Researchers from the University Rey Juan Carlos in Spain have reported the development of a novel, lightweight, and state-of-the-art sensor that possesses improved 3D human pose detection. The paper is currently in the pre-proof stage in the journal Displays.
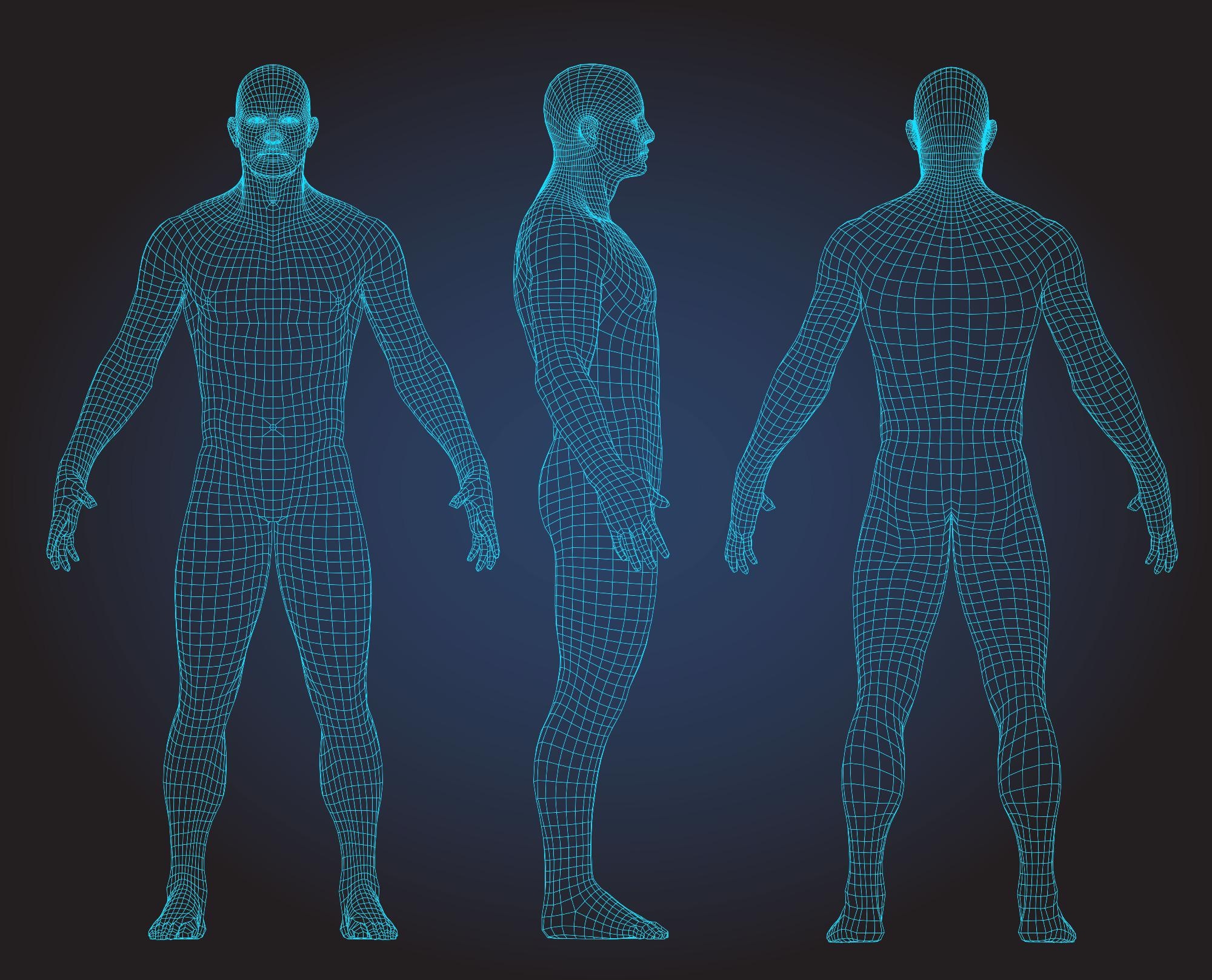
Study: Efficient 3D human pose estimation from RGBD sensors. Image Credit: Ovocim/Shutterstock.com
Human-Robot Interaction
The field of robotics is a key area of technological development in the 21st century. Robotics is a cross-sectional field of research that has been applied in several industries such as manufacturing, the military, biomedicine, mining, resource exploration, and even in space.
Optimal robotic performance relies on several factors, including the ability to manage huge data streams and react accordingly. Other applications such as domestic robots require systems to be lightweight and portable. Central to the development of cutting-edge technologies in the field of robotics is the use of agile and adaptable algorithms which can be incorporated into software systems.
A field of robotics and software design that has been gaining attention in recent years is Human-Robot Interaction. Human-Robot-Interaction is essential for the design of applications such as assistive robotics, home automation, and search and rescue robots. These types of robots must possess some level of awareness of human subjects, and streams of data from suites of sensors are employed to give devices this functionality. Audio and visual sensors enable verbal and non-verbal communication.
Computer vision gives these devices powerful Human-Robot Interaction capabilities. Human detection, gesture recognition, and human pose estimation are all used to interpret and act upon video signals from sensors attached to robots. Human pose estimation is particularly beneficial as it can help robots solve higher-level tasks. For instance, in assistive robots, it can inform the system as to whether a person has suffered a fall, allowing the robot to alert emergency services.
In essence, providing robots with superior human pose estimation capabilities helps them better understand an environment and scene, which is essential for the design of effective human-robot interfaces. However, the design of accurate, reliable, and adaptive human pose estimation systems is still a challenging area of research in robotics and computer vision.
Motion Capture Systems
One solution is to use a motion capture system. These systems are typically used in indoor environments but possess some limitations. Motion capture systems rely on markers worn by individuals and the use of multiple sensors and cameras placed around the environment. Cameras are usually placed in high vantage points, as this allows maximum coverage of a scene. The larger the area, the more cameras are needed in motion capture systems.
This makes motion capture systems cumbersome and costly, which hinders their widespread use for industrial or assistive applications. There is an urgent need for low-cost, lightweight, simple, easy to operate, and portable systems which can be widely distributed in commercial settings. Deep learning-based solutions such as convolutional neural networks provide an elegant solution to these issues, imbuing robots with enhanced human pose estimation capabilities.
The Paper
The authors have reported the development of an innovative, novel human pose estimation system that addresses the current challenges in the field. Their proposed system is lightweight and can be easily embedded in existing robotic systems that require real-time sensing capabilities. It does away with the need for cumbersome and expensive cameras and sensors that are typically required by conventional motion capture systems.
The system’s algorithm was trained with video sequences captured by commercial RGBD sensors, a common type of sensor used in conventional robots. The proposed hybrid human pose estimation system’s pipeline comprises two-dimensional estimation and three-dimensional registration stages. 2D poses are converted into 3D coordinates using an agile deep-learning algorithm to leverage depth information from sensors.
The computational burden and accuracy of several state-of-the-art deep learning processes for 2D pose estimation were evaluated by the authors. By evaluating these factors and converting 2D poses into 3D coordinates, the proposed system is an incredibly elegant and sophisticated approach to solving common issues faced by conventional pose estimation methods.
The accuracy of the proposed 3D pose estimation method was compared with other state-of-the-art algorithms. To compare the computational burden and accuracy of methods, the authors employed a publicly available international dataset. The novel system presented in the paper achieved comparable results with other algorithms with a lower computational cost.
Results of the work indicated that this proposed human pose estimation system provides a novel, low-cost, competitive, and lightweight solution that works in multiple scenes with different points of view and can be used with commercially available depth sensors.
The authors have stated that the system can be updated further to meet specific application needs with novel approaches which will improve it. Moreover, the proposed system can be adapted to other types of poses, such as animal poses, making it incredibly useful for multiple applications.
More from AZoM: Why and How Do We Dope Semiconductors?
Further Reading
Pascual-Hernández, D et al. (2022) Efficient 3D human pose estimation from RGBD sensors Displays 102225 [online, pre-proof] sciencedirect.com. Available at: www.sciencedirect.com/science/article/abs/pii/S0141938222000579
Disclaimer: The views expressed here are those of the author expressed in their private capacity and do not necessarily represent the views of AZoM.com Limited T/A AZoNetwork the owner and operator of this website. This disclaimer forms part of the Terms and conditions of use of this website.