By Surbhi JainReviewed by Susha Cheriyedath, M.Sc.Mar 25 2022
In an article recently published in the journal Energies, researchers presented a comparative study of efficient wind power prediction using machine learning methods.
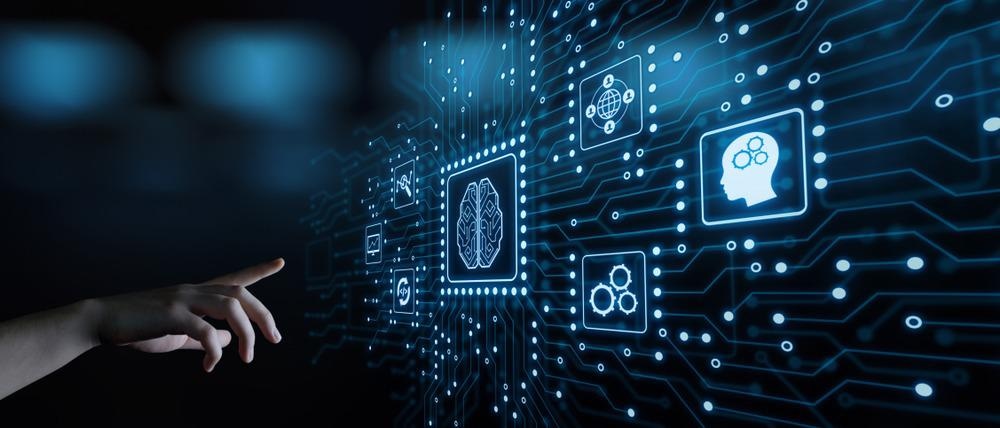
Study: Efficient Wind Power Prediction Using Machine Learning Methods: A Comparative Study. Image Credit: Alexander Supertramp/Shutterstock.com
Background
Wind power capacity has recently increased dramatically, making it a promising source of renewable energy. The biggest obstacles in wind power management are its intermittent swings, which are mostly caused by weather conditions, making its integration into a power grid a difficult operation. As a result, wind power forecasting is very important for the efficient integration of wind turbines into the power system.
There has been a growing interest in creating accurate wind power prediction algorithms during the last two decades. To improve wind power prediction, various data-driven methodologies have been developed in the literature.
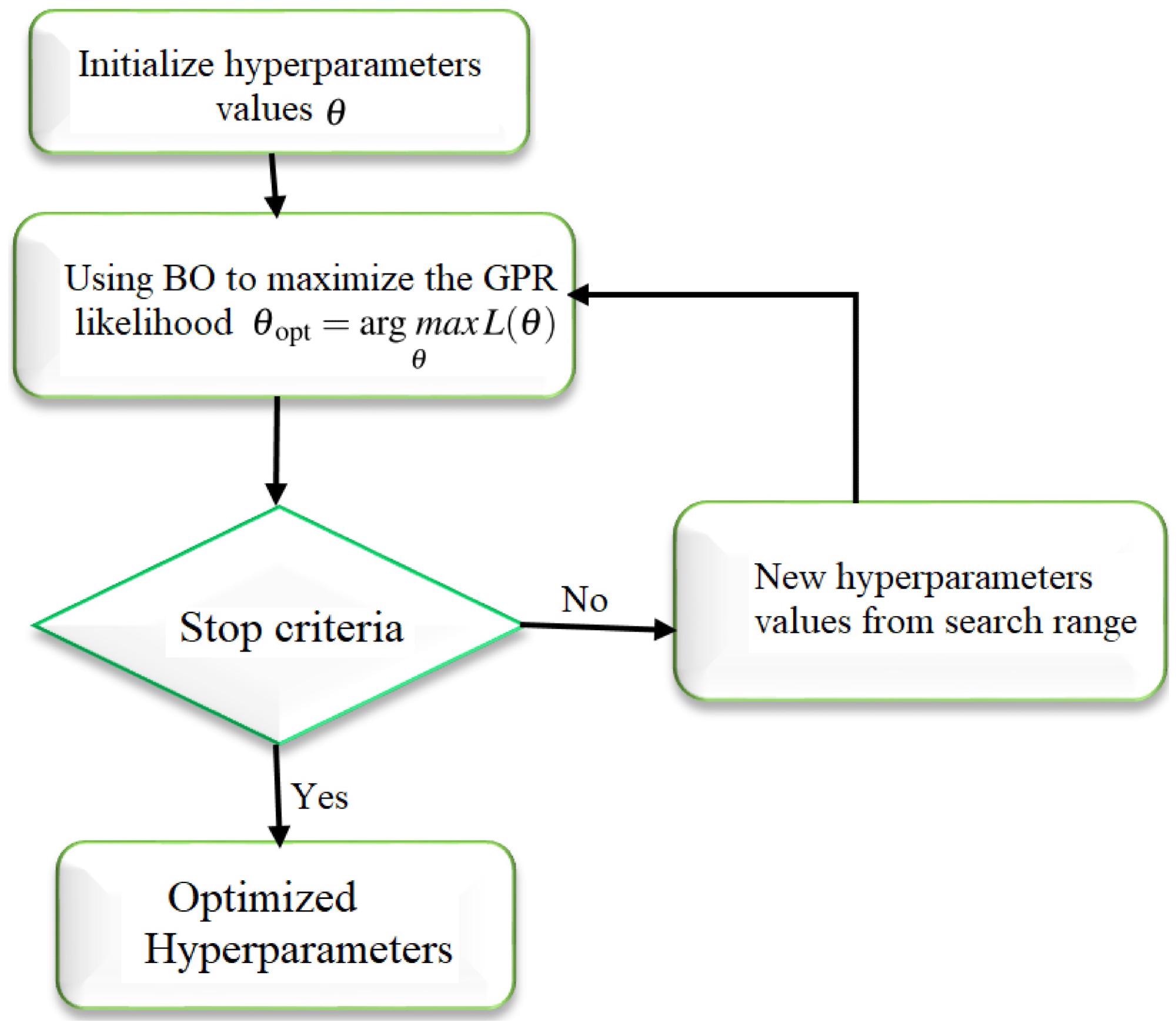
BO-based optimized GPR procedure. Image Credit: Alkesaiberi, A et al., Energies
Over the last decade, machine learning approaches have established themselves as a major topic of research within a data-driven framework by addressing a variety of tough challenges in electricity, gas, and other real-world applications. Lately, various machine learning approaches for predicting wind power have been developed in the literature by combining the advantages of diverse models.
About the Study
In this study, the authors presented the development of data-driven models that can properly estimate wind power generation. The potential of augmented machine learning models towards forecasting univariate wind power time-series data was examined. The authors used Bayesian optimization (BO) to fine-tune the Support Vector Regression (SVR) with multiple kernels, the hyperparameters of Gaussian process regression (GPR), and ensemble learning (ES) models e.g., Bagged trees and Boosted trees to improve the predicting performance.
To improve the forecasting performance of the analyzed models, dynamic information was added to their development. To simulate nonlinear wind power dynamics and estimate future wind power trends, multiple machine learning algorithms were used and compared.
The team used lagged measurements to incorporate time evolution into the design of the models under consideration. In addition, extra input factors (such as wind direction and speed) were used to increase wind prediction accuracy. The efficiency of the investigated models was verified by using actual measurements from three wind turbines in France, Kaggle, and Turkey.
The researchers provided a quick and easy machine learning method for forecasting wind power statistics. The accuracy of machine learning models for forecasting univariate wind power time-series data was verified. For the wind power forecast, seven machine learning algorithms were examined, including kernel-based methods (i.e., GPR and SVR models) and ensemble learning techniques (Bagging, Random Forest, Boosting, and eXtreme Gradient Boosting (XGBoost)). To develop the considered models, five-fold cross-validation was performed on the training set.
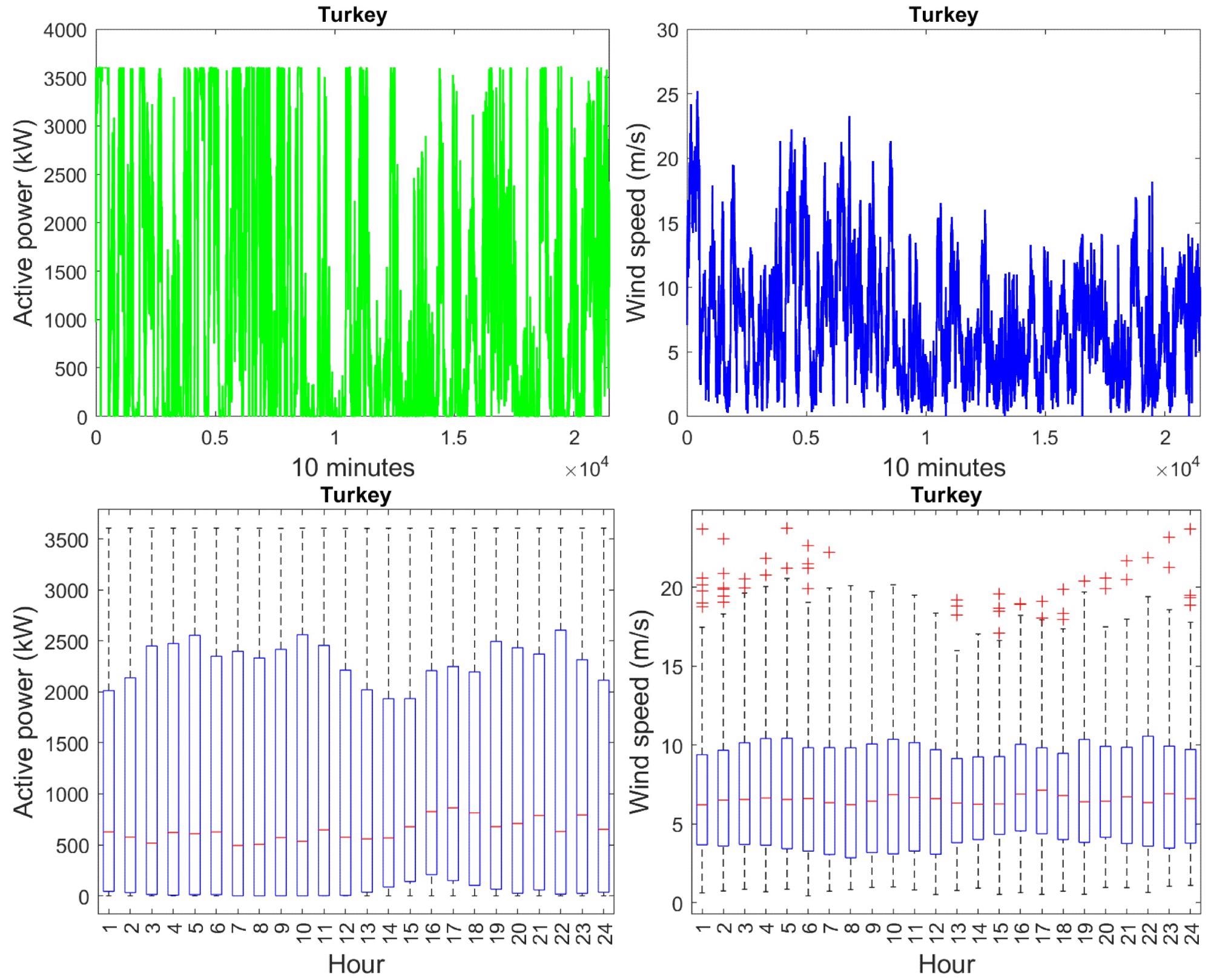
Wind power (top left) and wind speed (top right) data from Turkey wind turbine; box plot of hourly data for wind power (bottom left) and wind speed (bottom right). Image Credit: Alkesaiberi, A et al., Energies
Observations
In comparison to the Boosting tree (BT) model with input variables, the root means square error (RMSE) value of the BT model without any inputs was reduced by 157.51, and the R2 value increased by 0.18. By capturing nearly 99% of the variation in wind power data (i.e., R2 = 0.99), the Bagging trees model outperformed all other models. For the Turkey data, the GPRO had the greatest results, with an R2 of 0.9789, and for the Kaggle data, the Bagging technique had the best results, with an R2 of 0.9578.
Considering lagged data and input variables could help forecast wind power more accurately. The improved GPR and ensemble models also outperformed the other machine learning models. It was demonstrated that incorporating input variables improved the accuracy of wind power forecasts significantly. In comparison to static models, the results showed that utilizing dynamic models that consider important information from historical observations of wind power series enhanced forecast outcomes.
It was also observed that incorporating data from input factors (such as wind direction and speed) improved prediction accuracy. For all studies, no single strategy outperformed the others, although on average, the ensemble and GPR models achieved an effective prediction performance, with an R2 value of around 0.95.
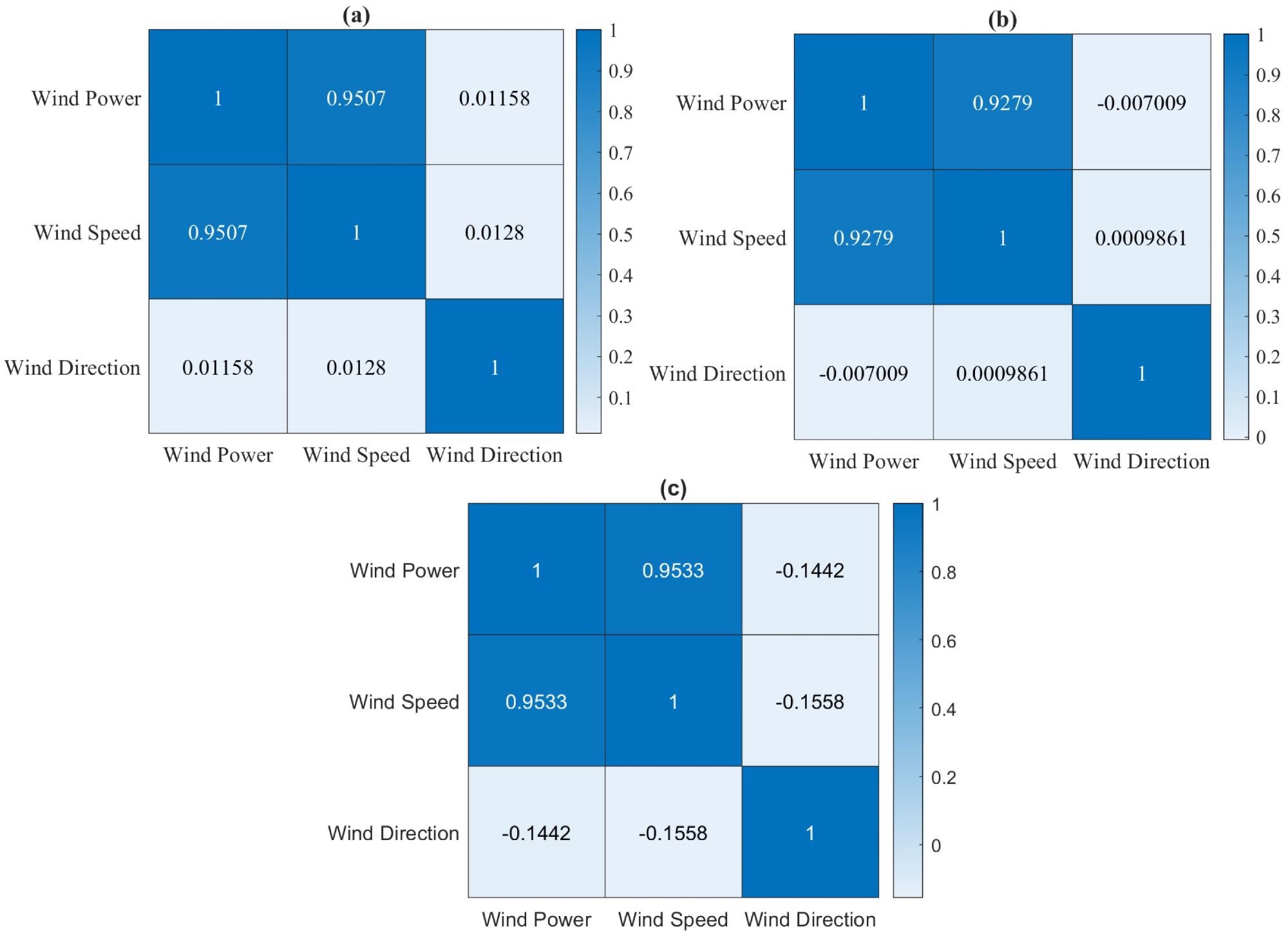
Heatmap of the correlation matrix of the wind turbine in (a) France, (b) Turkey, and (c) Kaggle. Image Credit: Alkesaiberi, A et al., Energies
Conclusions
In conclusion, this study elucidated dynamic machine learning models to predict wind energy efficiency. The authors emphasized that despite the positive results of employing dynamic machine learning approaches to predict wind power, future work should focus on creating wavelet-based dynamic machine learning methods to improve the robustness of machine learning techniques against noisy wind power measurements.
They suggested that another way to increase prediction quality is to include explanatory factors, such as meteorological measurements, in the machine learning approaches. They also believe that the dynamic machine learning models proposed in this study could be used to mimic the standard operating condition of the wind turbines, and the statistical monitoring charts like generalized likelihood tests and exponentially weighted moving average can be utilized to detect faults.
Disclaimer: The views expressed here are those of the author expressed in their private capacity and do not necessarily represent the views of AZoM.com Limited T/A AZoNetwork the owner and operator of this website. This disclaimer forms part of the Terms and conditions of use of this website.
Source:
Alkesaiberi, A., Harrou, F., Sun, Y., Efficient Wind Power Prediction Using Machine Learning Methods: A Comparative Study. Energies 15(7) 2327 (2022). https://www.mdpi.com/1996-1073/15/7/2327