Scientists from Spain have collaborated on a new research paper published in the journal Sustainability, applying machine learning to archaeology for critical issues such as determining artifact provenance and sustainability.
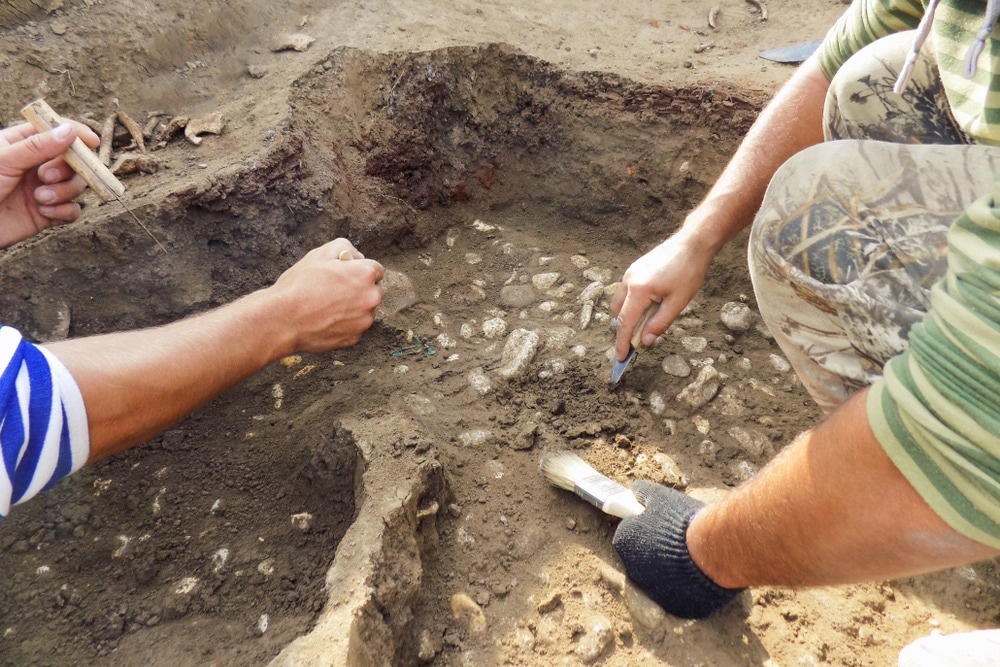
Study: Supervised Machine Learning Algorithms to Predict Provenance of Archaeological Pottery Fragments. Image Credit: Masarik/Shutterstock.com
Improving the Provenance and Sustainability of Archaeology
Archaeology is a key scientific field that reveals the secrets of the past, helping to fill in gaps in historical knowledge. The field is mature, yet there are still challenges that need to be overcome to further improve archaeological digs and provide data that enhances the context of findings in the historical record.
Key issues in the field of archaeology concern artifact provenance and sustainability. The most common artifacts found in archaeological digs are pottery shards, which can provide a wealth of information including age, evidence for cultural connections, knowledge exchange, and production technology.
There is an entire branch of archaeometry that investigates the physiological and geochemical analysis of artifacts, mainly pottery shards, to reveal their provenance. Archaeometry is the application of scientific and technological methods to the field of archaeological study.
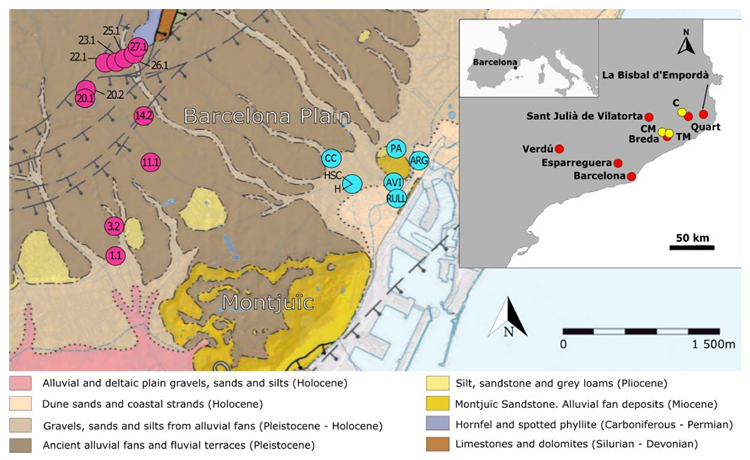
Geological map of the clay sampling sites (pink dots) and archaeological sites (blue dots) mainly around the Sarrià-Sant Gervasi and Ciutat Vella districts, respectively. The geological base map was modified from ICGC. The top right corner shows a geographical map with the location of each characterized production center (red dots) and the location of the three archaeological sites that were studied (yellow dots). Image Credit: Anglisano, A et al., Sustainability
Sustainability Issues
Archaeological findings occur not just in the context of the time period they are from but in the context of the modern world and the intervening historical period. Activities such as farming, construction, and urbanization complicate questions of provenance and the sustainability of archaeological digs and data.
Additionally, the development of novel approaches and analytical techniques and digital tools have caused an exponential growth in datasets. Whilst this would not be a problem normally in other areas of human activity, in archaeology it is complicating the economic sustainability of the field.
Routes toward improved sustainability in archaeology include the promotion of data standardization, open data, data sharing, and data recycling. Promoting these approaches minimizes the amount of analysis required during archaeological and archaeometrical analyses.
Current Approaches
Provenance studies require the definition of reference groups. However, reference samples, which are essential to these studies, are rarely used by disparate authors in the field of archaeology. Common approaches to retrieving information on artifacts include petrochemical and chemical methods or a combination of both. These are used both for isolated research and multiple investigations by research groups.
Large datasets are produced using methods such as neutron activation analysis and X-ray fluorescence. Processing these large datasets commonly requires statistical methods to be applied.
Conventional statistical analysis methods include hierarchical cluster analysis and principal component analysis or unsupervised cluster methods. Other kinds of analytical data such as shard profiles, color, and X-ray diffraction can be processed using these unsupervised methods.
However, these unsupervised methods cannot easily discriminate between classes of data corresponding to provenance sites that share similar features. A key issue is that data are not labeled before classification. In contrast, supervised methods are more powerful and suitable approaches. Key benefits of these methods are their ability to learn from training datasets and better knowledge of reference sample provenance.
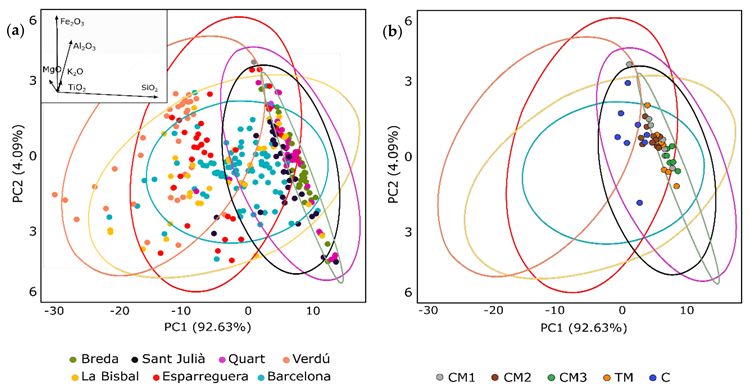
(a) PCA biplot of factor scores for the first two principal components for all the reference samples, 95% confidence ellipses were drawn for every class. Inset: PCA biplot of the most relevant variables. (b) The position of the samples of unknown provenience within the PCA biplot where the confidence ellipses were kept. Image Credit: Anglisano, A et al., Sustainability
The Study
The new paper in Sustainability has explored the use of machine learning to improve knowledge of provenance and consequent sustainability of archaeological investigations. Machine learning is a fast-growing field of scientific endeavor that is increasingly being employed in archaeology.
Deep learning approaches, especially deep convolutional networks, display growing accuracy in recognizing patterns by analyzing images. These approaches have already been successfully applied in remote sensing for prospection and artifact classification. Classification criteria in these approaches include morphology and the engravings on pottery shards.
The paper demonstrates the suitability of machine learning methods for providing key provenance information on pottery shards using chemical analyses. The research employed chemical datasets from six sites in Spain. These reference datasets were extended to a site in Barcelona that has produced pottery shards.
Discrimination models were trained and optimized to provide accurate provenance information on pottery samples from the region of Catalonia in Spain. Moreover, the trained machine learning models can be applied to other sites in the same region. The main aim of the study was to evaluate how supervised models could perform better than unsupervised approaches.
Another focus of the study was to extend the supervised clustering algorithm approach to provide enhanced provenance capabilities for the field of archaeology. This will help the archaeological community to easily implement these machine learning-based approaches and move away from conventional unsupervised methods.
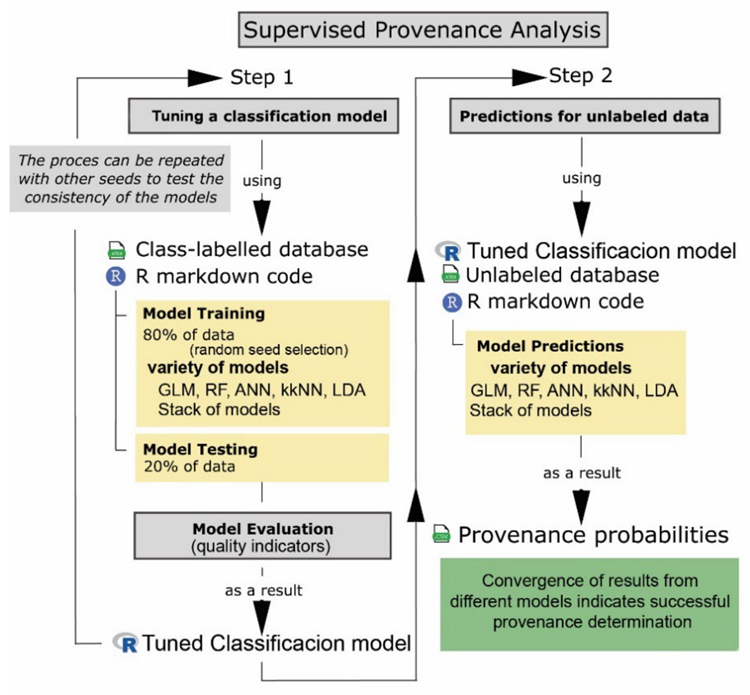
Schematic diagram of the two-step process (model tuning and predictions) to produce provenance probabilities for samples of unknown provenience using the R code to perform the “Supervised Provenance Analysis”. Image Credit: Anglisano, A et al., Sustainability
The research demonstrated an acceptable degree of accuracy for the supervised models. The authors have recommended that using a high number of reference samples, whilst providing improved algorithm training, would be an unsustainable approach. They have advised using smaller, balanced reference sample numbers.
In the long term, the presented approach, if generalized, can reduce the number of analyses needed to provide accurate provenance information for artifacts such as pottery shards. Once an exhaustive reference record has been achieved for particular regions, archaeologists only need analyze unknown samples without the need for reference samples. This will improve the sustainability of archaeological investigations.
Further Reading
Anglisano, A et al. (2022) Supervised Machine Learning Algorithms to Predict Provenance of Archaeological Pottery Fragments Sustainability 14(18) 11214 [online] mdpi.com. Available at: https://www.mdpi.com/2071-1050/14/18/11214
Disclaimer: The views expressed here are those of the author expressed in their private capacity and do not necessarily represent the views of AZoM.com Limited T/A AZoNetwork the owner and operator of this website. This disclaimer forms part of the Terms and conditions of use of this website.