expecting to eliminate it entirely. It needs to be understood, quantified, and used to make better decisions.
In fact, uncertainty is a positive. Just as a straight line that passes through all points on a graph reveals how straightforward a system is to characterize, a model containing negligible uncertainty is not likely to be very useful or insightful. It is the difficult and complex problems that come with uncertainty, and these are the issues that are valuable to resolve.
ML specialist Intellegens has taken every care to ensure that uncertainty is faithfully quantified within the predictions of its Alchemite™ algorithm. The algorithm expands on conventional methods that, at worst, do not really estimate uncertainty at all but assume a normal distribution, or only capture one of many contributions to the overall uncertainty.
Accurate quantification makes it possible, for example, to identify the likelihood of an experiment succeeding so that the user can target experimental work accordingly.
Intellegens CSO, Dr. Gareth Conduit, introduced some interesting new research work from his group at the University of Cambridge in a recent webinar. The work performed by Dr. Conduit and his team goes a little further in embracing uncertainty.
It focused on the design of concrete, for which measurements of many parameters associated with the material appear ‘noisy.’
Rather than simply quantifying the uncertainty, this work allowed the machine learning method to learn from the noise in the data, discovering that the uncertainty in one particular physical parameter could be used to help predict the concrete strength.
Two new concrete mixes were proposed as a result of this work, subsequently created, and then found to behave as predicted, which expanded the properties of mixes that are commercially available.
If we’re looking for some certainty in all of this it is that machine learning can do interesting things in the world of chemicals and materials. But only if you take the right approach to uncertainty!

This information has been sourced, reviewed and adapted from materials provided by Intellegens Limited.
For more information on this source, please visit Intellegens Limited.