Writing in the journal ACS Energy Letters, a team of scientists from Tsinghua University and Puri Materials, Inc. in China have applied a two-step machine learning method to improve the clustering and sorting of retired lithium-ion batteries.
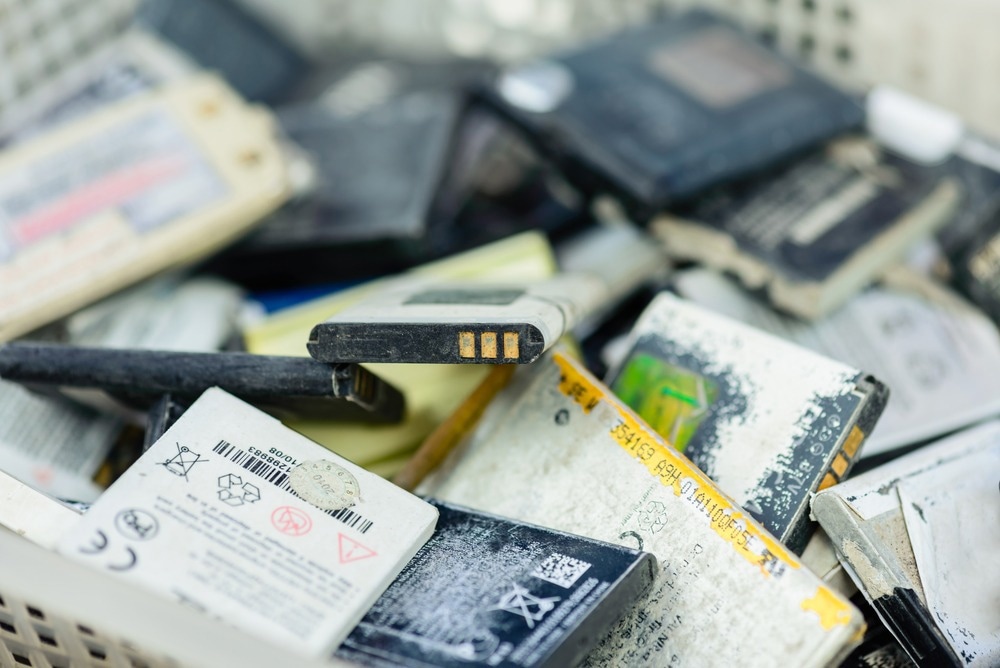
Study: Fast Clustering of Retired Lithium-Ion Batteries for Secondary Life with a Two-Step Learning Method. Image Credit: Vietnam stock photos/Shutterstock.com
Reusing Retired Lithium-ion Batteries
The electric vehicle industry has experienced rapid market growth since 2010, and with this rapid growth has come the consequent demand for lithium-ion batteries. This demand is predicted to double by 2025 and experience a fourfold growth by 2030. However, lithium resource scarcity is a key concern in the electric vehicle industry, facilitating the need for alternative, sustainable practices in lithium-ion battery manufacture.
Safe disposal of large-scale retired lithium-ion batteries has become a major global concern, as waste materials can possess potential ecotoxicity, causing serious environmental and health challenges. The heavy metals contained in their cathodes and corrosive acids used as electrolytes can leach into the environment if they are disposed of in landfills. Recycling raw materials is a key area of research currently.
One approach which could prove promising in terms of sustainability and cost reductions is to reuse retired batteries from electric vehicles. Safety concerns demand that batteries must be retired when their capacity reaches less than eighty percent of the initial capacity. However, whilst their reduced capacity limits their use in electric vehicles, many secondary applications can benefit from reused retired batteries.
Secondary uses of retired batteries include energy storage solutions, low-speed electric vehicles, and other applications where safety requirements are not quite as stringent. Repurposing retired lithium-ion batteries maximizes their value and extends their service lives. Furthermore, recovering the valuable heavy metals they contain reduces their environmental impact and has significant economic benefits.
Indeed, many studies have demonstrated the economic benefits of second-use batteries in applications such as residential photovoltaic energy/second-use energy storage systems. However, as the consistency of these batteries is worse than new devices, this can lead to performance degradation. Therefore, before reuse, batteries need to be classified and regrouped.
Clustering Methods
Currently, there is no standard accepted clustering method for retired lithium-ion batteries. Previous studies have employed long-time charge and discharge methods to elucidate their capacity and internal resistance. Current clustering methods include EIS, full charge-discharge approaches, pulse test methods, and computational approaches.
Estimating the remaining charge is a complex endeavor due to the influence of several factors on battery inconsistency and aging. These factors include ambient temperature and operating conditions. Batteries of the same type that display dissimilar aging can show different properties, such as voltage curves.
A suitable candidate method for evaluating these effects and providing sufficient charge estimation is the pulse test method due to its efficiency and the ability to accurately reflect aging processes. This can be combined with computational approaches to provide an accurate and efficient clustering and sorting method.
The Paper
A two-step sorting method is introduced in the paper which combines-short time pulse tests and computational approaches. The proposed classification method fully discharges the retired lithium-ion battery, which is then charged to 5% SOC. Short-time pulse tests are performed on the partially charged batteries, providing time savings of at least 80%. An accelerated aging test on a single battery was used to validate the pulse test method.
Six types of batteries were selected as experimental objects to verify the algorithm’s effectiveness when sorting the same lithium-ion battery type. Results indicated remarkably high classification accuracy for the study’s algorithm. When the pulse tests are combined with the machine learning clustering algorithm, batteries of similar performance can be rapidly clustered out, improving secondary battery clustering.
Supervised Gaussian process regression and unsupervised K-means clustering are employed to simultaneously sort and estimate the remaining lithium-ion battery capacity. K-means can effectively sort batteries of the same type, with the Gaussian mixture model further confirming this. Supervised Gaussian process regression possesses a correlation coefficient of over 98% for the remaining battery capacities.
The paper also demonstrated that increasing the number of training samples enhances the proposed approach’s capacity estimation performance. However, increasing the number of training samples is more time-consuming, facilitating a trade-off in terms of time cost and accuracy. Setting the proportion of training samples between 1/6 and 1/3 takes into account both time cost and accuracy.
Furthermore, an approach that utilizes an initial screening step followed by sampling of the training sets from each battery type can improve battery capacity estimation efficiency and performance. Finally, the research employed an automatic sorting machine to improve sorting efficiency. In summary, the paper provides key guidance for the fast clustering of retired lithium-ion batteries for secondary use.
More from AZoM: How are Bioplastics Made?
Further Reading
Ran, A et al. (2022) Fast Clustering of Retired Lithium-Ion Batteries for Secondary Life with a Two-Step Learning Method ACS Energy Letters 7, pp. 3817-3825 [online] pubs.acs.org. Available at: https://doi.org/10.1021/acsenergylett.2c01898
Disclaimer: The views expressed here are those of the author expressed in their private capacity and do not necessarily represent the views of AZoM.com Limited T/A AZoNetwork the owner and operator of this website. This disclaimer forms part of the Terms and conditions of use of this website.