By Surbhi JainReviewed by Susha Cheriyedath, M.Sc.Jun 29 2022
In an article recently published in the journal ACS Applied Energy Materials, researchers discussed the utility of a neural network to automatically capture key features for solid-state electrolyte superionic conductivity prediction.
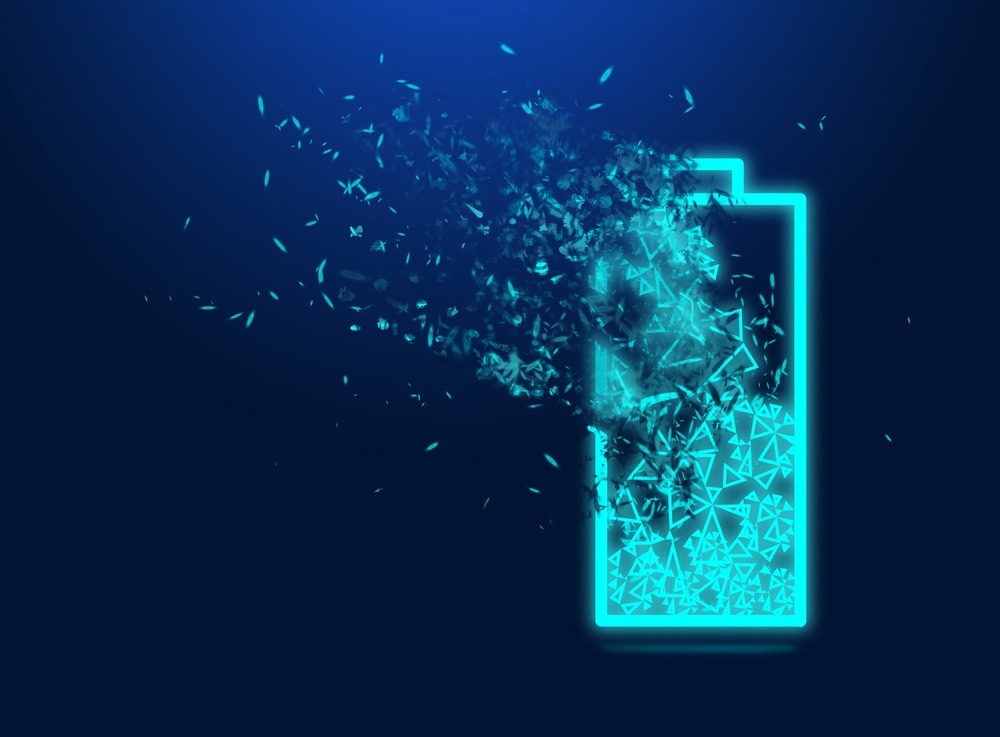
Study: Automatically Capturing Key Features for Predicting Superionic Conductivity of Solid-State Electrolytes Using a Neural Network. Image Credit: New Africa/Shutterstock.com
Background
There is an increasing need to improve the safety and performance of batteries as part of the global push to convert the transportation industry to infrastructure based on electricity. Solid-state batteries (SSBs) are an important future technology that may warrant both metrics. Although the community is aware of these benefits of solid-state design and great progress has been made in the laboratory, there are still challenges to the commercialization of SSBs in the transportation industry, such as identifying the best solid electrolyte materials. Large-scale screening techniques have been used to find solid-state electrolytes (SSEs) with high stability and ionic conductivity to solve this problem.
Machine learning (ML) has recently been used to identify robust SSE with this goal in mind. The majority of characteristics in current ML studies on SSEs that best capture the SSE structure are chosen by hand. However, manual feature selection necessitates human involvement in the ML process, which could be time-consuming and lead to bias during material selection and identification. Additionally, as manual ML feature construction relies on intuition drawn from existing knowledge, it may be challenging to explore material classes that are unrelated to one's previous experience.
About the Study
In this study, the authors demonstrated the capability of a neural network-based model to produce excellent predictive performance comparable to earlier manual feature-based studies with only two simple elemental features i.e., group and period, and one simple structural feature i.e., coordination number, while it automatically captured any potential secondary features and required less human involvement in model training.
The team combined a feed-forward neural network (NN) model with reduced primary features for elemental identification and structure. These main properties were associated with other secondary properties, such as electronegativity, from which the layers of the NN could be formed. The use of only three basic input features eliminated the need for feature selection and enabled an effective and robust model. The NN automatically learned the key features not typically defined in the data tables that best represented a candidate SSE material.
The researchers created a NN model which required only three input features for each atom to determine if a solid material exhibited superionic conductivity for SSE applications.
Observations
The findings revealed that the trained NN model successfully identified 11 out of 12 materials as superionic conducting materials. According to the sensitivity level determined using the test data, this translated to a sensitivity of 92%. The quantity of training data points needed was also decreased due to the lowered model parameters. When the training set size was reduced to the lower levels of 1200 data points and subsequently to 600 data points, the accuracy levels of the testing set remained at around 96%, which indicated that as low as around 600 data points sufficed for fully training of the NN model, according to research on the NN model's dependence on model parameters.
However, until the training set reached about 100 points, the accuracy was continuously above 80%, which suggested excellent flexibility of the constructed NN model. The testing set accuracy soon decreased when the quantity of training data points went below 500 points.
For a selection of recently experimentally examined materials, it was shown that superionicity predictions were accurate. With the use of the proposed NN model, the requirement for subjective manual selection of material descriptors and potential bias introduction was reduced. The trained model displayed a binary accuracy of 96% on the testing set and displayed no signs of overfitting, as evidenced by the accuracy level remaining constant across different training set sizes.
Conclusions
In conclusion, this study elucidated that the NN-enabled simple technique could effectively extract the majority of the data from SSE crystals without the assistance of a person. The created NN model might be a component of a bigger model that could simultaneously predict all important SSE characteristics, including ionic conductivity, stability, and electrical resistance.
The authors demonstrated that while significantly decreasing the time and effort needed to train and use such a model for SSE material screening, the reduced NN model exhibited potential for greater generalizability.
They believe that the proposed model is theoretically more generalizable than manual feature-based models and can even be used to predict other material properties.
Disclaimer: The views expressed here are those of the author expressed in their private capacity and do not necessarily represent the views of AZoM.com Limited T/A AZoNetwork the owner and operator of this website. This disclaimer forms part of the Terms and conditions of use of this website.
Sources:
Lu, Z., Adeli, P., Yim, C-H., et al. Automatically Capturing Key Features for Predicting Superionic Conductivity of Solid-State Electrolytes Using a Neural Network. ACS Applied Energy Materials (2022). https://doi.org/10.1021/acsaem.2c00493
https://pubs.acs.org/doi/10.1021/acsaem.2c00493