In a paper recently published in the journal ACS Energy Letters, researchers used an active learning scheme assisted by quantum computing to devise a layered photonic structure-based visually transparent radiative cooler. This system combined machine learning, quantum annealing (QA), and active data production and was tested for its cooling effect.
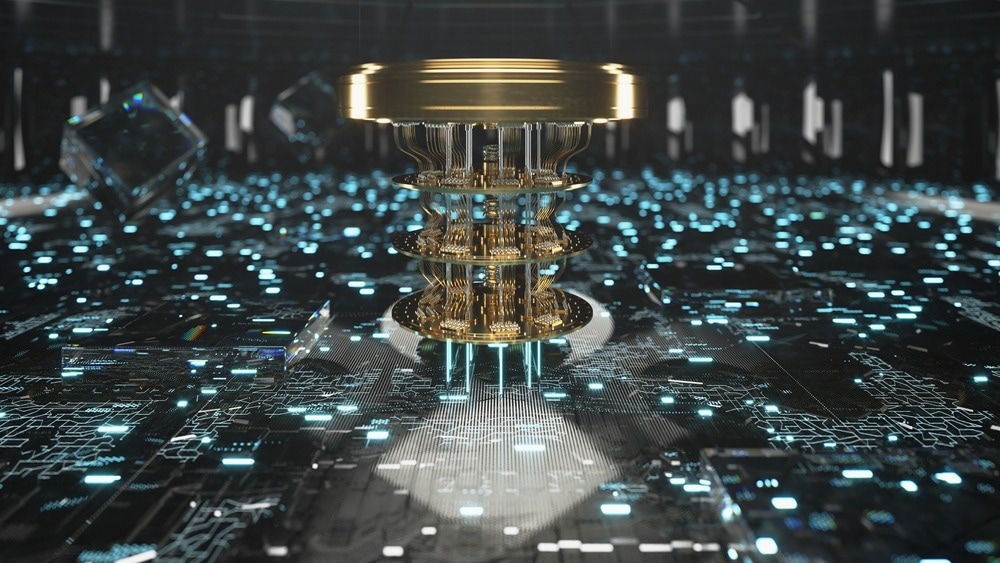
Study: High-Performance Transparent Radiative Cooler Designed by Quantum Computing. Image Credit: Bartlomiej K. Wroblewski/Shutterstock.com
Background
Passive radiative coolers, which use the atmospheric window (AW) to radiate heat to the colder outer space without requiring external energy, have generated a lot of attention. The majority of a building's cooling energy is dissipated through windows; hence, recent years have seen efforts to create transparent radiative coolers (TRC) to replace traditional window materials. In order to reduce the optical heating of an enclosed room, an ideal TRC should be able to efficiently block ultraviolet (UV) as well as near-infrared (NIR) photons in the solar spectrum.
To achieve the best radiative cooling performance through the AW, the TRC should also have a high emission efficiency within the mid/long-wavelength infrared (M/LWIR) domain. Given its basic planar geometry, accurately regulated dimensions, versatility in integrating multiple materials, and scalable manufacturing, layered thin-film photonic structures present a number of benefits for TRC development. Since quantum qubits can instantly evaluate the objective function to determine the global minimum via the quantum tunneling phenomenon, quantum annealing (QA), a quantum computing technique, proves to be excellent for optimization within a discrete space.
About the Study
In the present study, researchers described how QA and an active machine learning scheme were used to construct a high-performance TRC. The potential TRC in the study was composed of a planar multilayer (PML) photonic structure developed on a glass substrate having a polydimethylsiloxane (PDMS) top layer. The objective function associated with the optimization process was a figure-of-merit (FOM) that quantified how closely the developed TRC resembled the ideal TRC with respect to wavelength-dependent optical characteristics.
Each layer in the PML had a thickness of tl /Nl with a cumulative thickness of tl = 1200 nm. Enhancing PML complexity along with the number of Nl layers facilitated the creation of a TRC with greater performance, thus resulting in higher resolutions in the varying refractive index. Better optimum PML structures having lower FOM values could be designed by optimizing with higher Nl. To develop the TRC, the team employed the needle technique in conjunction with thickness refinement to represent the gradient-based optimization procedure. The TRC was constructed with traditional thin-film deposition methods based on the Nl = 24 that was predicted using QA-assisted optimization for the ideal structure. Two PML samples were produced, one of which had been annealed while the other had not.
Observations
In comparison to the Nl = 6 scenario, the irradiance transmitted for the highest Nl = 24 through the optimal designs predicted with QA-assisted optimization was significantly closer to that observed for the ideal TRC. The team noted that the transmission efficiency of the ideal structure having Nl = 24 was between 0.6 and 1.0 within the wavelength regime of over 1400 nm. Furthermore, the needle optimization revealed an optimized TRC having a FOM of 0.5166, which was almost 0.09 more than the FOM found by the QA-assisted optimization without taking into account the thickness optimization. Consequently, developing photonic structures derived significant benefits from QA-assisted optimization.
The team also observed that a 10-layer structure would be equivalent to the optimal PML since a few neighboring layers were made of the same material. The TRC that had undergone annealing was more transparent and visually homogeneous as compared to the unannealed TRC. Furthermore, in comparison to the TMM prediction, the constructed TRC had a reduced transmission efficiency within the 300 to 500 nm wavelength range. The results highlighted that annealing of the PML structure enhanced the film's crystallinity. Additionally, since the PDMS was on the PML, the TRC displayed near-unity emission efficiency in the AW.
When the FOM from the current study was employed as the comparative metric, it was discovered that the optimized TRC performed better than the triple-layer silver coating (TLSC). By creating a thermally isolated container with the constructed TRC mounted on the top side, the TRC's cooling capabilities could be tested. A glass slide was fitted at the aperture, and a second similar chamber was positioned next to it for comparison. It was observed that both chambers were heated by solar radiation, which was possible due to the TRC's transparency within the visible wavelength region.
Conclusions
To summarize, the team employed a QA-assisted active machine learning technique for window applications to develop a high-performance TRC. As compared to other coolers of a comparable design, the developed TRC had the lowest FOM values. The models demonstrated the enormous potential of the TRC to reduce the cooling energy used by buildings across the world.
Since they can be manufactured using cutting-edge deposition processes, the optimized PML structures present in the TRC may be upscaled for practical applications. Additionally, meta-materials used in optical, thermal, and mechanical applications, as well as general material design, can benefit from the QA-assisted optimization technique.
More from AZoM: What Materials are Used to Make Electric Vehicle Batteries?
Disclaimer: The views expressed here are those of the author expressed in their private capacity and do not necessarily represent the views of AZoM.com Limited T/A AZoNetwork the owner and operator of this website. This disclaimer forms part of the Terms and conditions of use of this website.
Source:
Seongmin Kim, et al., High-Performance Transparent Radiative Cooler Designed by Quantum Computing, ACS Energy Letters, 2022, 7, 4134-4141, DOI: https://pubs.acs.org/doi/full/10.1021/acsenergylett.2c01969