This article considers the use of artificial intelligence to help security professionals protect IoT systems.
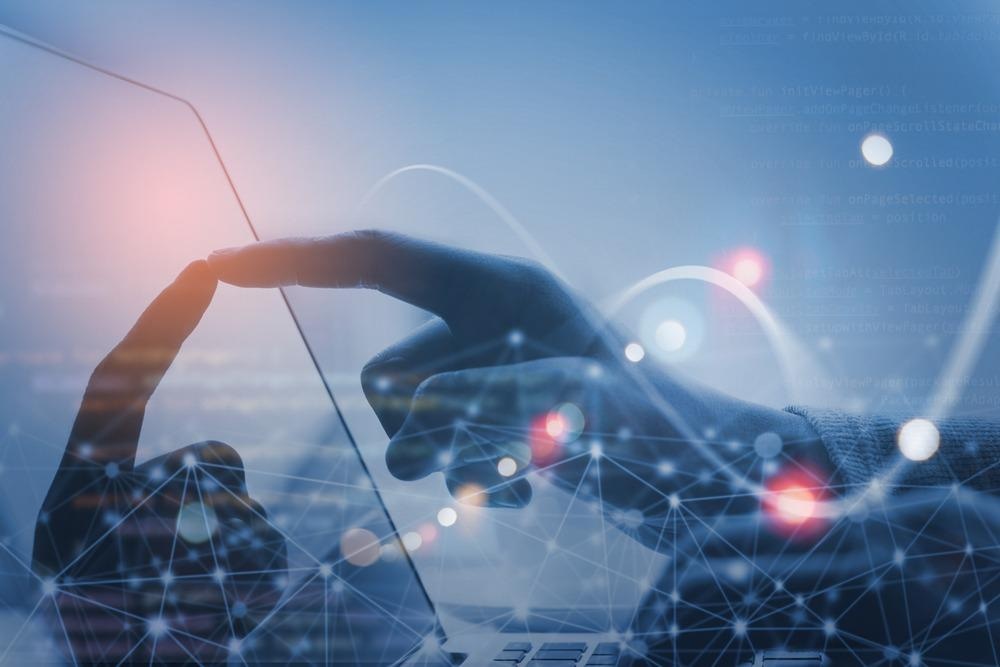
Image Credit: TippaPatt/Shutterstock.com
The Internet of Things (IoT) is still in its infancy, but threats to IoT systems and their potential for harm have become quite sophisticated. There are two reasons for this: the value of data and systems that IoT vulnerabilities can give access to; and the high number of potential attack vectors – discrete elements of IoT networks that are vulnerable to foul play.
Artificial intelligence (AI) software and algorithms help security professionals to wrest control of this technological battleground back from hackers and protect the IoT as it reaches maturity.
What is the IoT?
Only introduced in 2008, the Internet of Things and IoT systems are still fairly nebulous concepts, subjects of numerous and sometimes conflicting definitions. However, the IoT can be practically understood as a network or networks of machines and computing devices that can record and exchange data and perform digital and physical tasks with that data without human intervention.
For consumer IoT, this usually presents itself with an interfacing hub – often a mobile app or a central hub device. The interfacing hub then works with fringe devices that record data or perform tasks (or both) and connect with the rest of the IoT over wireless networks.
Industrial IoT (sometimes known as IIoT, and a key enabling technology for the much-heralded Fourth Industrial Revolution, or Industry 4.0) is in many ways a simple progression from the supervisory control and data acquisition (SCADA) networks powering industrial automation from the mid-twentieth century.
However, digitizing more aspects of industrial processes and driving networks with more powerful computers and AI software is currently delivering a step-change in industrial output in many sectors.
IIoT could be transformative in many industries, but two sectors have so far made the most use of smart technology for industrial automation: manufacturing (especially automotive) and logistics (although the AIs powering the world’s logistics systems are struggling to cope with the intense demands of global freight, as evidenced by worldwide supply shortages in 2021).
Vulnerability of the IoT and IIoT
IoT networks are particularly vulnerable to cyberattacks. The network effects that make them valuable also present numerous attack vectors – channels through which hackers can access and exploit data in their favor. Also in hackers favor is the lack of standardized security features and regulatory frameworks to protect IoT systems and devices.
Many individual devices that make up IoT networks have poor security on them or were not designed for connections to public networks. Attackers can breach network security through the IoT devices themselves (connected sensors, actuators, and hub devices), the networks they communicate on, and the mobile apps, online platforms, and cloud storage used to interface with them.
Breaches in an IoT network can reveal private information including video and audio from people’s homes. Consumer IoT processes a lot of data that is valuable to criminals.
Hacking IoT can tell burglars if people are at home; it can give useful information to fraudsters including IDs and financial activity and can be used to make sophisticated, targeted phishing attacks that are likely to work (these are known as spear-phishing attacks, and whaling attacks when there is a high-value target such as a politician or corporate executive).
In industry, the ramifications of vulnerable IoT systems are potentially much more costly. Attackers can leverage control over IIoT systems for ransom against firms losing thousands of dollars for every minute of lost production. In extreme scenarios, IIoT powering vital systems like electric grids, public transport, and healthcare networks could be vulnerable to malevolent state and non-state actors.
AI Helps Cybersecurity Professionals Protect IoT
The cybersecurity industry makes use of cutting-edge AI technologies to keep the odds in its favor against IoT attacks.
Machine learning (ML) is an AI application that instructs computers to process data and test hypotheses about the data to develop an “understanding” of it, which provides useful analysis if the ML algorithms are given the right parameters to work with and quality data.
Further Reading: How Can AI Overcome 3D Printing Defects?
Naïve Bayes is one popular algorithm for IoT security. It classifies data based on anomalous activities in data, which are assumed to come from independent events rather than a single attack. The algorithm, named after the Bayesian theorem on which it is based, has to be trained by human supervisors before it sets its target classes of activity, but then it can be let loose on live datasets to find and flag anomalous and potentially malevolent activity.
Decision trees are AI processes that make complicated sets of rules somewhat automatically. They are also trained with human supervision, but analyze each piece of data according to an iterative division of rules that ultimately provide an answer (often to a simple binary question like “attack or normal”).
Some decision tree-based AIs employ rule-learning techniques to develop their sets of rules automatically. This increasing level of artificial “agency” is made possible with increasing levels of computing power, including new computer architectures like quantum computing.
K-nearest neighbor (k-NN) techniques create classes to find based on the Euclidean distance between new pieces of data and data that has already been classified in the dataset. A geometric proxy is configured to set k-NN algorithms up, then they can get to work to discover patterns in large datasets.
Artificial neural networks (ANNs) use a mathematical equation to read large amounts of data and output target values through numerous iterative steps through nodes in the network. This method is inspired by the way electric signals pass through synapses in brains, although its similarity to organic brains is more illustrative than it is informative.
ANNs can change their decision-making models and analysis frameworks as they are presented with new information. This makes them more dynamic than other AI security measures, more adaptable when faced with changing tactics.
Cutting Edge AI for IoT Security
AI research is breaking new barriers in IoT security today. ANNs have been set loose for anomaly detection, sending data to human engineers to check. Researchers have also identified AI as a potential control method for IIoT security, identifying and also evaluating the damage of security breaches.
It is clear that the IoT needs to be looked after while it is still immature. AI tools are crucial for keeping the IoT safe, and allowing it to reach its full potential.
References and Further Reading
Cañedo, J., and A. Skjellum (2016). Using machine learning to secure IoT systems. 14th Annual Conference on Privacy, Security and Trust (PST) Available at: https://doi.org/10.1109/PST.2016.7906930.
Farivar, F., M. S. Haghighi, A. Jolfaei, and M. Alazab (2020). Artificial Intelligence for Detection, Estimation, and Compensation of Malicious Attacks in Nonlinear Cyber-Physical Systems and Industrial IoT. IEEE Transactions on Industrial Informatics. Available at: https://doi.org/10.1109/TII.2019.2956474.
Kuzlu, M., C. Fair, and O. Guler (2021). Role of Artificial Intelligence in the Internet of Things (IoT) Cybersecurity. Discover Internet Things. Available at: https://doi.org/10.1007/s43926-020-00001-4.
Radanliev, P., D. De Roure, K. Page, et al. (2020). Cyber risk at the edge: current and future trends on cyber risk analytics and artificial intelligence in the industrial internet of things and industry 4.0 supply chains. Cybersecurity. Available at: https://doi.org/10.1186/s42400-020-00052-8.
Radanliev, P., D. C. De Roure, J. R. C. (2020). Nurse, et al. Future developments in standardisation of cyber risk in the Internet of Things (IoT). SN Applied Sciences. Available at: https://doi.org/10.1007/s42452-019-1931-0.
Wang, S. and Z. Qiao (2019). Robust Pervasive Detection for Adversarial Samples of Artificial Intelligence in IoT Environments. IEEE Access. Available at: https://doi.org10.1109/ACCESS.2019.2919695.
Disclaimer: The views expressed here are those of the author expressed in their private capacity and do not necessarily represent the views of AZoM.com Limited T/A AZoNetwork the owner and operator of this website. This disclaimer forms part of the Terms and conditions of use of this website.