‘The Age of Plastic’ was an album by the 1980s British new wave group, The Buggles – a point to which we shall return!
More pertinently, it is a term often used to describe the remarkable rise of this class of material since the development of the first synthetic plastic, Bakelite, in 1907. From a materials science perspective, the proliferation and reach of new plastics-related technologies in a comparatively short time has been a spectacular achievement. Over just a few generations, the production, development, and distribution of tens of thousands of grades of lightweight, strong, flexible new materials have made plastics intrinsic to modern life.
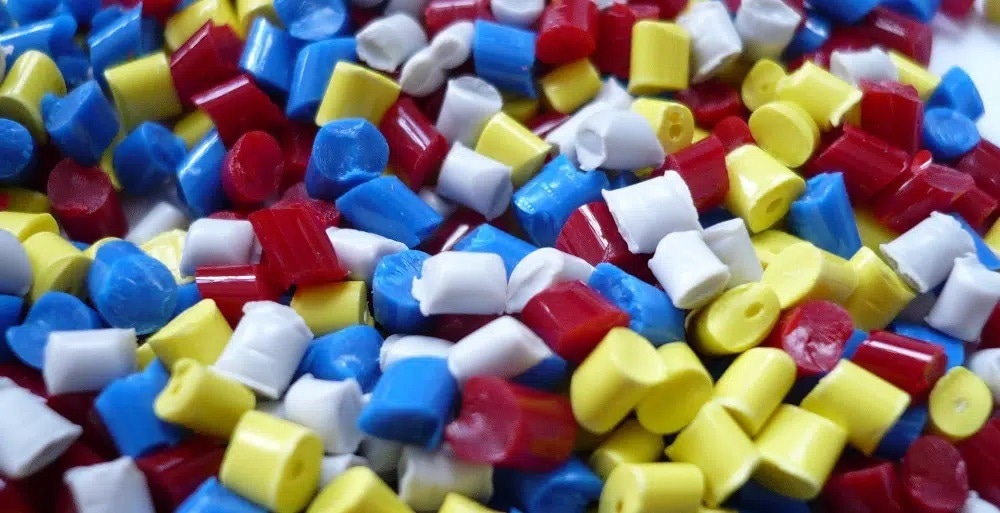
Image Credit: Intellegens Limited
But we should also acknowledge the scale and severity of plastic’s environmental consequences. Plastic production is tied to oil-centric economic models, the main driver of climate change. Plastic pollution is blighting the seas, with catastrophic consequences already being felt by marine life and vital ecosystems.
It is no longer possible to simply innovate a way out of these problems. Fundamental political decisions must be made concerning where society invests, along with significant upgrades to recycling infrastructures and a serious societal commitment to reducing single-use plastic items and unnecessary packaging.
But, while innovation is certainly not a ‘magic bullet’ that can be deployed to solve the plastics challenge, research and development in the world of plastic must be part of the solution.
For example, the percentage of biopolymers derived from non-petrochemical sources can be increased, and better use can be made of recycled feedstock in manufacturing.
Harmful additives can be reduced, and plastics can be made more biodegradable for applications where disposal cannot be avoided. Care can also be taken to make plastics less likely to degrade in applications, where such an effort will significantly extend their usable life.
So polymer science is important. This is a science that retains an element of ‘art.’ This is partly because, at the molecular level, polymers consist of long chain molecules, which are often tangled in arrangements more complex and organic than, for instance, metals or ceramics.
It can be difficult to understand and gain precise control of properties, which means that big differences can result from subtle changes in how polymers are blended, processed, and which additives are used. Cookery terms, such as ‘recipe,’ are often used in relation to polymer formulation.
This is where machine learning can come in. Costly investments in both experiment and simulation are required for the strong element of empirical research in polymer R&D in order to identify beneficial tweaks in formulation, chemistry, or process parameters.
Machine learning specialist Intellegens is increasingly discovering polymer R&D organizations seeking to use its tools to more effectively mine the data built up through this work.
Such organizations use machine learning to identify relationships within the data that provide clues on how to change their processes or materials. Time and costs are saved by identifying areas in which it would be most beneficial to the focus of an experiment or simulation.
The relationships found by machine learning are captured in models that can be used to predict how changing system inputs affects the outputs. These models might, in the future, be used to manage polymer production – for instance, to change process parameters in response to the inevitable variability in incoming recycled feedstock composition. The models also embody vital knowledge about the system (some of that ‘art’), an important added benefit in an industry where so much expertise is locked-up in the minds of experts who may leave or retire.
The hit track from that The Buggles album was ‘Video Killed the Radio Star’. Take a look at its lyrics: “Rewritten by machine on new technology, and now I understand the problems you can see.” Maybe The Buggles foresaw something more positive for the real Age of Plastic.

This information has been sourced, reviewed and adapted from materials provided by Intellegens Limited.
For more information on this source, please visit Intellegens Limited.