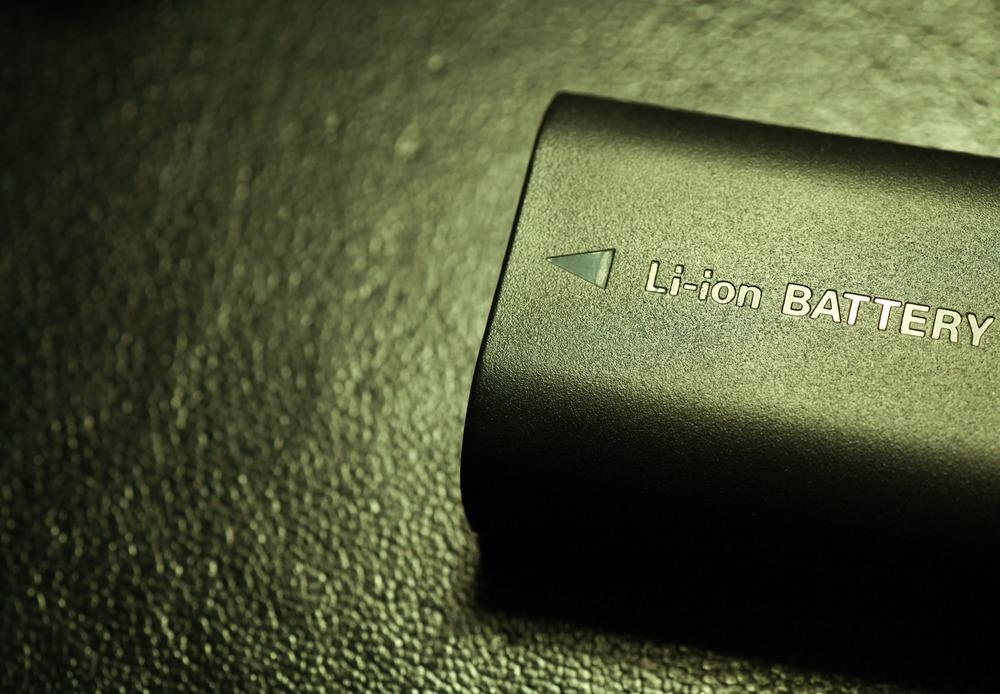
Image Credit: Janaka Dharmasena/Shutterstock.com
According to a new study in the journal Nature Materials, researchers from Stanford University have harnessed the power of machine learning technology to reverse long-held suppositions about the way lithium-ion batteries charge and discharge, providing engineers with a new list of criteria for making longer-lasting battery cells.
This is the first time machine learning has been coupled with knowledge obtained from experiments and physics equations to uncover and describe how lithium-ion batteries degrade over their lifetime.
How is Machine Learning Used in the Study?
Machine learning accelerates analyses by finding patterns in large amounts of data. In this instance, researchers taught the machine to study the physics of a battery failure mechanism to design superior and safer fast-charging battery packs.
The Problems with Fast-Charging Lithium-Ion Batteries
Fast charging can be stressful and harmful to lithium-ion batteries, and resolving this problem is vital to the fight against climate change. A fast-charging solution could be used to make electric cars much more competitive with conventional combustion-engine vehicles.
The study focused on lithium-ion battery electrodes, which comprise tightly packed nano-sized particles. During charging and discharging cycles, lithium ions exchange between the anode and cathode, leaking into the particles and streaming back out. This continuous exchange makes particles enlarge, shrink and fracture, progressively minimizing their ability to hold a charge. A rapid charging process exacerbates this situation.
To view this system in very fine detail, the study group observed the actions of cathode particles created from nickel, manganese, and cobalt - a blend called NMC, which is one of several most common in electric vehicle battery cells. These particles soak in lithium ions during discharge and shed them during charging.
The study team used extremely bright X-rays from a synchrotron to obtain a global view of particles going through a rapid charging phase. They then used a scanning X-ray transmission microscope to view individual particles.
Finding a Solution to Fast-Charging Issues
Results of the experiments, in addition to information from scientific models associated with fast charging, were integrated into machine learning algorithms. The computer essentially picked out or formulated the right equations, and therefore the correct physics.
Scientists have previously thought that variations between electrode particles were unimportant and that their capability to keep and release ions was restricted to how quickly lithium could move within the particles. According to this previous understanding, lithium ions stream out and into all particles simultaneously, at approximately the same pace.
However, the new study demonstrates that the particles themselves manage the pace of lithium ions leaving cathode particles during charging. A few particles shed many of their ions straight away, while others discharge hardly any or no particles at all. The quick-release particles also carry on streaming ions at a quicker rate than slow-release or non-releasing neighboring particles. This superior performance of individual particles, which the study team described as “the rich getting richer”, had not been previously identified.
Using high-powered observation tools, the study team began to understand the ways lithium ions move within a lithium-ion battery. The unequal discharging and charging cycles put a lot of necessary strain on the electrodes and diminish their operating lifetimes. Understanding this system on a foundational level is a vital step in solving the rapid charging roadblock.
The researchers say their new research may potentially lead to improvements in the cost, durability, storage capacity, and other key attributes of battery cells for a broad array of applications, from powering electric vehicles to the industrial-scale storage of clean energy.
Building on Previous Research
The new Stanford University study expands on two previous developments by the same research team. In one previous study, the researchers used a more common application of machine learning to drastically speed up battery testing methods. In the other previous study, machine learning was used to narrow down many possible charging techniques to discover which methods work best.
Even though these studies helped researchers advance their understanding and decrease the time required to gauge battery lifetimes by 98%, they did not expose the primary physics or chemistry that causes large differences in how long individual batteries last, which the most recent study did reveal.
The Stanford team said merging the findings of all three studies may potentially cut the time required to deliver a groundbreaking battery for electric vehicles, possibly by as much as two-thirds faster. The new blended strategy may also be used to develop grid-scale systems necessary for greater usage of intermittent clean energy sources, such as wind and solar power.
Further Reading and Resources
Chui, G. In a leap for battery research, machine learning gets scientific smarts. SLAC National Accelerator Laboratory. [Online] Available at: https://www6.slac.stanford.edu/news/2021-03-08-leap-battery-research-machine-learning-gets-scientific-smarts.aspx
Vollrath, M. New machine learning method from Stanford, with Toyota researchers, could supercharge battery development for electric vehicles. Stanford News. [Online] Available at: https://news.stanford.edu/2020/02/19/machine-learning-speed-arrival-ultra-fast-charging-electric-car/
Golden, M. AI accurately predicts the useful life of batteries, Stanford and MIT researchers find. Stanford News. [Online] Available at: https://news.stanford.edu/2019/03/25/ai-accurately-predicts-useful-life-batteries/
Disclaimer: The views expressed here are those of the author expressed in their private capacity and do not necessarily represent the views of AZoM.com Limited T/A AZoNetwork the owner and operator of this website. This disclaimer forms part of the Terms and conditions of use of this website.