OCAS, a leading steel research center, is a joint venture between the Flemish regional government and ArcelorMittal. In this case study, OCAS employed Alchemite™ deep learning to model the behavior of different steels.
Even minor improvements in steel performance or the cost of processing and raw materials can result in enormous economic benefits. OCAS is also constantly seeking to reduce the time-consuming and costly physical experiments and analysis required to achieve these results.
The project aimed to leverage microstructural imaging information to explore the so-called 'PSP triangle' (which stands for Processing-Structure-Properties) for steels. A key aim was to learn whether steel microstructures, a treasure trove of information, could be exploited through machine learning.
The project applied the Alchemite™ deep learning software1 from Intellegens. Designed for use in materials research and development, the features of Alchemite™ include its ability to generate machine learning models from real-world, sparse, noisy experimental data and the accuracy with which it quantifies the uncertainty associated with its predictions.
Alchemite™ was trained on an OCAS steel dataset consisting of 23 unique treatments, four material chemistries, nine processing variables, and microstructural image data. The microstructure images were encoded for Alchemite™ using a combination of deep learning and principal component analysis.
The project investigated the accuracy of the machine learning model and how training the model using different combinations of image, process, and property data varied the results. It also aimed to establish a greater understanding of the impact of image magnification on the accuracy of predictions and to see how sensitive the predictions were to different parts of the dataset.
Alchemite™ was ultimately used to optimize experimental processing parameters to best meet specific property requirements for the steels after model assessment.
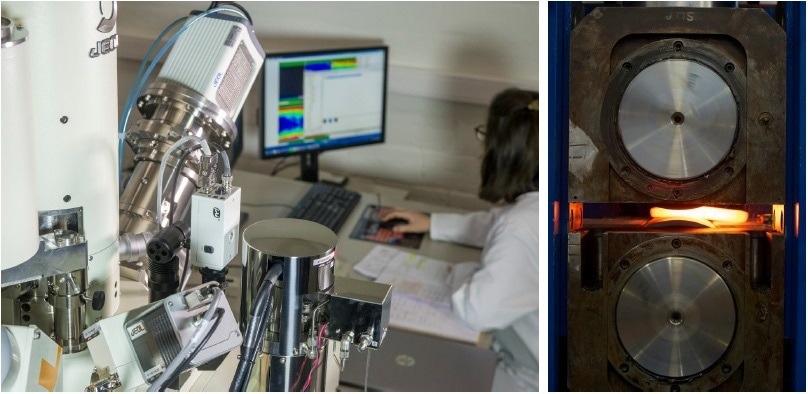
Figure 1. Microscopy (left) and a rolling process (right) at OCAS. Image Credit: Intellegens Limited
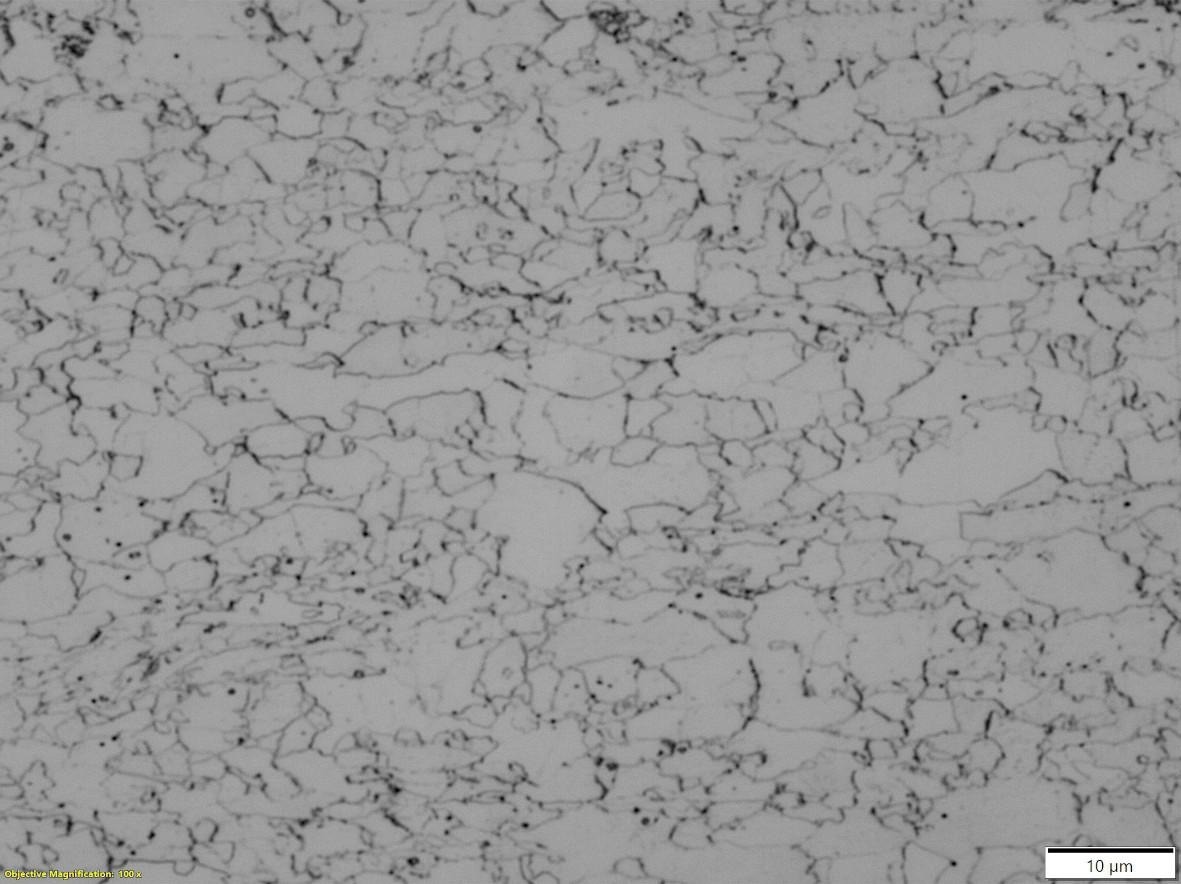
Figure 2. Sample microstructure image from the project. Image Credit: Intellegens Limited
Outcomes
- In property prediction, Alchemite™ could build models with strong accuracy (R2values > 0.8). By including microstructure image data, accuracy was improved.
- Model accuracy was best when using microstructure images at one particular magnification as an input. This may enable the range of images captured in future tests to be reduced, saving time and cost. It also suggests that features which resolve at this magnification are the most significant.
- It was possible, in some circumstances, to remove up to 30% of the image data without significantly impacting model accuracy. This suggests that not every sample needs to be imaged – again, this could reduce cost.
- An understanding of the importance of including processing and microstructural data in different circumstances was developed by the project.
- In the quest to understand which specific inputs were most useful in predicting performance, 'explainable AI' tools were helpful. Figure 3 shows one example, a sensitivity plot.
- A set of processing parameters to achieve target properties for the steel were designed by Alchemite™.
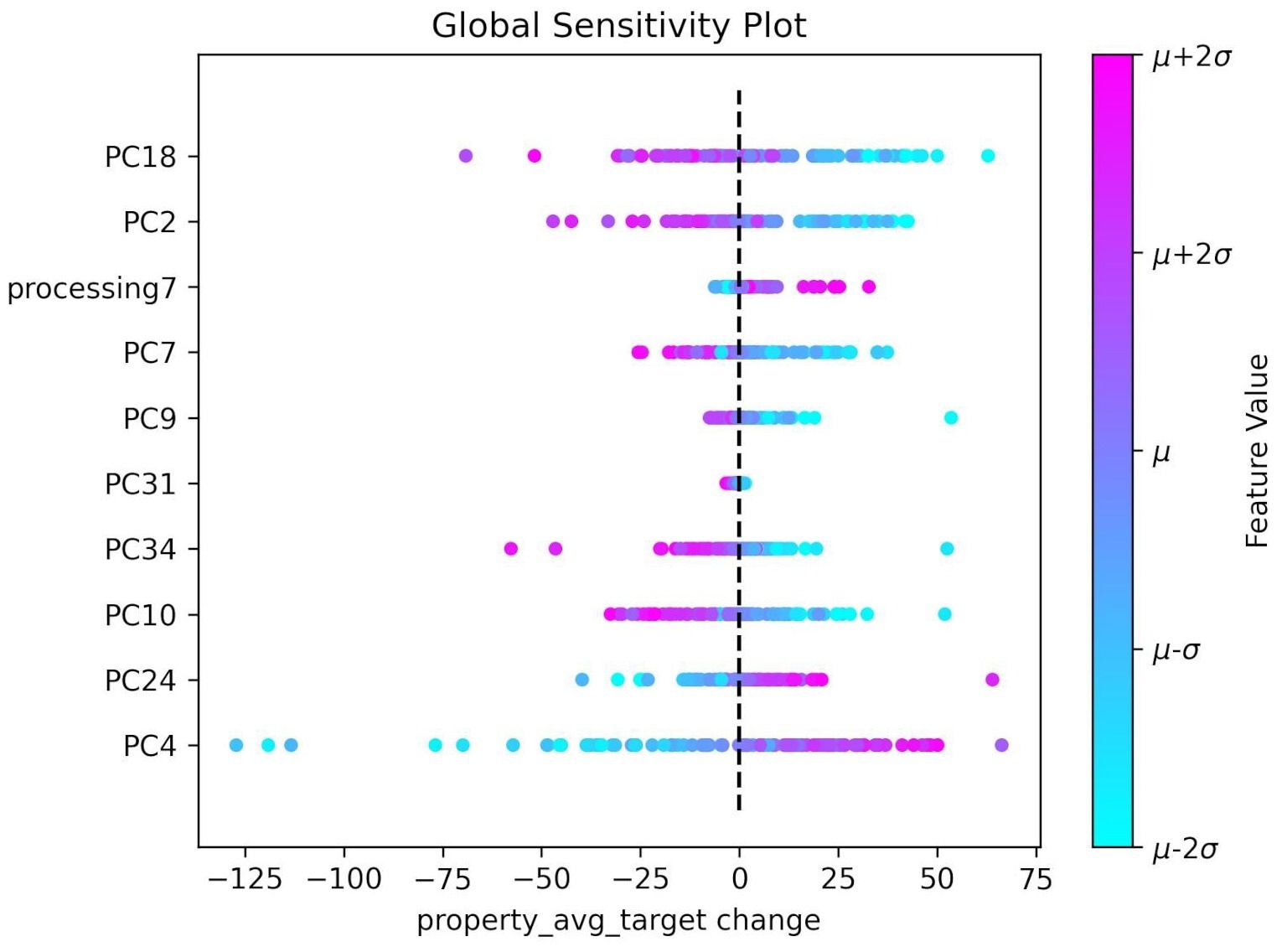
Figure 3. Global sensitivity plot – an ‘explainable AI’ tool in Alchemite™. The plot enables the overall impact of each variable on the target property to be assessed. Image Credit: Intellegens Limited
Summary
The benefits of the project were assessed by the OCAS project team:
- Alchemite™ deep learning allows for the extraction of hidden information from microstructural images.
- Models with good predictive power are provided by Alchemite™, from processing or microstructure to properties.
- When supported by analytics features, such as importance charts and sensitivity plots, and through providing reliable uncertainty estimates on predictions, the model becomes less of a 'black box.'
- For extracting helpful information out of real-world datasets, the ability to deal with sparse and noisy data is essential.
The project has validated the use of the deep learning method for real–world steels applications and provided us with insights that can help us to improve steel properties and focus valuable experimental resources more efficiently. We found the feature importance and sensitivity plots to be a very worthwhile guide."
Lode Duprez, Chief Scientific Officer, OCAS.
About OCAS and Intellegens
A research center of Finocas, OCAS NV is a joint venture between global steel leader ArcelorMittal and the Flemish region. Providing steel and metal-based services, products, and solutions to metal processing companies worldwide, OCAS co-develops steel applications, develops alloys and coatings, and produces and tests samples.
OCAS has over 150 researchers and engineers and is equipped with state-of-the-art facilities and R&D tools within its laboratories in Zelzate and Zwijnaarde (Belgium). Intellegens provides unique deep learning software, Alchemite™. The organization's focus is on simplifying the application of machine learning to accelerate innovation in chemicals, materials, manufacturing, and beyond.
When presented with real-world, sparse, noisy data, Alchemite™ can train machine learning models. Having originated at the University of Cambridge, method development is ongoing at Intellegens. The method has been deployed with success in industrial R&D and process improvements in additive manufacturing, superalloys, formulated products, chemical processes, drug discovery, and batteries.
References
- “Alchemite™ deep learning - solving complex problems with real-world data”, Intellegens White Paper (2021)

This information has been sourced, reviewed and adapted from materials provided by Intellegens Limited.
For more information on this source, please visit Intellegens Limited.