Artificial Intelligence and its related tools, such as machine learning, deep learning, and neural networks, are revolutionizing every field of life. The domain of materials science and engineering is constantly evolving by employing these effective tools for the discovery and development of novel materials. The use of machine learning has provided a significant boost to the field of materials discovery, and experts are using this advanced technique to discover various types of materials.
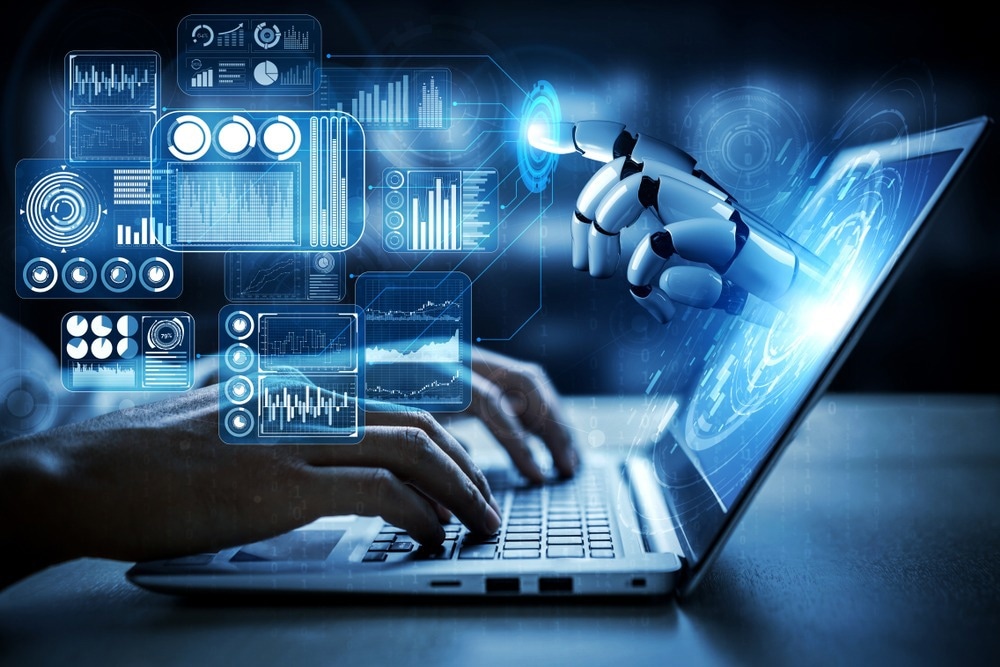
Image Credit: Summit Art Creations/Shutterstock.com
An Overview of Machine Learning for Materials Sciences
In recent times, machine learning (ML) has become the center of attention, especially for research studies. ML models are much more efficient, and their operation is based on a data-driven approach. These models can analyze massive amounts of data without the need for extensive domain knowledge or profound insights into a specific field.
An article published in the Annual Review of Materials Research states that ML is being extensively utilized for predicting the properties of new materials, such as the bulk stability of perovskite oxides, dielectric properties of polymers, and electronic bandgap of different classes of inorganic materials.
Machine Learning (ML) has expanded its capabilities beyond property prediction to facilitate the exploration, design, and creation of innovative materials across diverse applications and material categories. This is achieved by uncovering novel insights into the fundamental chemical or physical relationships that influence properties of interest.
ML, in addition to property prediction and design of new materials, has significantly influenced Molecular Science and Engineering (MS&E), particularly in the realm of molecular simulation. ML is extensively utilized to create interatomic potentials and enhance the efficiency and accuracy of ab initio simulations. Ab initio simulations involve working with simplified and schematic representations of intricate physical systems.
ML as a tool for Accelerated Materials Discovery: Prediction of Crystallographic Symmetry Groups
Physics-guided machine learning can accurately model and calculate the electronic structure and other properties of solid materials based on known crystal geometry and electronic density. The crystal structure is defined by symmetry properties, lattice parameters, and centering.
The conventional method for predicting the crystal structure of a solid material involves calculations using atomic-scale computational methods, particularly density functional theory (DFT). However, these calculations can be computationally expensive. The recently available efficient ML models, along with the extensive materials databases, offer an alternative and convenient approach to estimating crystal structures and other material properties through surrogate models.
In the latest article published in the Journal of Physical Chemistry, the researchers introduced a robust ML model achieving a weighted balanced accuracy (BA) of over 95% to forecast crystal space groups and other symmetry groups for binary and ternary crystals based solely on their chemical composition with a minimal set of features.
This algorithm is a big step towards the complete prediction of crystal structure from chemical composition, providing a platform to develop a model for a long-standing challenge in condensed matter physics.
The achieved weighted balanced accuracies range between 95-98% for ternary materials and 80-83% for binary materials. This is accomplished using a small set of features, including stoichiometry, ionic radii, ionization energies, and oxidation states for each element in the compound. The effectiveness of this small feature set in achieving high accuracies suggests a robust capture of the main physical aspects. The developed classifier is an extended random forest classifier capable of handling multi-label multi-class problems.
Deep Learning for Materials Discovery
The exploration of novel functional materials is crucial for technological advancements in various applications, including clean energy and information processing. Identifying energetically favorable inorganic crystals is a key focus in solid-state chemistry. While experimental approaches have cataloged around 20,000 computationally stable structures in the Inorganic Crystal Structure Database (ICSD), the impracticality of scaling this strategy is evident due to associated costs, processing limitations, and synthesis complexities.
In the latest article published in Nature, the researchers devised a strategy to overcome the challenges of scaling up materials exploration by employing large-scale active learning, enabling the creation of accurate predictive ML models for stability that can guide materials discovery.
The new approach involves two key steps: first, the development of methods for generating diverse candidate structures, incorporating new symmetry-aware partial substitutions (SAPS) and random structure search. Second, the researchers leveraged state-of-the-art graph neural networks (GNNs) to enhance the modeling of material properties based on structure or composition.
In the evaluation process, candidate structures, filtered using the GNoME framework in both structural and compositional aspects, were validated through Density Functional Theory (DFT) calculations. The energies of the relaxed structures not only confirmed the stability of crystal structures but were also fed back into the iterative active-learning workflow as additional training data and structures for candidate generation.
The performance of GNoME models, as measured by test loss, demonstrated improvement following a power law with increased data. This aligns with neural scaling laws in deep learning, indicating that ongoing discovery efforts could lead to continued enhancements in generalization.
Graph neural networks (GNNs) trained on an extensive and diverse set of first-principles calculations proved effective in efficiently discovering inorganic materials. This approach substantially increased the number of stable crystals, exceeding the capabilities of traditional methods by more than an order of magnitude. The associated datasets also empowered machine-learned interatomic potentials, enabling accurate and robust molecular dynamics simulations for previously unseen bulk materials.
New ML Model for Discovery of Materials
Scientists at the U.S. Department of Energy’s (DOE) Argonne National Laboratory have successfully demonstrated an automated process for identifying and exploring promising new materials through the integration of machine learning (ML).
The researchers employed the element carbon as a prototype, utilizing the ML algorithm to predict the atomic arrangements under various temperatures and pressures, resulting in different substances. The algorithm constructed phase diagrams mapping hundreds of metastable states of carbon.
To train the ML algorithm, the scientists utilized synthetic data generated through molecular dynamics and density functional theory, common tools in computational chemistry. The synthetic data approximated experimental results that would be obtained through physical experiments.
The ML algorithm demonstrated success in predicting familiar phase diagrams for carbon. Additionally, the computer-generated phase diagrams not only confirmed existing knowledge but also provided insights into previously unexplained experimental observations.
Material innovation is crucial for technological advancement and industrial growth. Machine learning plays a significant role by reducing computational costs, shortening development cycles, and enhancing accuracy in the screening of novel materials and predicting material properties. It has emerged as a promising research approach in this field. With the development of efficient and fast ML algorithms with each passing day, it is expected to play an essential role in the future of materials science.
More from AZoM: New Advancements in Battery Life Extension
References and Further Reading
Merchant, A. et al. (2023). Scaling deep learning for materials discovery. Nature 624, 80–85. Available at: https://doi.org/10.1038/s41586-023-06735-9
Mitchem, S., (2022). Scientists Use Machine Learning to Accelerate Materials Discovery. [Online]
Available at: https://www.anl.gov/article/scientists-use-machine-learning-to-accelerate-materials-discovery
Srinivasan, S. et al. (2022). Machine learning the metastable phase diagram of covalently bonded carbon. Nat Commun 13, 3251. Available at: https://doi.org/10.1038/s41467-022-30820-8
Morgan, D., & Jacobs, R. (2020). Opportunities and challenges for machine learning in materials science. Annual Review of Materials Research, 50, 71-103. Available at: https://doi.org/10.1146/annurev-matsci-070218-010015
Alghofaili, A. et. al. (2023). Accelerating Materials Discovery through Machine Learning: Predicting Crystallographic Symmetry Groups. The Journal of Physical Chemistry C, 127(33), 16645-16653. Available at: https://doi.org/10.1021/acs.jpcc.3c0327
Disclaimer: The views expressed here are those of the author expressed in their private capacity and do not necessarily represent the views of AZoM.com Limited T/A AZoNetwork the owner and operator of this website. This disclaimer forms part of the Terms and conditions of use of this website.