Widespread in aerospace, laminated fiber-reinforced polymer matrix composites are also increasingly used in other sectors, including the automotive industry. The identification of optimal cutting parameters for a given tool composite pair is of utmost importance, as this can significantly reduce component non-conformance. During final aircraft assembly, surface delamination during machining results in 60% of part rejections.
A significant experimental effort is required for understanding and controlling delamination, which typically manifests in systematic drilling and the subsequent analysis of a thousand holes. The Intellegens deep learning software, Alchemite™, can reduce this experimental time by quantifying complicated nonlinear tool-composite relationships.
Alchemite™ guides tooling design and selection before an experimental campaign by facilitating the analysis of complex data relationships. From sparse and noisy data based on 80% fewer experiments than a usual testing program, Alchemite™ delivered useful predictions of future tooling performance in a study at the University of Sheffield Advanced Manufacturing Research Centre (AMRC), enabling further experimental cost savings. It was also able to identify which features in the system were and were not relevant to performance – information of value in designing future experiments.
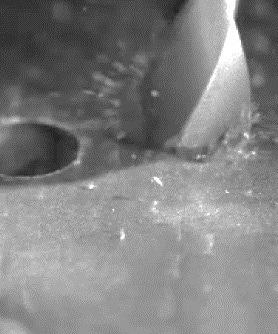
Image Credit: Intellegens Limited
Laminated fiber-reinforced polymer (FRP) matrix composites are seen in industries with a drive towards high-performance, lightweight components - such as aerospace - due to their highly-tailorable design and excellent mechanical properties.
Although this tailorability increases design options, it can negatively impact costs, productivity, and sustainability during manufacture. This can be particularly apparent in machining, where FRP part-specific defects occur.
A common cause for prescribing overly-conservative cutting tool use limits is process uncertainties that result in a large, unpredictable defect generation based on part quality criteria. An application-specific approach is required to identify the most effective cutting strategies due to the wide array of available tool designs and workpiece material configurations.
Using an exhaustive, wide-boundary, DoE-based approach, optimal cutting parameters can be found, with slow and costly testing needed to identify absolute tool life limits.
A novel machine learning-based method is established by the work described in this article to predict tool life from start-of-life performance data, minimizing experimental cost and time. As the original dataset was sparse, with 82% of the target data missing, the project was particularly challenging.
Intellegens’ novel machine learning software, Alchemite™,1 builds comprehensive models from sparse and noisy data and leverages the unique insights of deep learning. In this study, the AMRC provided tooling time series data on 55 drill/composite pairs, recording 23 machining responses, including hole quality metrics and in-process measurements. Using its intuitive drag-and-drop interface, this data was easily uploaded into the Alchemite™ Analytics software.
The deep learning model was trained on the tooling dataset. Alchemite™ was able to train a model with a high coefficient of determination of 0.73, despite the missing 82% of data. The core Alchemite™ algorithm was used in combination with various data pre-processing steps to achieve this high accuracy and reduce the inherent noise.
As Figure 1 shows, these steps included data grouping, followed by aggregation. Analysis showed that, although data from a typical testing dataset of over 1,000 holes was available, 200 data points were adequate for providing deep insight into a tool’s future cutting performance with the right aggregation.
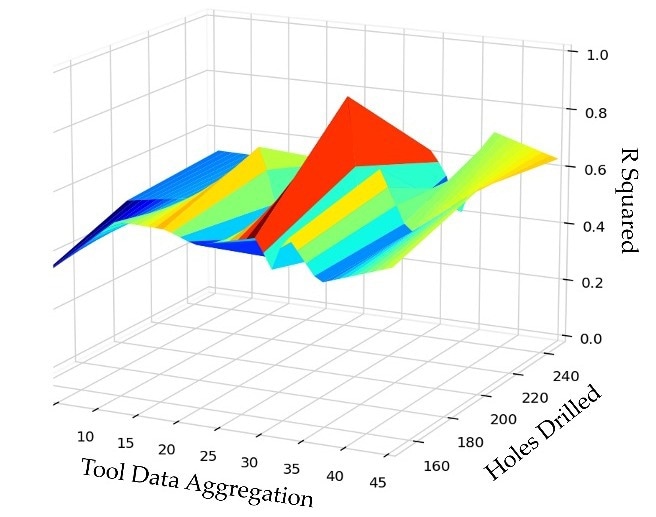
Figure 1. Alchemite™ identifies the optimal holes drilled for predicting future tool performance. The plot shows how the accuracy of the model (R-squared) can be improved by optimising data aggregation and that data from 200 tests (‘holes drilled’) enables high accuracy. Image Credit: Intellegens Limited
Alchemite™ created a tool-composite model with excellent predictive power by using insight from sparse data to quantify underlying, complex nonlinear property/property relationships.
Tool life can be estimated thanks to the ability to accurately predict exit delamination (Figure 2) for a future number of drilled holes and the impact of factors such as material selection on tool life and tooling geometry.
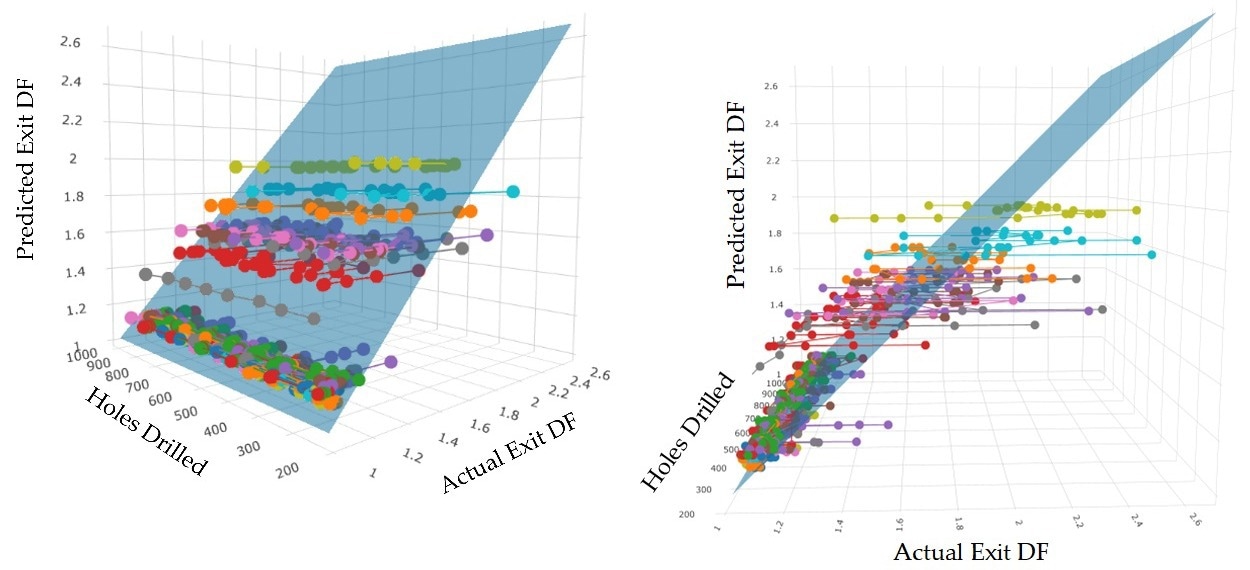
Figure 2. Two projections of a 3D plot showing how the accuracy of predicting exit delamination factor (DF) varies with the number of holes drilled. Each colour in the connected points indicates a unique tool-composite pair. Image Credit: Intellegens Limited
Ensuring that unsuitable tools are not unnecessarily tested and that only the most promising candidates are taken forward for more comprehensive tooling trials can inform the design stage of an experimental campaign.
Making useful decisions based on only 20% of the typically-acquired performance data allows progress to be made based on far fewer tests, resulting in up to 80% reductions in the direct costs associated with testing, such as material wastage, machining and technician time, as well those associated with equipment maintenance and overhaul.
Identification of variables that were irrelevant to predicting tool life performance, allowing additional experimental streamlining, was enabled by the use of explainable AI tools in Alchemite™, such as the importance chart.
The predictive accuracy of Alchemite™ increases in proportion to the amount of data added. As the tool-composite database increases, therefore, the optimum tool for a new composite component can be determined with increased certainty.
Consequently, this project could be extended to identify optimal tool-composite pairs with a more guided approach, reducing experimental costs and time. More significantly, a deeper understanding of the high-dimensional feature space will result from populating the tool-composite database with new experimental data, leading to a continual cycle of improved operational performance.
About the AMRC and Intellegens
A network of world-leading research and innovation centres, The University of Sheffield Advanced Manufacturing Research Centre (AMRC) works with manufacturing companies from around the globe. To help the industry develop economic methods of controlling drilling-induced delamination, the AMRC has undertaken a number of historic CFRP and CFRP/metallic stack drilling trials.
A unique machine learning solution for real-world experimental and process data problems in industrial R&D and manufacturing is provided by Intellegens. Based on a methodology that originated in the University of Cambridge, the Alchemite™ deep learning software can model sparse, noisy data, where other machine learning approaches cannot succeed.
It has accelerated innovation in various sectors and areas, including developing formulated products, alloys and component design, additive manufacturing, drug discovery, and optimizing chemical processes.
References
- “Alchemite™ deep learning - solving complex problems with real-world data”, Intellegens White Paper, 2021 [Online]. https://intellegens.com/alchemite-deep-learning-solving-complex-problems-with-real-world-data/

This information has been sourced, reviewed and adapted from materials provided by Intellegens Limited.
For more information on this source, please visit Intellegens Limited.