Researchers have devised a data-centric approach to generative modeling for 3D-printed steel. The team believes that their model could help determine the quality of a design and the material before fabrication. The research is published in the Proceedings of the Royal Society A.
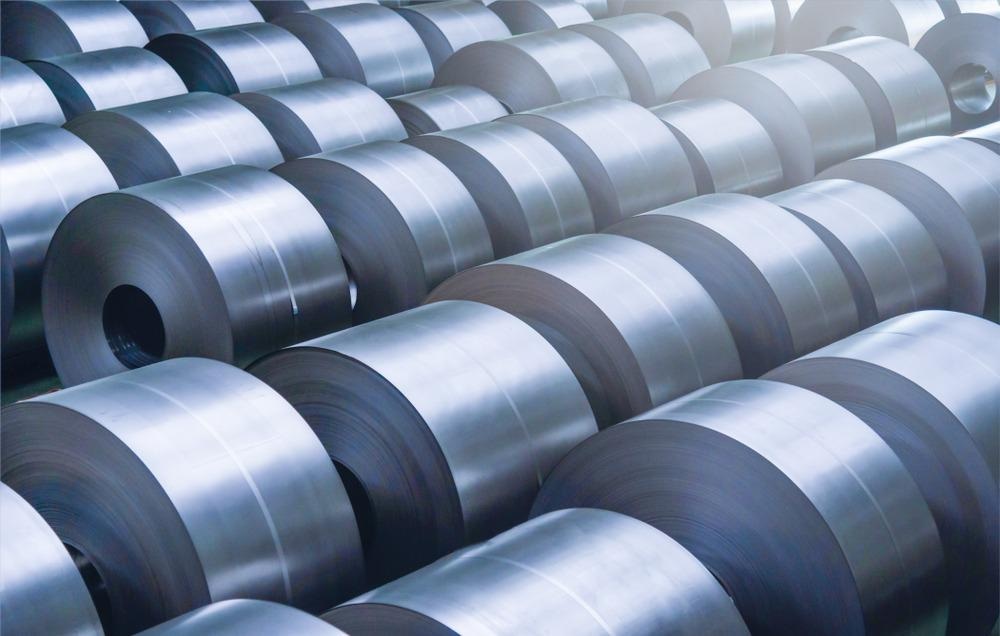
Study: A data-centric approach to generative modelling for 3D-printed steelProc. Image Credit: Nuttawut Uttamaharad/Shutterstock.com
Additive manufacturing (AM) processes, commonly known as 3D printing, present a multitude of exciting opportunities across numerous industries including engineering, aerospace, and automotive. This is due to the development of AM for metallic materials which has facilitated the production of complex and intricate components such as fuel nozzles for jet engines.
However, unlike conventional materials, metals that have been fabricated and formed via AM processes exhibit greater levels of variation in both their geometric and mechanical properties.
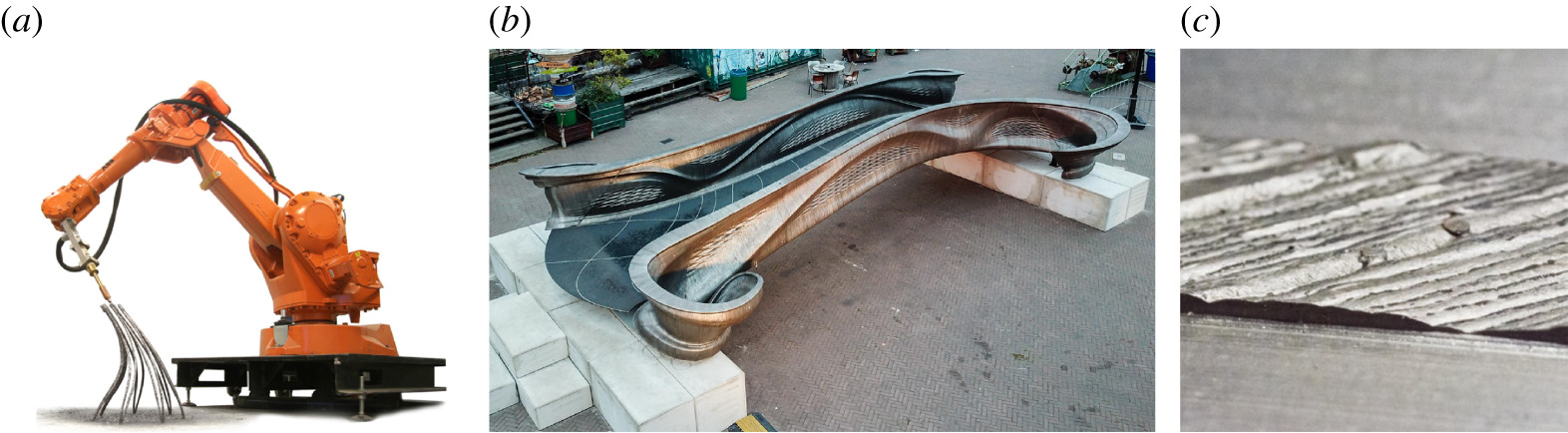
(a) The three-dimensional printing protocol developed by MX3D uses a weld head attached to a robotic arm (image by Joris Laarman, www.jorislaarman.com). (b) Pedestrian bridge manufactured using three-dimensional-printed steel. (c) Close-up of the geometric variation on the surface of the material. Image Credit: Dodwell T. J., et al., Proceedings of the Royal Society A
These variations are not widely understood which impedes post-manufacture testing for establishing safety standards which mean manufacturers are met with a certain barrier.
Steel WAAM Components
Of all the emerging technologies in AM, one of the most promising for the production of large-scale components is wire arc additive manufacturing (WAAM). This process is a variation of direct energy deposition (DED) technology and utilizes an arc welding process to 3D-print metal components.
Unlike conventional metal powder AM methods, WAAM melts metal wire using an electric arc as a heat source on a metal substrate base. When the wire is melted and expressed in the form of beads on the substrate. As the beads adhere to one another, a layer of metal material is produced. The process is then repeated, layer-by-layer until the metal component is completed.
However, there are still challenges associated with this process owing to the uncertainty around the structural and mechanical properties and complex thermal deformations. Hence the need for a method to support an effective material characterization for WAAM: “Specifically, we develop a generative statistical model that enables ensemble-based predictions of the performance of a stainless steel WAAM component before it is manufactured,” explained Dodwell.
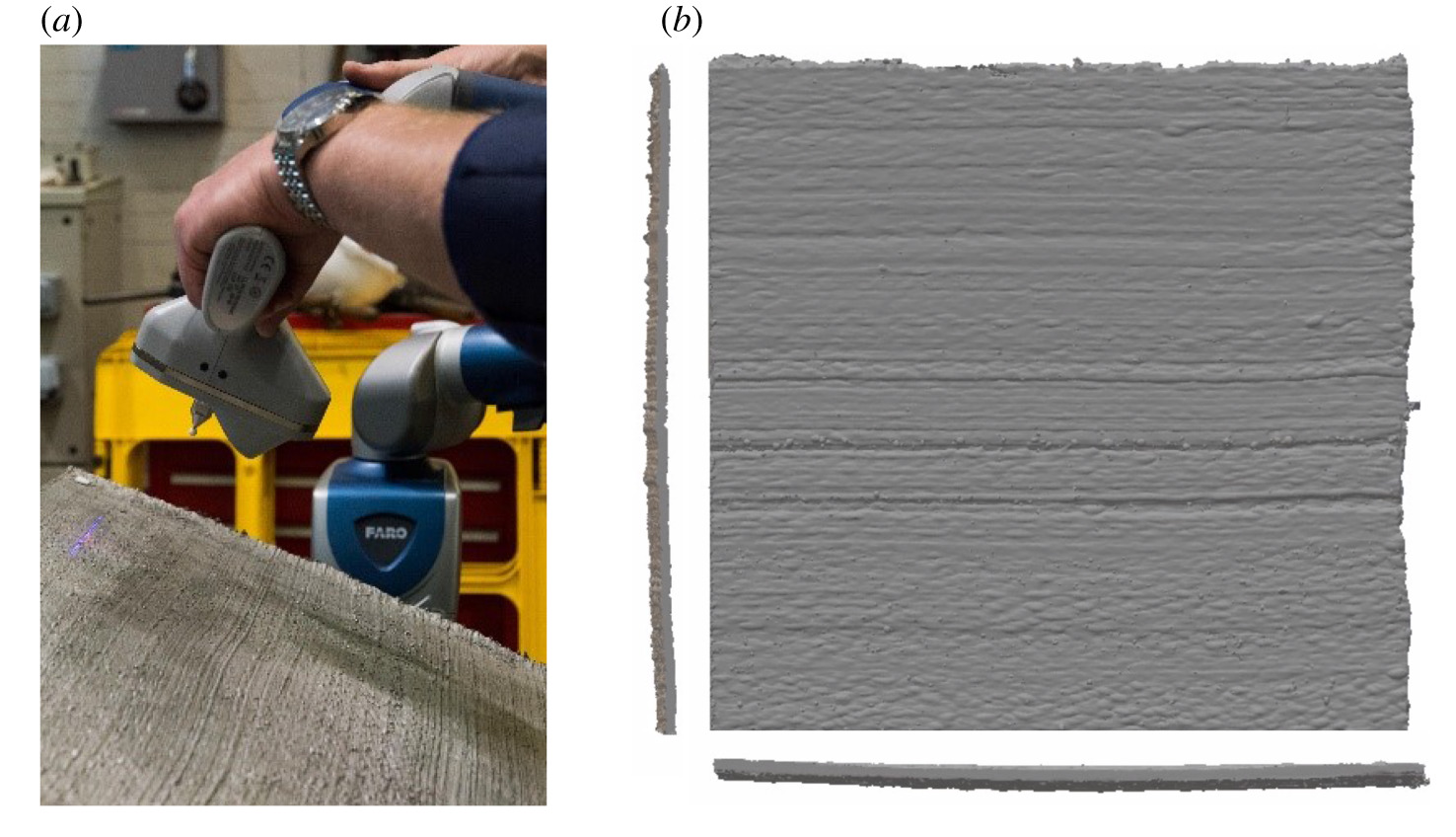
Laser scan of a three-dimensional-printed steel sheet. (a) Photograph of the hand-held scanning equipment. (b) Orthographic projection of (a portion of) the scanned sheet, denoted a panel. The notional thickness of the panel is 3.5 mm. (Observe a slight curvature in the notionally flat-panel introduced by the residual stress). Image Credit: Dodwell T. J., et al., Proceedings of the Royal Society A
Statistical Modelling
In order to successfully characterize the mechanical properties of WAAM steel effectively, the team also needed to develop a method for isolating geometric variation characterization. Then by combining the generative statistical models for mechanical and geometric variation in WAAM steel, a unified statistical model was produced. What is more, this generative statistical model treats both sources of variation independently.
“The statistical models described above are combined into a unified statistical model whose instantiations can be realized in the finite-element software ABAQUS, in order that the nominal design of a CHS can be endowed with realistic surface geometry and material properties according to this unified statistical model,” says Dodwell.
The team demonstrated that acquiring relatively small amounts of training data can be utilized for successfully training a generative statistical model for AM steel. “Approximate predictions for the performance of a hypothetical component are a prerequisite for an efficient and low-cost design using WAAM,” says Dodwell.
This paves the way for making general predictions of performance at a variety of structural lengths, however, the team state that experimental testing remains imperative where safety-critical certifications are concerned.
However, showing the capacity to produce a generative statistical model for AM steel and WAAM can enhance future research potential which uses stochastic simulations to determine key performance aspects of AM metallic materials.
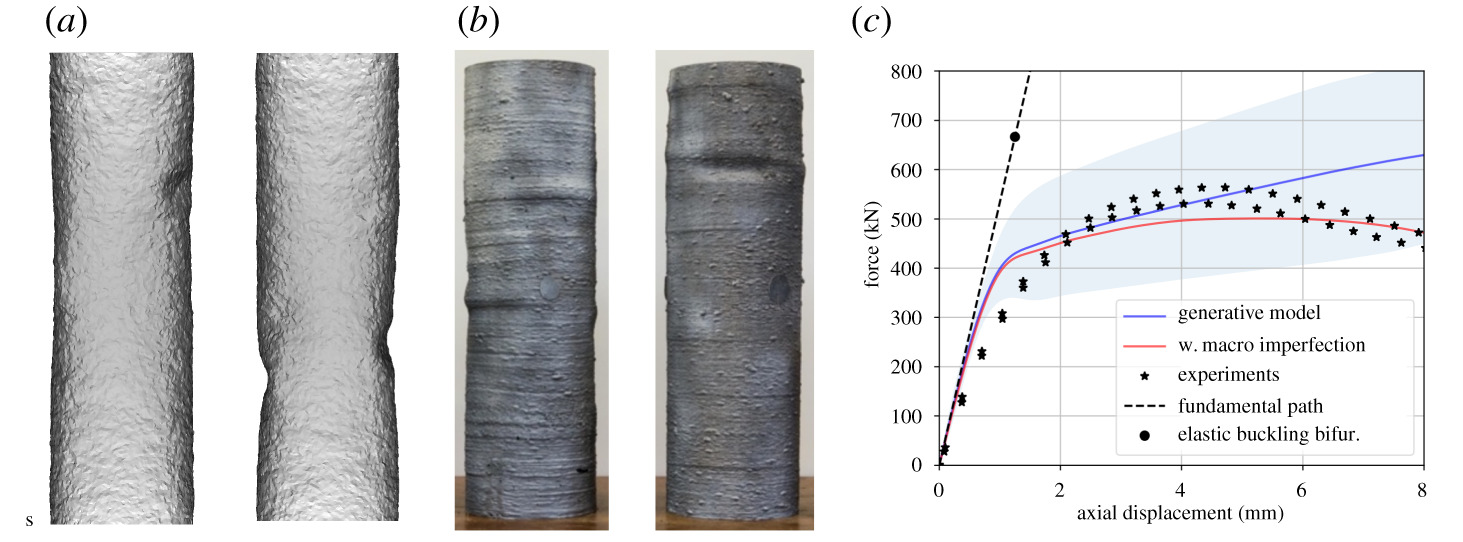
(a) Samples from the trained generative model. (b) Buckled cylinders, experimentally produced. (c) Predicted and experimental load-displacement curves based on the generative model, with and without macro-scale geometric imperfections introduced, and a perfect elastic model. Two s.d. regions are shaded. Image Credit: Dodwell T. J., et al., Proceedings of the Royal Society A
Dodwell and his team believe that their model will be well-suited to the task, “enabling the quantification of (and distinguishing between) both epistemic uncertainty, due to the limited training data, and aleatoric uncertainty, due to variation inherent to the printing protocol.”
Stochastic simulations are important in the AM process as it helps identify areas that should be targeted in relation to structural and mechanical integrity. These simulations are set to play a crucial role in improving printing protocols and reducing the cost of safety certification.
One key distinction between previous work on AM and the present work Dodwell and his research team conducted is that their model is both generative and independent of any particular design. This means that limited training data on just one type of component could ample enough to facilitate predictions for the performance of another, as yet unseen component.
References:
Dodwell T. J., Fleming L. R., Buchanan C., Kyvelou P., Detommaso G., Gosling P. D., Scheichl R., Kendall W. S., Gardner L., Girolami M. A. and Oates C. J. 2021 A data-centric approach to generative modelling for 3D-printed steelProc. R. Soc. A.4772021044420210444 https://royalsocietypublishing.org/doi/10.1098/rspa.2021.0444
Disclaimer: The views expressed here are those of the author expressed in their private capacity and do not necessarily represent the views of AZoM.com Limited T/A AZoNetwork the owner and operator of this website. This disclaimer forms part of the Terms and conditions of use of this website.