Understanding defect dynamics and evolution in high entropy alloys (HEAs) s is complicated due to the wide and intricate configurational space in HEAs. Machine learning techniques have significant potential in defect detection in HEAs.
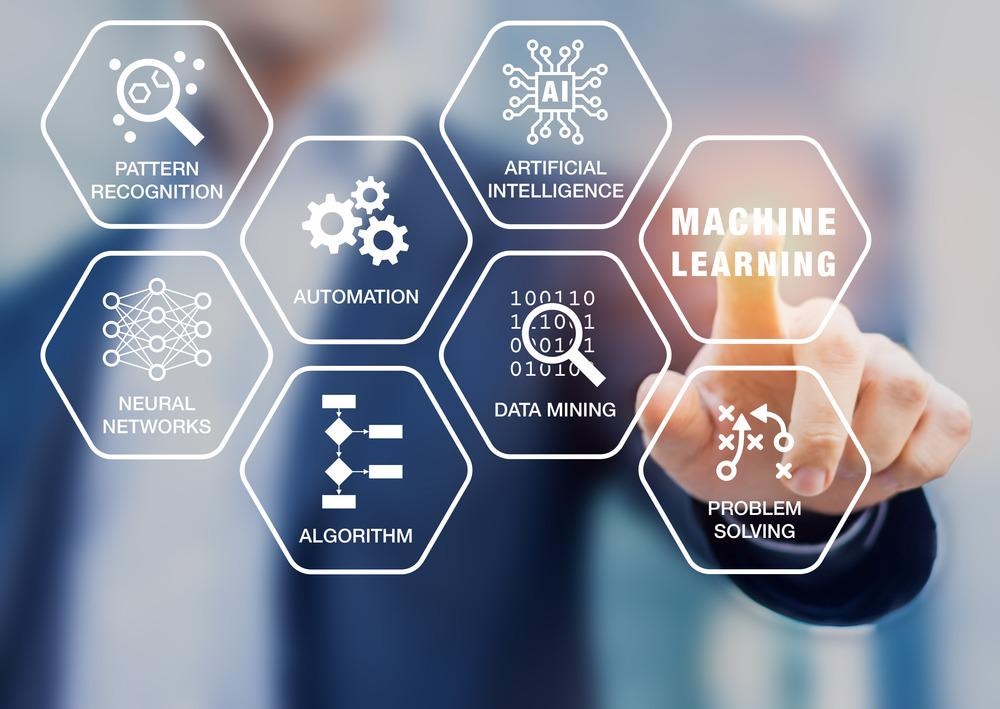
Image Credit: NicoElNino/Shutterstock.com
Chemical synthesis of high entropy alloys (HEAs) with different metallic compositions and characteristics is a developing field of research. HEAs are alloys made by combining five or more elements in variable volumes and HEAs have four core properties, which are sluggish diffusion, high entropy, lattice distortion, and cocktail effects. The primary properties of HEAs are corrosion resistance, biocompatibility, high specific strength, and thermal stability.
HEAs can be used in multiple industries such as defense, space, nuclear, energy generation, and stealth applications, and are used to develop coating materials, electromagnetic shielding materials, and catalytic materials.
Machine learning (ML) uses experimental data to predict future material qualities, and the application of ML in materials design and discovery is a rapidly growing research area. The databases used in ML for HEA applications are based on surrogate models, iterative frameworks (adaptive design and learning), autonomous synthesis, atomistic simulations, and text mining. ML models such as support vector model (SVM), kernel-based, extreme learning machine (KELM), and deep neural network (DNN) can be used for defect detection in HEAs.
The main advantage of ML is that it leverages implicit correlations between various descriptors and observations, shows great potential in identifying the controlling elements for irradiation damage, and modeling the local environment dependence of defect evolution.
Machine Learning and Defect Detection in HEAs
Defects in HEAs significantly impact deformation mechanics and strength. Recent studies use machine dynamics (MD) and machine learning to investigate the dynamics of defects in HEA alloys. The main focus of recent studies is to identify defects in face-centered cells and body-centered cells of HEAs.
ML methods and models can be used to interpret the type of defects based on the irradiation response of HEAs. Irradiation response is the output generated when the HEAs are exposed to radiation. The critical aspect is that during the measurement process of irradiation response of HEAs, the response generated due to non-ionizing radiation must not be included, which can be done effectively with ML models and methods.
The interatomic potential describes the interaction between the pair of atoms or within the group of atoms in HEAs. The bonding of atoms occurs during the formation of HEAs and the mathematical model of interatomic potential of bonding of atoms of HEAs has repulsive and attractive forces. Defects that occur due to the interatomic potential can be identified using the ML models as the accuracy of the prediction of defects mainly depends upon the solution of mathematics models.
In HEAs, defect evolution (growth of a defect) occurs immediately after the defect formation, and the ML-based models provide accurate information about the defect evolution in HEAs. Based upon the requirement of the application, the information of defect evolution supports the process of identification of appropriate HEAs and rejects inappropriate HEAs
The most challenging problem in HEAs is building the small supercell with randomly distributed atoms and simulating each atom's actual position in a highly stoichiometric chemical environment without any defect. Various mathematical models based upon ML are used to construct HEA supercells and identify defects developed in HEAs.
Conclusion and Future Scope
The use of machine learning for the production and defect detection of HEAs is still in its infancy, and some challenges must be resolved before industrial applications. The most critical challenge is the availability of open data and open-source software, and the consistency challenge with International Standard Organization (ISO) standards of HEAs.
Additionally, the process of developing force fields or potential energy functions for MD simulations is also difficult due to a higher number of stoichiometric possibilities and the effect of thermodynamic parameters during the formation of HEAs.
Another difficulty is generating a defect-free supercell in HEAs using ML methods and models. While the special quasi-random structural model is frequently utilized for ML-based solutions, it is unknown whether it accurately replicates the experimental observation of HEAs.
The density and functional theory of ML simulation of HEAs involve multiple chemically complicated elements and require the higher processing power of computers to solve them due to which their ML simulation remains restricted to a limited number of atoms in HEAs.
The scalable molecular dynamics (used for simulation of HEAs) is based upon complex calculations and requires high-performance computers, but a significant constraint in the application of scalable molecular dynamics is the restricted number of universal potential functions that can be used to adequately represent metallic bonding in the HEA.
The future scope in defect detection and discovery of HEAs is using artificial intelligence and ML methods. In addition to this, the results of artificial intelligence and ML methods must be validated with further experimental investigation.
References and Further Reading:
Shijun Zhao, Application of machine learning in understanding the irradiation damage mechanism of high-entropy materials, Journal of Nuclear Materials, Volume 559, 2022,153462,ISSN 0022-3115, https://www.sciencedirect.com/science/article/pii/S0022311521006826?via%3Dihub
Katiyar, N.K., Goel, G. & Goel, S. Emergence of machine learning in the development of high entropy alloy and their prospects in advanced engineering applications. emergent mater. (2021). https://link.springer.com/article/10.1007%2Fs42247-021-00249-8
Disclaimer: The views expressed here are those of the author expressed in their private capacity and do not necessarily represent the views of AZoM.com Limited T/A AZoNetwork the owner and operator of this website. This disclaimer forms part of the Terms and conditions of use of this website.