In this article, AZoM talks to Kate Kemsley, head of the Core Science Resources team at the Quadram Institute, about their research, and how they use NMR for labeling in the food industry.
Would you tell us about how Oxford Instruments and The Quadram Institute came to collaborate?
The Quadram Institute has worked with high-resolution, high-field NMR (Nuclear Magnetic Resonance) technologies for some time. In 2012, The Institute became interested in benchtop technology. This work remains ongoing, and thanks to the support of the UK's Technology Strategy Board, a collaboration was started with Oxford Instruments which is also still ongoing.
Why is Quadram’s work around vegetable oil composition essential?
While working with vegetable oils, our primary goal was ascertaining whether or not benchtop NMR could offer the kind of information necessary for labeling.
Standard reference methods exist for this purpose, such as GC-FID (Gas Chromatography – Flame Ionization Detector), but our aim was to develop a much more rapid method that offered minimal consumable costs, with no sample preparation requirements.
We acquire neat oils directly from the bottle, placing these into the NMR tubes to allow analysis. This approach means that our test only takes five minutes per sample, from beginning to end.
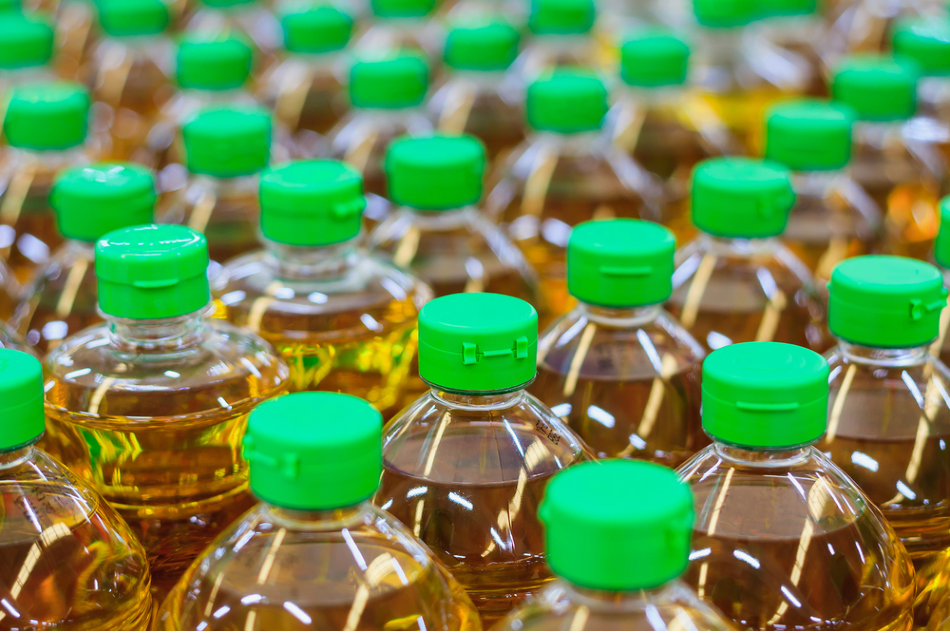
Image Credit: Kwangmoozaa/Shutterstock.com
What can benchtop NMR show us about vegetable oils?
We employ benchtop NMR in the analysis of different parts of the molecules of vegetable oils. Examining NMR signals from the molecules’ olefinic and bisallylic regions supplies invaluable information about the degree of unsaturation of the oils.
Signals from the glyceride region assist in the quantification of the measurements because these signals are generally constant across samples. Once the fatty acid profile of oil has been obtained, you can use that profile for a wide range of applications.
Can you tell us about your work in developing a screening method to determine the authenticity of argan oil?
Developing a screening method required a supply of argan oils which were of guaranteed provenance. Oils were supplied via our existing contacts at the Food and Agriculture Organization of the United Nations; an organization with a specific interest in affordable screening methods for agricultural food products’ authentication.
The sample collection was comprised of hundreds of authentic argan oils, and a number of potential adulterants.
Our team obtained proton NMR spectra from all of the oils before processing them. Since argan oil is a natural product, a degree of variation was anticipated between the spectra, but our results were found to be consistent with the literature values for the various fatty acid proportions.
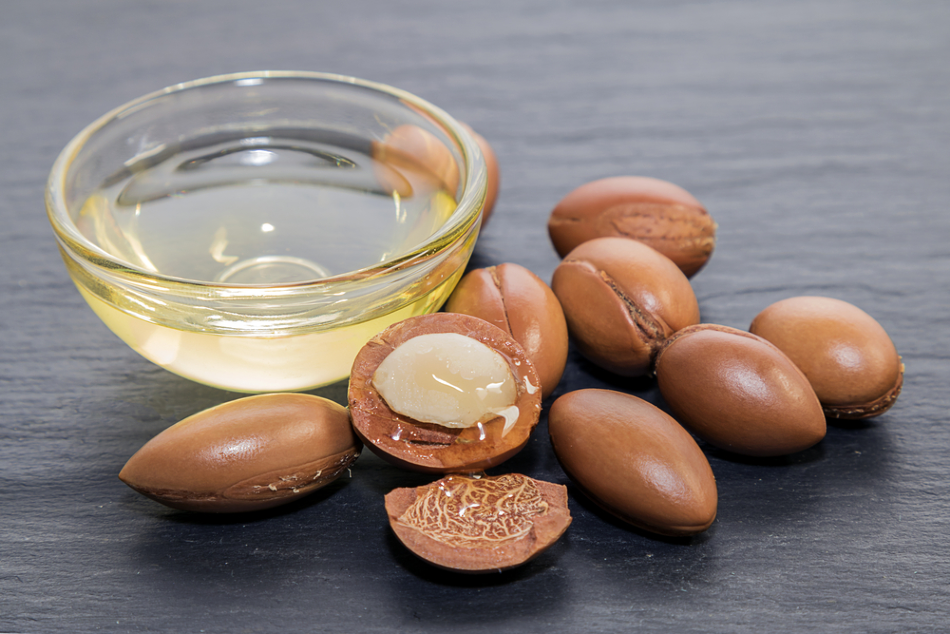
Image Credit: Juanamari Gonzalez/Shutterstock.com
We had to exclude some samples that had oxidized while they were stored. Once these had been removed, we treated the remaining data statistically in order to place a confidence region around the group. This region was where we would anticipate finding 95 % of the authentic argan oils.
The confidence region allows us a direct means of detecting mixtures with other oils, down to concentrations of approximately 20 % - a useful percentage for a rapid screening tool.
Should the fatty acid profile of a contaminating oil be highly similar to that of argan oil, however, this approach would not be sensitive enough.
To address this issue, we used information from across the whole of the spectrum, rather than just from a small number of peak integrals. We tackled this problem using single-class modeling, also known as outlier or anomaly detection. Once again, we defined a boundary to reject or accept items from the class, but this approach used the whole spectrum, with the boundary being much more mathematically complex.
You also work with coffee. Why is coffee another key product in the food industry that should be authenticated?
The coffee supply chain is incredibly long. It is only possible to grow coffee in tropical countries, but coffee is processed and consumed across the globe. Valuable Arabica coffee is highly vulnerable to fraud as once the beans have been roasted and ground, it is virtually impossible to detect differences via visual inspection. An analytical test is essential in these cases, and our goal was to see out if benchtop NMR could provide a solution to this problem.
When a proton NMR spectrum of the lipophilic phase is obtained from a ground roast coffee, this spectrum will include many signals from primary metabolites, minor compounds, and breakdown products resulting from the roasting process. At 60 MHz, a large number of these peaks can be seen to overlap, meaning that only the more prominent compounds can be picked out.
In contrast, however, caffeine features a number of clear, easily identifiable signals. And, most crucially, a small isolated peak can be found which originates from a compound named 16-O-methylcafestol (16-OMC). This is particularly interesting because this compound seems to exist in extracts from Robusta but not Arabica coffees.
This finding could lead to a rapid, simple, and affordable authenticity test.
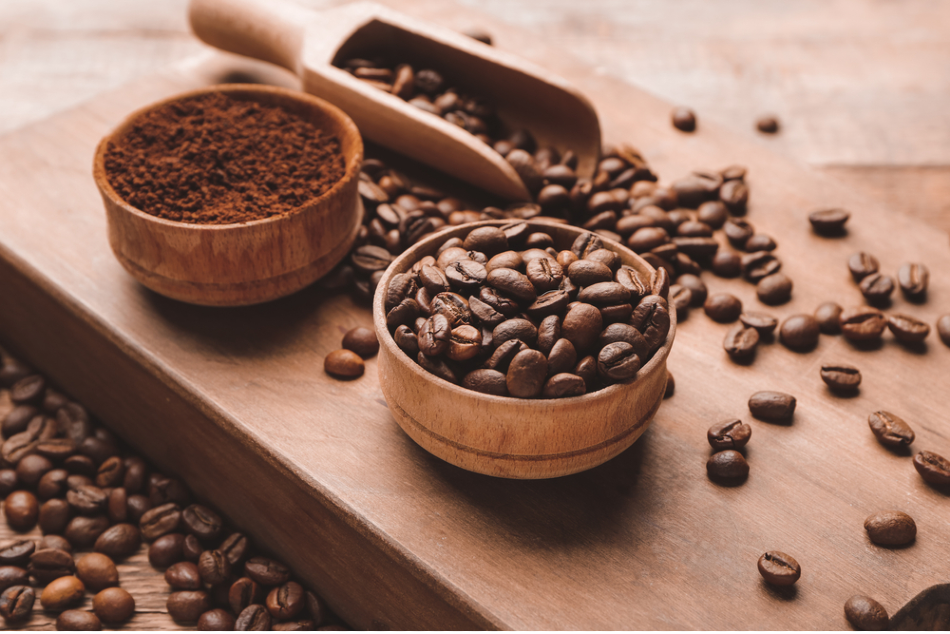
Image Credit: Pixel-Shot/Shutterstock.com
Can you tell us about the use of benchtop NMR to identify psychoactive drugs?
This project began around two years ago. We were approached and asked to develop a benchtop NMR test which could facilitate the fast identification of novel psychoactive drugs, more commonly known as legal highs or designer drugs.
This work has since expanded to also explore small-molecule pharmaceuticals, as well as other controlled substances. This project was carried out in collaboration with Manchester Metropolitan University because the university possesses one of very few UK licenses allowing it to synthesize and store these substances.
My role in this project has centered around automated data analysis. The challenges here, especially when working with seized drug samples, can be linked to unknown concentrations of active ingredients, degradation of samples from uncontrolled storage, and samples that have been mixed with a range of other substances.
Thankfully, a highly accurate routine now exists facilitating the identification of a wholly unknown sample. This sample could be comprised of a single compound, or a mixture either with other active substances or excipients.
The approach utilizes pattern matching and a database of reference compound database. The approach is centered around the identification of numerous nearest neighbors, employing additional manipulations to deal with mixtures where required. There is currently a patent application in progress for this method.
The process takes a spectrum of the unknown sample and pre-processes it to only extract the specific regions of interest. So, for example, we can exclude peaks from the solvent if this is required.
The next stage in the process involves a comparison of the sample spectrum to a database of known compounds. This database includes pharmaceuticals and controlled substances, as well as a wide range of excipients. Several hundred reference spectra are checked, with the closest matches ranked in order of similarity.
If there is no close match found, this could indicate that the sample is a mixture. Where this may be the case, combinations of the closest single compound matches will be used to synthesize a further collection of mixture spectra via spectral addition. In the final step of the process, the sample spectrum will be compared against these mixtures, enabling the best match to be identified.
Our work was published lately in an open-access paper. During the study, we looked at over 400 seized samples in total. This research allowed us to confirm that the use of benchtop NMR with pattern recognition has the potential to be a highly effective tool in the rapid screening of seized street drugs. Within this study, in particular, we were able to accurately identify controlled substances in 99 % of the samples examined.
About Professor Kate Kemsley
Professor Kate Kemsley is the head of the Core Science Resources team at the Quadram Institute, based in Norwich, UK. Her group helps to provide a substantial platform of technologies to support the institute’s work, such as NMR, microscopy, mass spectrometry, and flow cytometry.
Disclaimer: The views expressed here are those of the interviewee and do not necessarily represent the views of AZoM.com Limited (T/A) AZoNetwork, the owner and operator of this website. This disclaimer forms part of the Terms and Conditions of use of this website.