AZoM speaks with Michael Foshey, Mechanical Engineer in the Computer Science and Artificial Intelligence Laboratory at Massachusetts Institute of Technology. Michael is part of a team of researchers at MIT that has developed a data-driven system that accelerates the process of discovering new 3D printing materials with multiple mechanical properties. The research was supported by BASF.
Can you give our readers an overview of your recent research?
In our recent research, we focus on applying machine learning and automation methods to accelerate the development of new types of materials and processes.
How does incorporating a machine learning approach aid in the discovery of additive manufacturing materials?
In this work, our optimization algorithm uses a model to navigate different designs and uncover a set of formulations that have optimal trade-offs in compression strength, toughness and modulus. This model is based on previous data from our previous tests and does not rely on any prior knowledge about the materials science behind the formulation.
Compared to conventional material development methods, this model relies much less on having prior knowledge about the underlying chemistry. This system could be especially beneficial in cases where chemistry is not well known.
A multiobjective optimization algorithm was used as part of your research. Can you give our readers an insight into how it was implemented?
In this work, we utilized an algorithm that finds a set of formulations with optimal trade-offs in compression modulus, toughness and compression strength. The optimization algorithm works by exposing a set of design parameters in the formulation that can be tweaked to attain better material properties.
While the algorithm is working, it selects design parameters that it predicts will have optimal material properties. The sample with that formulation is then 3D printed, tested and reported back to the algorithm. The algorithm predicts another new formulation with optimal properties with these updated results, and the cycle continues.
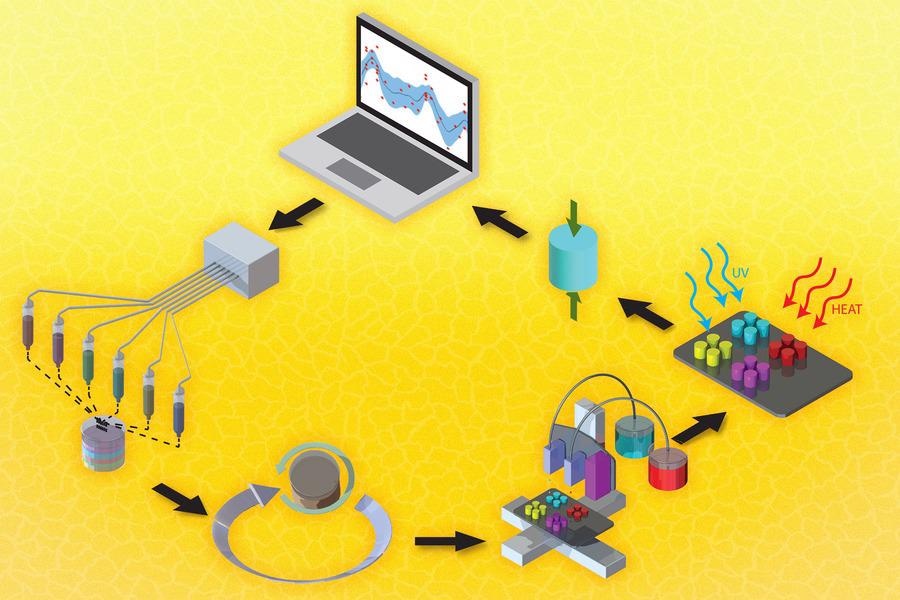
Researchers at MIT and BASF have developed a data-driven system that accelerates the process of discovering new 3D printing materials that have multiple mechanical properties. Image Credit: Computer Science and Artificial Intelligence Laboratory/ MIT.
One of the outcomes of using the multiobjective optimization algorithm is the creation of better-performing materials. How is this outcome achieved?
While the optimization is working, it develops a model that can predict formulations with optimal material properties. As it tests more formulations, the model becomes more accurate and can better predict material properties.
The algorithm is coupled with a semiautonomous fabrication platform. What is the role of the semiautonomous fabrication platform?
The semiautomated fabrication platform is a system we developed to produce and test formulations efficiently. In this work, we tested 150 distinct formulations in quadruplicates. The semiautomated fabrication platform allowed us to prepare these efficiently.
Different autonomous systems have recently been proposed to accelerate material development and substantially reduce human labor. What benefits can multiobjective optimization algorithms bring in comparison to recently proposed developments?
They tend to complement each other when used together, as we have in this work. Multiobjective optimization is a data-driven process that works best when you can test a large number of samples. Autonomous systems for materials development typically drive down the cost of experimentally testing materials, meaning that it is less expensive to try a large number of samples, making multiobjective optimization algorithms more effective.
What are some of the main benefits of satisfying multiple objectives simultaneously?
For most applications, materials need to have high performance for multiple objectives, such as high strength and low cost. Optimizing for multiple objectives allows us to find materials that have optimal trade-offs in important parameters.
What are your predictions for the future of machine learning use when investigating materials for additive manufacturing use?
I think machine learning will continue to have a significant impact on materials development in general. Developing new materials is paramount for solving societal scale problems like curbing the amount of carbon dioxide humans produce. More materials need to be developed than ever before, and one way to do this is to automate the development process. This work exemplifies how humans can use machine learning techniques to enable systems to develop new materials without human input.
What is next for the team at MIT?
We would like to continue to develop our optimization pipeline and expand it to materials and process development for more applications. We are also developing an open-source optimization software package called AutoOED for anyone to start using multiobjective optimization algorithms for their application. Anyone interested in using it can download the software for free at autooed.org.
Where can readers find more information?
The full paper can be viewed here. The open-source optimization software package, AutoOED, can be accessed here. And for more information on research that I am involved in, your readers can find out more here.
About Michael Foshey
Michael Foshey is a Mechanical Engineer with the Computational Design and Fabrication Group. Foshey’s research interests include: 1) Developing new types of additive manufacturing processes and systems that unlock new applications for 3D printing. 2) Implementing machine learning algorithms to discover, optimize, and understand new high-performance materials and processes. 3) Developing novel types of sensors to understand better how humans and robots interact with their environment.
Disclaimer: The views expressed here are those of the interviewee and do not necessarily represent the views of AZoM.com Limited (T/A) AZoNetwork, the owner and operator of this website. This disclaimer forms part of the Terms and Conditions of use of this website.