The term intralogistics was initially defined in 2003 as the “organization, control, implementation and optimization of the internal flow of materials, information flows and the turnover of goods in industry, trade, and public facilities.”1
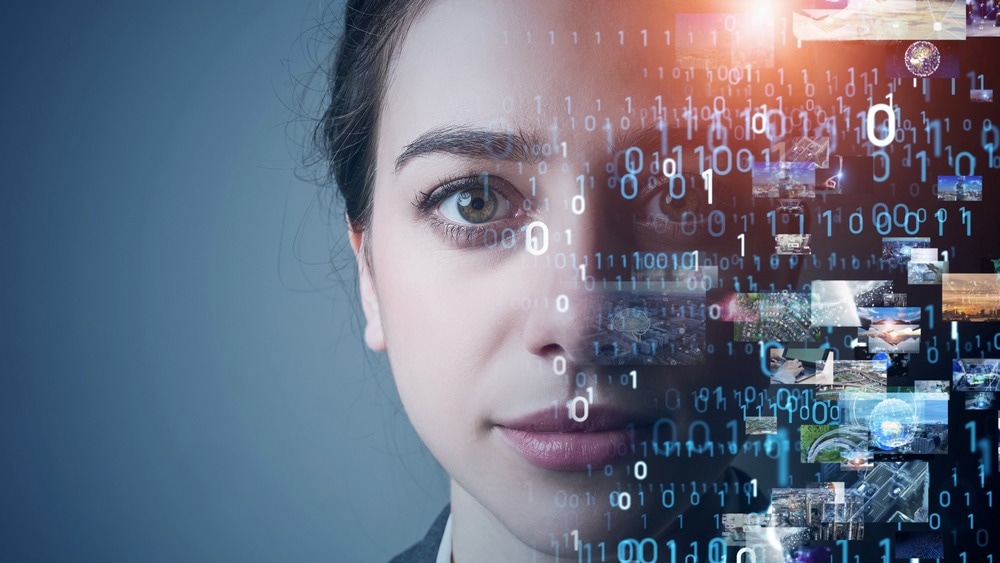
Image Credit: metamorworks/Shutterstock.com
Since then, the rapid development of automation technologies has led to the emergence of machine vision cameras as essential components in the creation of automated solutions for intralogistics.2
This article explores the importance of machine vision cameras in such solutions while discussing some of the challenges faced in the field.
The numerous applications of machine vision cameras in autonomous guided robots (AGRs) and autonomous guided vehicles (AGVs) will also be highlighted. The efficacy of Allied Vision’s Alvium camera platform in addressing current challenges will also be outlined.
The Importance of Machine Vision Cameras in Automated Intralogistics Solutions
Machine vision uses computer vision techniques to resolve real-world industrial problems associated with visual elements. Machine vision cameras provide a robust visual perception capability, allowing automated systems to efficiently “observe” and precisely interpret their surrounding environment.
This capability extends to tasks such as detecting surface quality, assessing size and positioning and identifying potential defects. In intralogistics, where accurate object detection, identification, and tracking are essential, machine vision cameras facilitate real-time monitoring and analysis.
In addition to capturing high-resolution images or video footage, these cameras can detect electromagnetic radiation far beyond the visible spectrum, which advanced algorithms can process to extract important information.
Due to their incredible versatility, machine cameras extend beyond traditional manufacturing applications, finding utilization in automated intralogistics solutions across food processing, medical, and agricultural fields.2
Machine Vision Cameras in AGVs and AGRs
AGRs and AGVs depend on machine vision cameras, often supported by additional sensors, to coordinate a variety of functions. While navigating an environment, cameras capture images of the vehicle’s surroundings. These images are then evaluated using algorithms.
To establish the vehicle’s position and orientation, relevant features can be extracted and then compared with pre-mapped data. This enables AGRs and AGVs to accurately identify and localize themselves, which is crucial for locating products in a warehouse and avoiding obstacles.2,3
Likewise, for the detection and recognition of objects, images captured by machine vision cameras are assessed using sophisticated algorithms. This allows AGRs and AGVs to identify large objects such as pallets.4
Nuances in color, shape, and textures can also be assessed to support the identification of organic materials like vegetables and fruits.5 This information can then guide a vehicle’s actions, for example—picking and placing goods.
Given their extensive application, machine vision cameras must meet various requirements to reliably and accurately conduct the numerous functions demanded of AGRs and AGVs. These conditions are described below:
Appropriate Image Processing Algorithms
Due to the intricacies of image processing algorithms, vision-based tracking can result in delays. Consequently, AGVs are often faced with the challenge of reconciling restricted computational capacity with the need for resource-intensive image processing.
To attain maximum output, optimize operational efficiency, and reduce time delays, the operator should choose the optimal algorithms.6
Accurate Camera Calibration
Geometric camera calibration—a process that involves estimating the camera’s parameters needed for capturing an image of a scene onto the imaging sensor—is crucial in machine vision.
These parameters can be categorized as intrinsic (which outlines the camera’s internal geometry, such as focal length and lens distortions) or extrinsic (which determines the camera’s orientation and position relative to real-world coordinates.7
Calibration must additionally be conducted for deficit pixel correction (DPC) and fixed pattern noise (FPN) to guarantee that image sensors produce accurate, high-quality, and consistent images for a variety of applications.3
Sufficient Image Resolution
Higher image resolutions are typically preferred since they can detect smaller and, thus, more distant markers. Subsequently, a robot identifying an object tag or a vehicle tracking tape on the floor can operate at higher resolutions with greater precision.8
Reliable Connectivity System
The AGV’s connection system is responsible for seamless communication between the vehicle and various components, including the sensors, camera, and larger IT infrastructure.
Its primary responsibilities involve real-time data transmission, collaboration with other systems, and remote administration. To sustain the integrity of the connectivity system, operators should maintain network interface cards, high-quality wireless connection protocols, and access points.9
Challenges in the Field: Framing the Future
Although machine vision cameras have revolutionized intralogistics, their application to AGRs and AGVs introduces numerous challenges.
The intralogistics environment is complex, characterized by several obstacles, dynamically shifting shelf loads, and irregular lighting conditions. It is challenging to integrate cameras capable of proficiently performing in dynamic scenarios.
Ensuring real-time processing of camera data is crucial for quick navigation decisions. However, achieving real-time processing while simultaneously managing significant data volumes can cause substantial pressure on computational resources.6
This complexity is compounded further by possible interoperability problems. Limited operability between machine vision cameras and AGVs can cause compatibility concerns that impair overall system performance.10
Cameras designed for mobile vehicles must adhere to specific specifications to operate effectively onboard a vehicle in potentially rugged environments. These specifications include:
- Small size and low weight
- Shock and vibration resistance
- Low energy consumption
- Extended temperature range and waterproof housing
To meet safety-relevant requirements, fast image transfer and multi-camera applications necessitate:
- Low latency - This helps to avoid collisions with humans and objects
- Established long cable systems - These connect multi-cameras to one host
- Low system overhead for lean system structure
- Multi-camera options for a 360-degree view3
The Alvium Camera Platform for Intralogistics
Allied Vision is an industry-leading manufacturer of machine vision cameras, offering a variety of innovative solutions for intralogistics applications. Among its product portfolio, the Alvium camera series fulfills requirements through its numerous camera models that are relatively lightweight, compact, and have low power consumption.
Based on ALVIUM® Technology, there are six interface options for several requirements. Offering a diverse selection of high-quality lens mounts, image sensors, housing options, and spectral sensitivities, the Alvium platform delivers remarkable flexibility with over 200 cameras.3
The Alvium camera platform, therefore, provides numerous advantages to intralogistics applications. These benefits are described below. 3
Versatility
The platform’s configurability and interface options make it adaptable to various systems and environments, thereby boosting its compatibility and ease of integration.
Due to their sugar cube format, a shared SDK, and a single CSI-2 Driver, the cameras can be integrated into any vision system and subsequently upgraded or replaced with any other Alvium camera.
High-Quality Imaging
Offering innovative sensor technology and a broad spectrum of resolution options, Alvium cameras deliver high-quality images across visible, near-infrared, short-wave infrared, and ultraviolet spectra. This is vital for accurate object detection, recognition, and inspection.
Interface Options
Each Alvium interface possesses unique strengths and is well-suited for various scenarios. Factors such as the distance between the camera and the host system, data transfer requirements, and whether networking between multiple cameras within a system is required, determine the choice of interface.11
With six different interface options, Allied Vision Technologies’ Alvium platform provides the ability to fulfill the diverse demands of the machine vision and imaging industry:
- Alvium G1 with the GigE vision interface
- Alvium G5 with the 5GigE vision interface
- Alvium U with the USB3 vision interface
- Alvium C with the MIPI CSI-2 interface
- Alvium FP3 with the FPD-Link III interface
- Alvium GM2 with the GMSL2 interface
Reliability and Durability
Manufactured with industrial-grade hardware, Alvium cameras are designed to endure challenging intralogistics environments, guaranteeing reliable and consistent performance.
Image Preprocessing
Allied Vision’s ASIC with integrated ISP finalizes image preprocessing, offering an optimized image to the host computer. This minimizes the burden on the host and frees up the CPU for critical algorithms required for AGRs and AGVs.
Final Snapshot: Machine Vision Cameras in Automated Solutions for Intralogistics
Machine vision cameras have become essential tools in the creation of automated solutions for intralogistics. Their ability to capture visual information allows AGRs, AGVs, and other automated systems to conduct tasks autonomously.
The Alvium camera platform is flexible and possesses several unique, innovative features. This platform tackles industry challenges by enabling the seamless integration of machine vision cameras into intralogistics processes, thus improving accuracy, efficiency, and productivity.
References and Further Reading
- Arnold D. (2006) Intralogistik: Potentiale, Perspektiven, Prognosen. Springer-Verlag. doi.org/10.1007/978-3-540-29658-4
- Smith ML., et al. (2021) The quiet revolution in machine vision - a state-of-the-art survey paper, including historical review, perspectives, and future directions. Computer in Industry. doi.org/10.1016/j.compind.2021.103472
- Allied Vision. (2023). Available at: https://www.alliedvision.com/en/ (accessed 27 July 2023)
- Molter B, et al. (2018) Real-time Pallet Localization with 3D Camera Technology for Forklifts in Logistic Environments. 2018 IEEE International Conference on Service Operations and Logistics, and Informatics (SOLI). doi.org/10.1109/SOLI.2018.8476740
- Al-falluji, RA. (2016) Color, Shape and Texture based Fruit Recognition System. International Journal of Advanced Research in Computer Engineering & Technology. Corpus ID: 212461219
- Zhang T, et al. (2021). Improved Camshift Algorithm in AGV Vision‑based Tracking with Edge Computing. The Journal of Supercomputing. https://doi.org/10.1007/s11227-021-03974-3
- El Ghazouali S, et al. (2022). Optimised calibration of machine vision system for close range photogrammetry based on machine learning. Journal of King Saud University - Computer and Information Sciences. https://doi.org/10.1016/j.jksuci.2022.06.011
- Oščádal P, et al. (2020). Smart Building Surveillance System as Shared Sensory System for Localization of AGVs. Applied Sciences. https://doi.org/10.3390/app10238452
- AGV Network. The 7 AGV Components That Bring Autonomous Robots to Life. Available at: https://www.agvnetwork.com/agv-technology/agv-components (Accessed on 17 August 2023).
- Cupek R, et al. (2020). Autonomous Guided Vehicles for Smart Industries – The State-of-the-Art and Research Challenges. Springer Nature. https://doi.org/10.1007/978-3-030-50426-7_25
- AlliedVisionTV. (2016). Which camera interface is the right one for my application? Available at: https://www.youtube.com/watch?app=desktop&v=Co2PR1wDRLk

This information has been sourced, reviewed and adapted from materials provided by Allied Vision.
For more information on this source, please visit Allied Vision.