From EDAXDec 14 2022Reviewed by Olivia Frost
In this interview, AZoM speaks with Matt Nowell and Dr. Will Lenthe from EDAX about new tools for EBSD data collection and analysis.
Please could you introduce yourselves and your roles at EDAX?
Matt Nowell: I am Matt Nowell, and I am the EBSD product manager at EDAX. I have a passion for EBSD microstructural characterization and joined TexSEM Labs (TSL), upon graduation from the University of Utah in 1995 with a degree in material science and engineering.
Dr. Will Lenthe: My name is Dr. Will Lenthe, and I joined EDAX as the principal software engineer in 2020. I first garnered interest in electron microscopy as an undergraduate at Lehigh University before completing my Ph.D. at the University of California Santa Barbara in Tresa Pollock’s group.
My graduate work included the collection and quantitative analysis of multiple 3D EBSD data sets. As a postdoc in Mark De Graef’s group at Carnegie Mellon University, I soon developed a spherical approach to forward model-based EBSD indexing.
Could you provide an overview of the EBSD product line at EDAX and some of the new emerging technologies?
Matt Nowell: Some of the recent developments that are going to be available from our EBSD product line include the new Velocity™ Ultra high-speed CMOS detector and a new version of OIM Analysis™, which has updates to the OIM Matrix™ package and will now include spherical indexing capability.
The Velocity is our high-speed CMOS detector series, and it is a detector that is really good for routine characterization. Moreover, with the faster acquisition speed of the Ultra, this detector will become ideal for 3D and in-situ characterization - where time is of the essence. Essentially, it shortens the time to results. It can scale to the needs of a lab, and regardless of the camera, it will still produce high-quality, accurate orientation data on a wide range of materials.
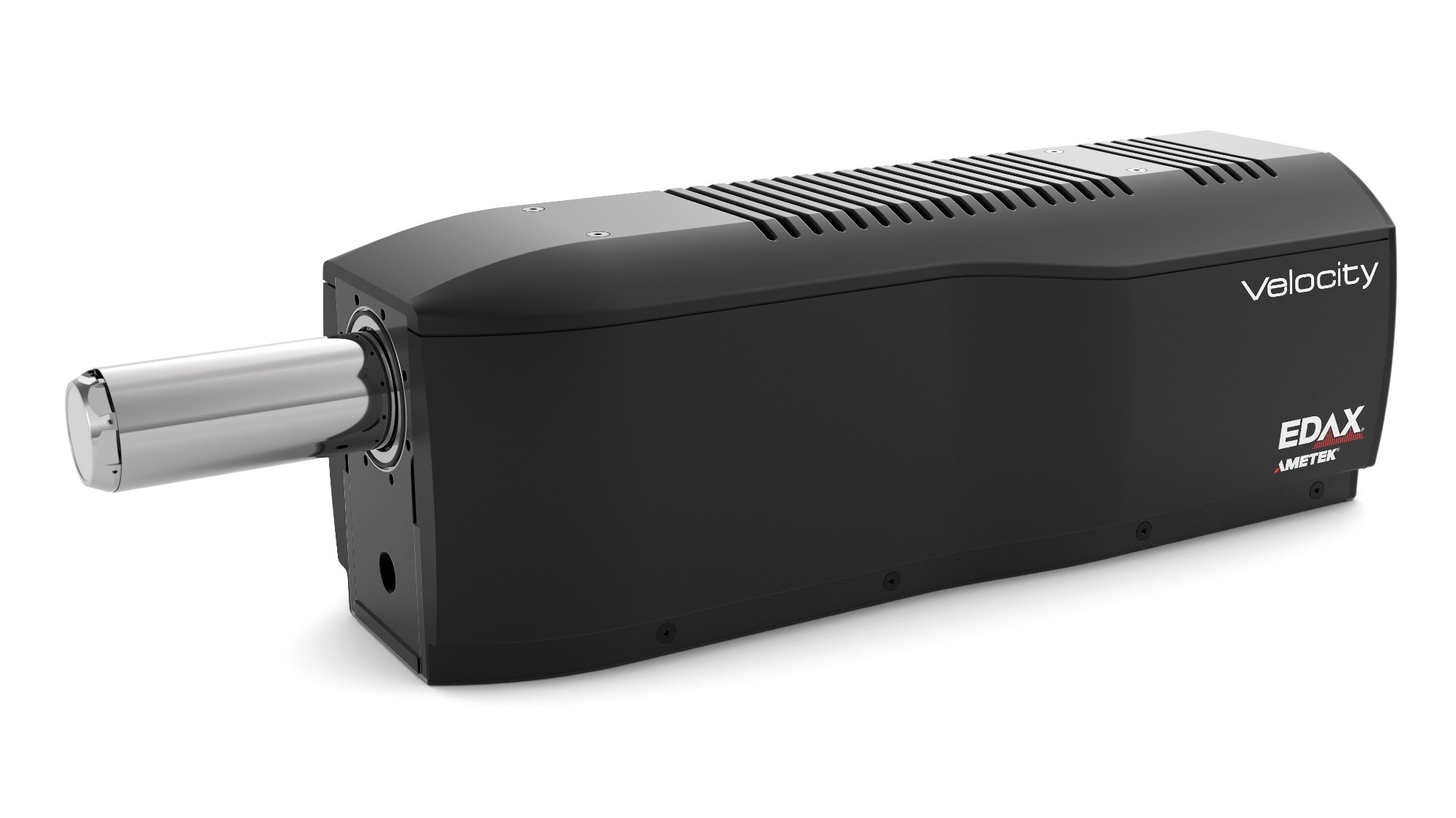
Velocity Ultra EBSD Camera.
Image Credit: EDAX
How does the new Velocity Ultra compare to earlier models in the same series?
Matt Nowell: This series was rolled out a few years back now, and this year we have introduced the Velocity Ultra, which is the fastest EBSD detector currently available. It provides live indexing speeds of up to 6,700 indexed points per second. This capability was only achievable through an optimized CMOS sensor - a low-noise, high-speed sensor tuned for EBSD signal and operation.
The Ultra has improved effective sensitivity via more efficient data transfer. We can get the data out of the sensor into the computer faster while still maintaining high-quality data. It is really important to emphasize that there is no compromise in terms of the data quality that the detector is capable of providing.
Across the range, we have the Pro, the Plus, the Super, and now the Ultra. Having the option to select from multiple models allows you to meet the throughput demand of a lab. There is also consistent quality across all the models. Depending on the client’s needs, there are speeds that will fit the application requirements. The Ultra provides the highest speeds for applications where acquisition speed is the critical goal.
What advantages does the Velocity Ultra have, and does it open up the potential for any new scanning strategies?
Matt Nowell: Thanks to the benefits provided by the Velocity Ultra, we can collect data from a range of materials at varying speeds. The device is not limited to nice equiaxed nickel grains but can also gather quick results from, for instance, a deformed aluminum sample. Even with this range of materials, we can still achieve the fastest speeds and get high-quality data from these different microstructures.
One of the biggest advantages we see with the Velocity Ultra, and with high-speed cameras in general, is we can now collect data faster.
We can also measure grain shape a little bit better and collect statistically significant data in just a few minutes. This means that users can measure the whole microstructure, texture, grain size, and misorientation in just a single scan, meaning that users can be more time-efficient when using an SEM. This completely changes things from when things were slower.
When and where is high-speed data acquisition useful?
Matt Nowell: High-speed data acquisition is extremely useful in a variety of scenarios. One example would be an in-situ experiment where things are happening dynamically, and we want to be able to capture information as quickly as possible.
For instance, let us consider recrystallization in a copper material. To characterize this, we start with the deformed material. As the material is heated, new recrystallized grains nucleating grow within the deformed material. The faster we can collect the EBSD, the larger the area, the finer the time slices, and the smaller the spatial resolution that we can analyze.
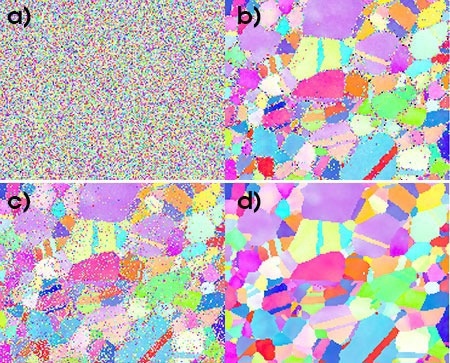
Caption: a) Raw pattern and b) NPAR pattern using Hough indexing and c) raw pattern and d) NPAR pattern using spherical indexing with a bandwidth of 127.
Image Credit: EDAX
Having this capability allows us to say how fast different grain boundaries migrate and which grain orientations grow. Faster detectors help to enable better in-situ experiments.
Interestingly, 3D analysis is another application where a high-speed Velocity Ultra will be particularly useful. With these cameras, we will be able to cover larger 3D areas while still maintaining good spatial resolution that complements the direction of modern FIB system developments, including plasma FIBs and laser ablation systems. Being able to collect data faster helps to facilitate that type of analysis.
Another interesting example of using large data sets is measuring retained Austenite. For instance, when checking a turbine blade, we are looking at a relatively large area with large orientation packets. However, we are also trying to look at the very fine elements with the different packets because the retained Austenite in this microstructure can be pretty small.
Therefore, we want to look at large areas but do this with a good spatial resolution. This requires about a 30 million point data set and would take about 80 minutes to collect.
How does the Velocity Ultra handle the different grain sizes of a material?
Matt Nowell: The power of the high-speed Ultra really comes into play when there is a wide range of grain sizes at hand, for example, a friction stir weld in an aluminum alloy. When it comes to measuring grain size, we need to measure multiple points per grain to get a decent representation of the grain size.
However, we have to select a step size based on the smaller grains that we are going to measure. For example, if we are looking at 700 microns by 500 microns with a 250-nanometer step size, that will give us about six million data points in about 15 minutes. This step size will allow confidence analysis of 1-micron grains and larger.
When looking at a grain map, grains are randomly colored to show the morphology, and we are able to see that there are smaller grains and larger grains, meaning we can then plot out the grain size distribution and assign a color to it.
From this, it is clear that when we have enough data points to resolve the smallest grains statistically and then take this data and break it down into small- and large-grained regions from this bimodal distribution microstructure. Therefore, we can analyze this full field of view in one pass and crop and partition out what we want. From this, we can understand or at least fully visualize the grain size differences and the microstructural transition across this welded zone.
Could you describe some of the high-speed camera and detectors’ benefits and how this impacts input and output?
Matt Nowell: We are expanding our capability to the widest range of materials possible. The speed of the Velocity detector and the Octane EDS detector allows us to get results in minutes rather than hours or days.
We also offer our Pegasus package with our Octane series EDS detectors; by combining these EDS-EBSD, we get accurate quantitative analysis of complex materials. They are both very high-speed, high-efficiency detectors.
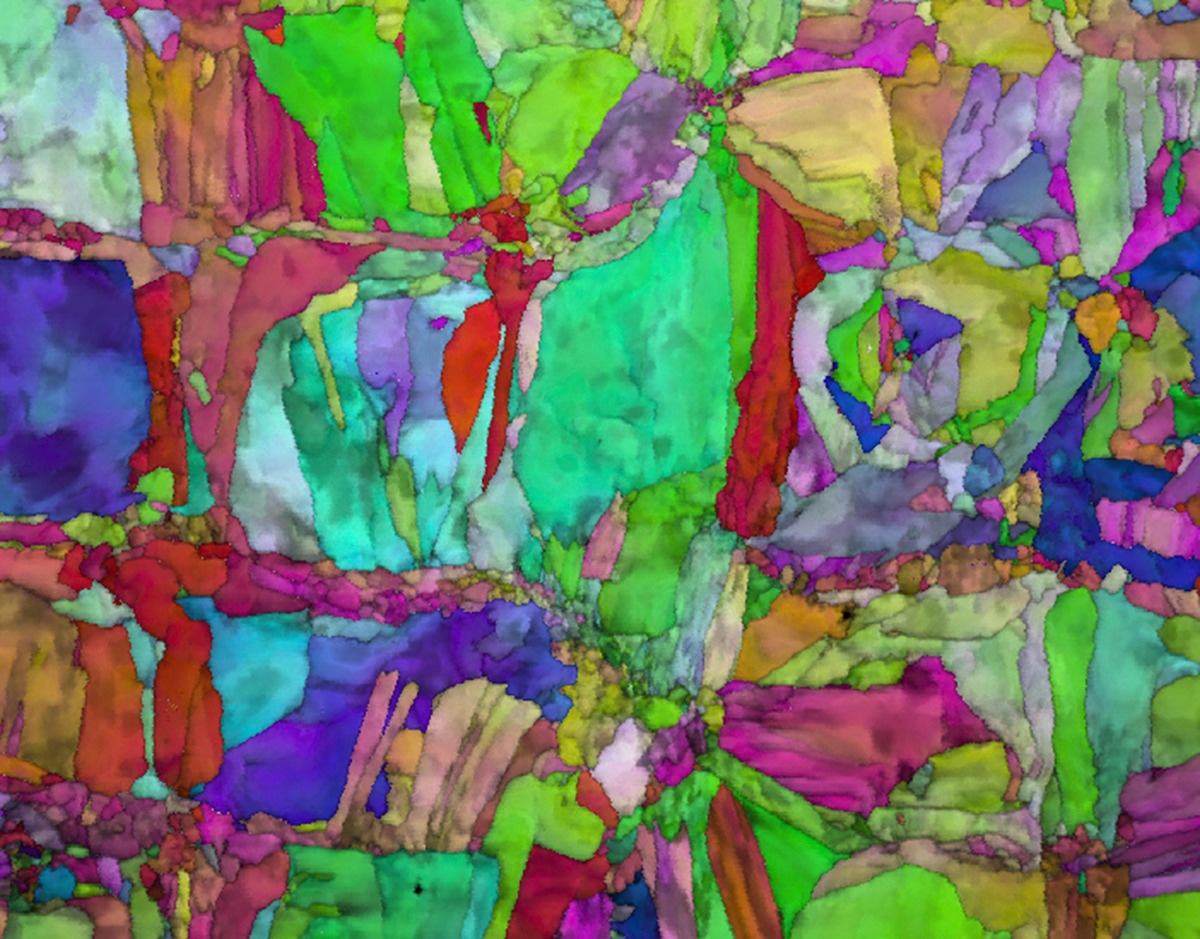
EBSD orientation map from additively manufactured 316L was collected at 6,700 ipps with Velocity Ultra with a 99% indexing success rate.
Image Credit: EDAX
With the Octane Elite detector, we can get better output count rates even at a high input count rate. We are about five times better than other detectors. This is important because as we go into the realm of higher speeds, we want to make sure we have enough counts in the detector and enough counts per pixel at these acquisition speeds.
In short, we want to be able to get lots of counts in to get lots of counts out efficiently.
How do you use the EDS information, and how is this useful when combined with the EBSD data?
Matt Nowell: We can use the EDS information to differentiate crystallographically similar materials. For example, aluminum and copper are both face-centered cubic materials. This means the band positioned within the EBSD patterns from these phases are the same. However, they are chemically distinct and easy to identify with EDS. Once we have that phase differentiation, we can identify the particular phases’ orientations, meaning we get the correct quantitative analysis of each phase by combining the EDS and EBSD data.
This enables unparalleled EBSD data quality. This grants us access to several tools in our system that allow us to achieve the best possible results. We also have a wide range of indexing tools to do that: we have our triplet indexing and our Hough transform-based indexing.
We also have NPAR, which improves the signal and noise through local spatial averaging, and we have ChI-scan, which is the integrated EDS-EBSD. We have our confidence index value that says how reliable is each measurement we make, and then we have our dynamic pattern simulation tools and our faster spherical indexing.
Could you provide an overview of OIM Analysis and how the new version enhances the system?
Matt Nowell: Collecting the crystallographic orientation data is the first part of EBSD, but we need to do something with that data to develop a better understanding of a material and its microstructure. We can use OIM Analysis to visualize a number of different types of microstructural features, and we can analyze things like orientation, misorientation, and texture and understand them through partitioning and reporting.
Within OIM Analysis, we also have our reindexing tools. This is where we can take the EBSD patterns that are initially collected on the microscope and analyze them further. We can find additional phases we can index better. And that will include things like pattern simulation and spherical indexing. Therefore, what we have done is develop our next version of OIM analysis - OIM Analysis v9 - and this is the version that will be available with Velocity Ultra.
This version offers 30 times improvement in file opening, 20 times improvement in drawing the maps, six times improvement in grain calculations, and it has been optimized for the larger data sets that can be collected using the Velocity system.
We have also improved our OIM Matrix module. The OIM matrix is our pattern indexing based on the forward modeling approach.
How does the improved OIM Matrix module enhance pattern indexing and/or forward model-based indexing?
Dr. Will Lenthe: To answer this question, I first need to address what forward model-based indexing is. Essentially, forward model-based indexing involves solving a forward problem instead of an inverse problem, hence its name.
All that forward model-based indexing means is that instead of trying to do that backwards problem of deducing a material property from an experimental measurement, we do the forward problem of simulating the experiment for a material property. The reason that this is important is that inverse problems are often mathematically ill-posed, which means that they have a lot of pitfalls. By doing a forward problem, we avoid that issue.
With the forward model approach, the idea is that we describe the physics of the experiment that we are doing, which can involve an arbitrary number of factors, but typically you have a beam that is used to probe the sample and is modulated by the interaction. The exit signal is then captured with a detector. Normally, you want to start with the simplest model you can and add complexity until the fidelity is sufficient for whatever you are doing.
As you can imagine, this may become pretty challenging for some experiments, meaning that often these approaches are significantly more computationally intensive than inverse approaches, which is why inverse approaches are widely used. Despite being a bit of extra work, the forward model delivers a much better indexing result.
How can the benefits of forward modeling be applied to Electron Backscatter Diffraction (EBSD)?
Dr. Will Lenthe: For EBSD, we use the EMsoft package, which splits the forward modeling process of taking a sample and simulating the resulting EBSD pattern into three parts to make it computationally tractable; the backscatter electron yield, a dynamical diffraction simulation, which is the most expensive part, and then using the experimental geometry and orientation to simulate an individual pattern.1
The Monte Carlo simulation is a standard backscatter Monte Carlo approach. The main difference is that instead of just keeping the energy distribution, which is what is normally of interest, a 4D histogram to know how many electrons exit the sample in each direction and from what scattering depth is required. The model assumes a monochromatic source, a perfect zero-width incident beam, and a semi-infinite sample.
The model counts all of the electrons that come out of the sample, tracking the final scattering even before exiting and the direction that each left the sample. Once the Monte Carlo simulation is complete, a dynamical simulation is run for discrete energy bins. Starting from the depth provided by the Monte Carlo simulation, a block wave approach is used to get the diffracted intensity for the entire sphere.
Finally, to simulate the patterns, you basically just loop over all of the different simulations that you have already. Essentially you end up using the geometry of your detector and the rotation of the crystal to position your detector on the full sphere of diffracted intensity and then crop down the detector shape.
Once you’re able to simulate a pattern, how is that used to actually index?
Dr. Will Lenthe: The first approach was dictionary indexing, which is a brute-force solution. The idea is to simulate a pattern for every possible orientation, creating a so-called dictionary of patterns. Then to index an experimental pattern, you just compare it against the entire dictionary and determine which one is the best match. The orientation used to simulate the best matching pattern is the indexing result.
The challenge here is that you need impractically large dictionaries to get a sufficiently high resolution. Therefore, we have to use a courser dictionary and then refine the orientation between those grid points by using a nonlinear optimization algorithm.
What benefits does dictionary indexing offer?
Dr. Will Lenthe: Dictionary indexing gives you robustness, but as you can imagine, it is much more expensive because you need to simulate all of the orientation space and then compare against every pattern separately.
Then you have this trade-off between increasing your dictionary resolution, which means that you have a finer space to search within when you are carrying out the refinement step, which means spending less time on the refinement.
You end up with a classic trade-off where you cannot really improve the time it takes, no matter what you do. There is only a certain space that is viable because, at some point, your dictionary gets too large to be tractable on one side, and on the other side, it becomes so sparse that you do not actually find a good initial orientation.
Is there a faster forward-model-based indexing technique?
Dr. Will Lenthe: Spherical harmonic-based EBSD indexing starts with the same forward model as dictionary indexing but uses a different comparison approach. Instead of simulating a 2D EBSD pattern for every orientation, the experimental geometry is used to project the collected pattern back onto the sphere. The indexing problem is then reduced to finding the rotation of the resulting spherical image that best aligns with the spherical image produced by the forward model.
To find the best rotation, we need to measure the correlation for every possible orientation, just like in dictionary indexing. The difference is that for a pair of spherical images, the similarity can be simultaneously computed for every possible rotation efficiently using spherical harmonic transforms. Conceptually this is analogous to finding the shift that best aligns two 2D images using an FFT-based cross-correlation. The spherical harmonic transform is the equivalent of a Fourier transform on the sphere and has an analog to the convolution theorem. The math is more challenging since the basis functions are more complicated than the Sin and Cos used for Fourier transforms, but there is a significant speed improvement compared to dictionary indexing.2
What are the main differences between the various indexing techniques?
Dr. Will Lenthe: Dictionary indexing and spherical indexing are built from the same forward model, so they have similar advantages compared to inverse approaches like Hough transform-based indexing. Compared to Hough indexing, both techniques provide improved phase discrimination, noise tolerance, and angular precision. The main disadvantage for both is that the initial simulation is relatively expensive. Fortunately, it only needs to be computed once for a given material, and accelerating voltage and the Matrix module comes with simulations for every material in our database.
The biggest advantage of spherical indexing over dictionary indexing is the significant speed improvement, with thousands of patterns per second easily indexed on modest computer hardware. Another more subtle advantage is that the dictionary of patterns generated for dictionary indexing uses a single pattern center (experimental geometry) for every pattern. Since spherical indexing uses the geometry during back projection, a unique pattern center can be used for every point in the scan. That means you can achieve high-quality results over large scans that have a pattern center shift without having to do a subsequent refinement step.
Finally, since spherical indexing only has a single user parameter, there is a really shallow learning curve compared to both Hough and Dictionary indexing. The bandwidth parameter (no relation to the width of a band in an EBSD pattern) describes how far out in frequency space to go for the spherical harmonic transform. Increasing bandwidth provides higher fidelity but is more computationally expensive so indexing takes longer.
With all three approaches integrated into OIM Analysis, it is easy to try them all on your own datasets. The ability to combine advanced image processing approaches like NPAR with fast spherical indexing can give excellent results on some very challenging samples.
About Matt Nowell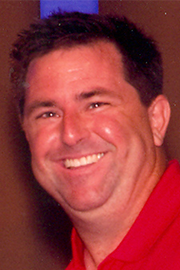
Matt Nowell is the EBSD Product Manager at EDAX and has a passion for EBSD and microstructural characterization. Matt joined TexSEM Labs (TSL) upon graduation from the University of Utah in 1995 with a degree in Materials Science and Engineering. At TSL, he was part of the team that pioneered the development and commercialization of EBSD and OIM. After EDAX acquired TSL in 1999, he joined the applications group to help continue to develop EBSD as a technique, and integrate structural information with chemical information collected using EDS.
Within EDAX, Matt has held several roles, including product management, business development, customer and technical support, engineering, and applications support and development. Matt has published over 70 papers in a variety of application areas. He greatly enjoys the opportunity to interact with scientists, engineers, and microscopists to help expand the role that EBSD plays in materials characterization. In his spare time, Matt enjoys playing golf and pondering if changing the texture of his clubs will affect his final score.
About Will Lenthe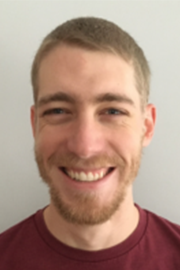
Will Lenthe joined EDAX as the Principal Software Engineer in 2020. He took an interest in electron microscopy as an undergraduate at Lehigh University before completing a Ph.D. at the University of California Santa Barbara (UCSB) in Tresa Pollock’s group.
His graduate work included the collection and quantitative analysis of multiple 3D EBSD datasets. As a postdoc in Marc De Graef’s group, he developed a spherical approach to forward model-based EBSD indexing.
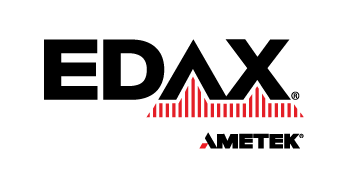
References
1. Callahan, P. G., & De Graef, M. (2013). Dynamical electron backscatter diffraction patterns. Part I: Pattern simulations. Microscopy and Microanalysis, 19(5), 1255-1265.
2. Lenthe, W. C., Singh, S., & De Graef, M. (2019). A spherical harmonic transform approach to the indexing of electron back-scattered diffraction patterns. Ultramicroscopy, 207, 112841.
This information has been sourced, reviewed, and adapted from materials provided by EDAX.
For more information on this source, please visit EDAX.
Disclaimer: The views expressed here are those of the interviewee and do not necessarily represent the views of AZoM.com Limited (T/A) AZoNetwork, the owner and operator of this website. This disclaimer forms part of the Terms and Conditions of use of this website.