Machine vision and embedded vision systems both fulfill important roles in industry, especially in process control and automation. The difference between the two lies primarily in image processing capabilities and size: embedded vision systems deliver compact efficiency, and conventional machine vision systems provide high-performance and versatility. But the precise boundary between the two can be hard to pin down…
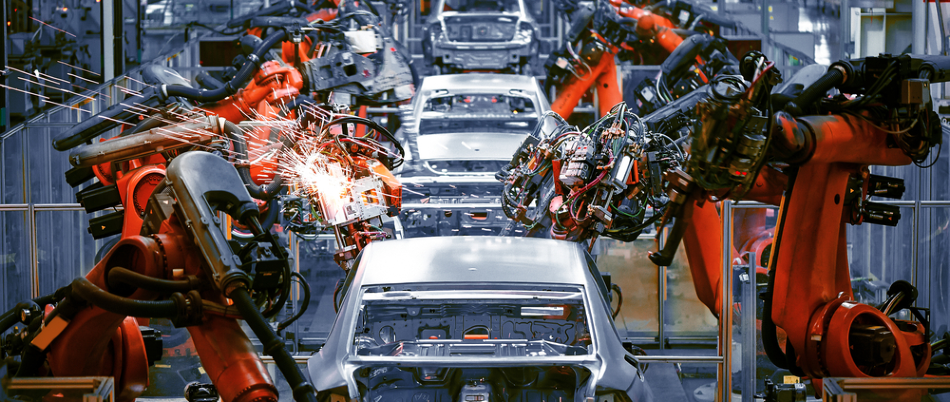
Image Credit:Shutterstock/Jenson
Many industries rely on machines that can automatically capture, process, and interpret visual information from the world around them in order to make decisions. Systems that enable machines to “see” are traditionally known as machine vision systems, and they are common in automobile manufacturing, medical imaging, electronics assembly, and packaging. In fact, any industry where visual inspection is important is a likely application of machine vision.
“Machine vision” does not refer to any one specific technology – rather it refers to any system where a machine interprets visual information. Such systems can glean useful information from a (usually) 2D view of the world, and this information is then used to produce an output.
For example, consider a system of cameras used to check for defects in components on a conveyor system. Typically, the cameras would take simultaneous images of a component as it passes a certain point, and these unprocessed images would then be relayed to a computer. Software corrects, processes and interprets the images to determine whether a specific component is defective. If a defect is detected, the system produces an output to remedy this, the offending component is automatically removed from the production line.
Variations on this concept are used throughout the industry to spot defects in all kinds of things, from sub-micron imperfections in semiconductor wafers to rotten tomatoes.1,2,3 Conventional machine vision systems (consisting of one or more image sensors connected to a server-class computer) use standard components, drivers, and image processing software. They are potentially bulky but cheap to set up, and capable of providing highly precise and versatile image processing.
Embedded vision systems differ from conventional machine vision systems in terms of how and where the image processing takes place. Embedded vision systems are all-in-one devices, consisting generally of a camera mounted onto an image processor. With everything on-board, a PC is not required – image capture and processing can take place within a single device.
Examples of embedded vision include pattern and object recognition in autonomous vehicles and drones. In these applications, space is at a premium: embedded vision forgoes the bulkiness and weight of fully-fledged machine vision systems but still provides useful decision-making capabilities. For example, a simple embedded system in a drone could be used to detect the distance of the ground, enabling altitude correction in response.
As a rule of thumb, embedded vision systems are less powerful but much easier to use and integrate than conventional machine vision systems. Embedded vision systems can cost more to set up – hardware is often tailored to the specific application – but are highly compact and incur lower operational costs due to decreased computational requirements and low power consumption.
Embedded vision systems can technically be considered a subset of machine vision systems, but differences in capabilities and applications have kept them more or less distinct from each other. Until recently, embedded systems have not been capable of the performance of PC-based systems.
But, as the amount of computing power that can be fit into a given space has reliably increased over the years, the PCs used in machine learning systems are getting smaller while the onboard processors in embedded vision devices are getting more powerful. As a result, the difference between conventional machine vision and embedded vision is becoming less distinct. Indeed, the processors in today’s embedded vision systems have the equivalent processing power to machine learning systems from only a few years ago.4
ALVIUM® Technology
Allied Vision’s range of Alvium cameras was developed to bridge the gap between conventional machine vision and embedded vision.
Where standard embedded image sensors rely on the processing power of a host processor, Alvium cameras include their own Image Signal Processors and intelligent Image Processing Libraries. Whatever signal is captured by the sensors is processed inside the camera, and then this is sent to the host. As a result, drivers are independent of sensors used, resulting in a versatile, easily upgradeable, and future-proof embedded vision system.
Traditional machine vision systems involve transmitting and processing a high volume of largely redundant image data.6 The Alvium range of cameras drastically reduces the computational burden on the host processor by carrying out onboard image correction including defect pixel correction, deblurring, mirroring, and cropping.7
The range consists of the 1500 series and 1800 series: high-performance industrial-grade cameras designed to enable long-lasting sensing solutions in any environment.
The Alvium 1500 series is a camera module for embedded vision system development. It was built with connectivity and integration at the forefront, with only one MIPI CSI-2 driver required to cover every camera in the series. The Alvium platform features an efficient power management system for minimal power consumption. The camera is available as a bare board, or with an integrated lens mount. Alvium cameras with integrated lens mounts all undergo a precise sensor alignment process to guarantee sharp, distortion-free image capture.
The Alvium 1800 series is a crossover between a conventional machine vision camera and an embedded camera. As well as featuring all the embedded image processing capabilities of the 1500 series, it can be used with an embedded host processor or connected to a PC via MIPI CSI-2 or USB3 Vision interfaces.
Click here to find out more about the Alvium camera range.