Life Cycle Assessments have become an important part of the evaluation of new materials and projects in recent years. Writing in the journal Sustainability, researchers from France and the US have investigated the role of artificial intelligence in conducting material Life Cycle Assessments.
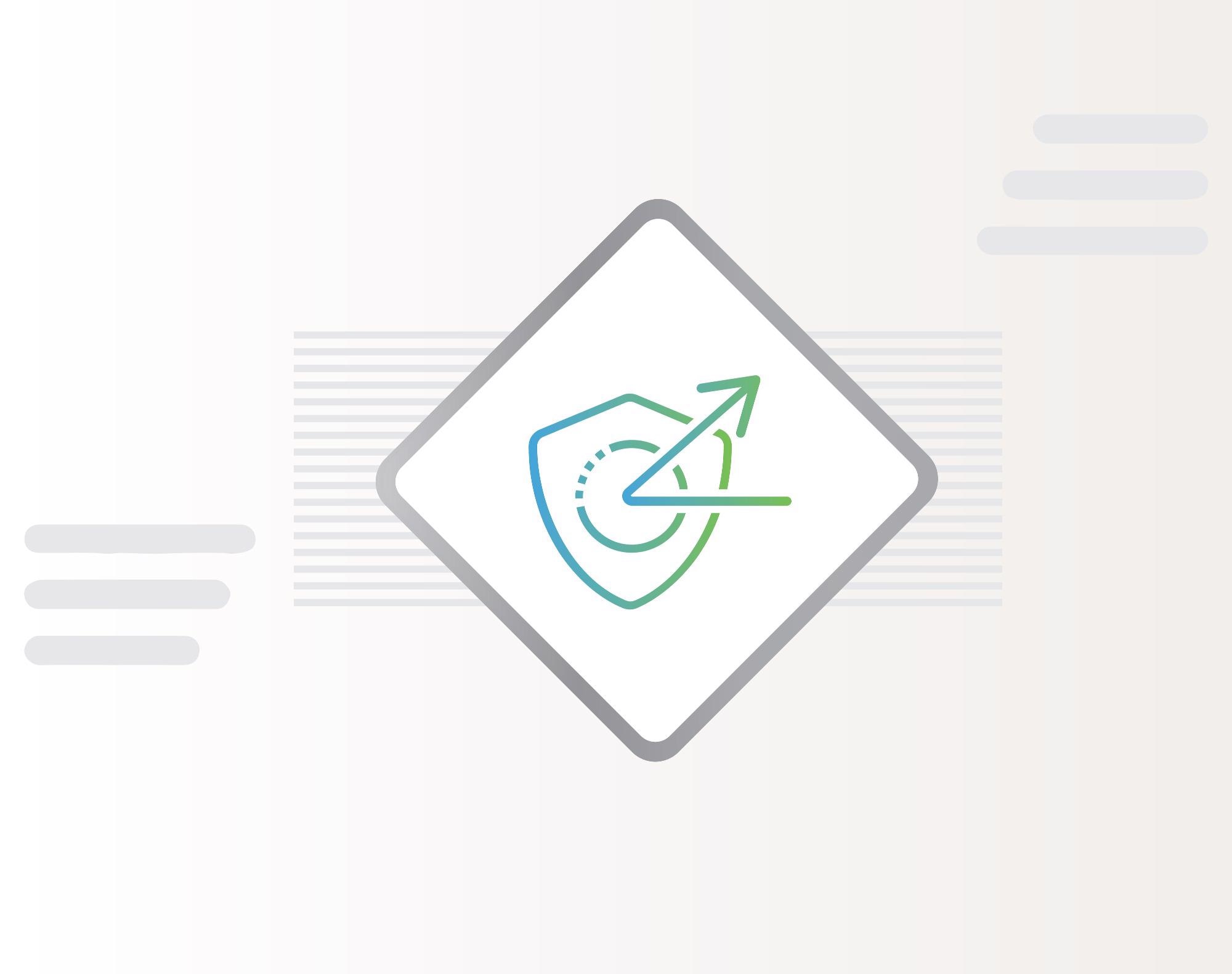
Study: Implementing Artificial Intelligence Techniques to Predict Environmental Impacts: Case of Construction Products. Image Credit: Diyajyoti/Shutterstock.com
Life Cycle Assessments in the Construction Industry
Construction is one of the main industries in modern society. Buildings account for approximately forty percent of the primary energy consumption and thirty-six percent of greenhouse gas emissions in Europe, significantly contributing to anthropogenic climate change. Due to the urgent need to address human impact on the environment and achieve net-zero carbon emissions, there is a pressing urgency for reducing the contribution of the construction sector.
There is a deepening understanding of the need for energy efficiency in the construction industry, but there is a poorer understanding of the impacts of building materials themselves. Amongst the strategies developed recently to tackle this issue, Life Cycle Assessments are a powerful analytical tool.
Life Cycle Assessments evaluate the environmental impact of a material or product over its entire lifespan, from the exploitation and processing of raw materials to the generation of waste and eventual disposal of materials. Life Cycle Assessments are time-intensive and require meticulous analysis and robust, systematic investigation to understand the total impact of a product, process, or service.
Many agencies and bodies have developed frameworks for developing Life Cycle Assessments in recent years. For instance, the European Union has developed Level(s), a framework to integrate Life Cycle Assessments into sustainability initiatives as part of its transition to carbon neutrality and a green economy. Whilst not a certification scheme, Environmental Product Declarations can be used to communicate the results of Life Cycle Assessments to stakeholders and customers.
A flow diagram representation of the ensemble method. Image Credit: Koyamparambath, A et al., Sustainability
Artificial Intelligence
There is a growing need to estimate the environmental impact of products and materials in the construction industry with a high degree of accuracy. Artificial intelligence has developed rapidly in recent years, finding applications in numerous industries, and can play a key role in improving the accuracy of Life Cycle Assessments.
Artificial intelligence is a wide-ranging discipline that envelopes everything that makes a machine “intelligent.” A subset of artificial intelligence is machine learning. Machine learning refers to statistical and mathematical algorithms that “learn” from previous experience in the form of datasets to improve future performance. Machine learning has numerous real-world applications.
Various methods have been utilized in recent years to leverage the power of artificial intelligence for Life Cycle Assessments. These include the agent-based model, a model based on game theory. Researchers have modeled the stakeholders in Life Cycle Assessments such as individuals, groups, and corporations. Models aim to maximize the payoff of each strategy permutation. Agency-based models and Life Cycle Assessments have been used together on multiple occasions by different researchers. Recent reviews have highlighted the growing use of AI and machine learning in Life Cycle Assessments.
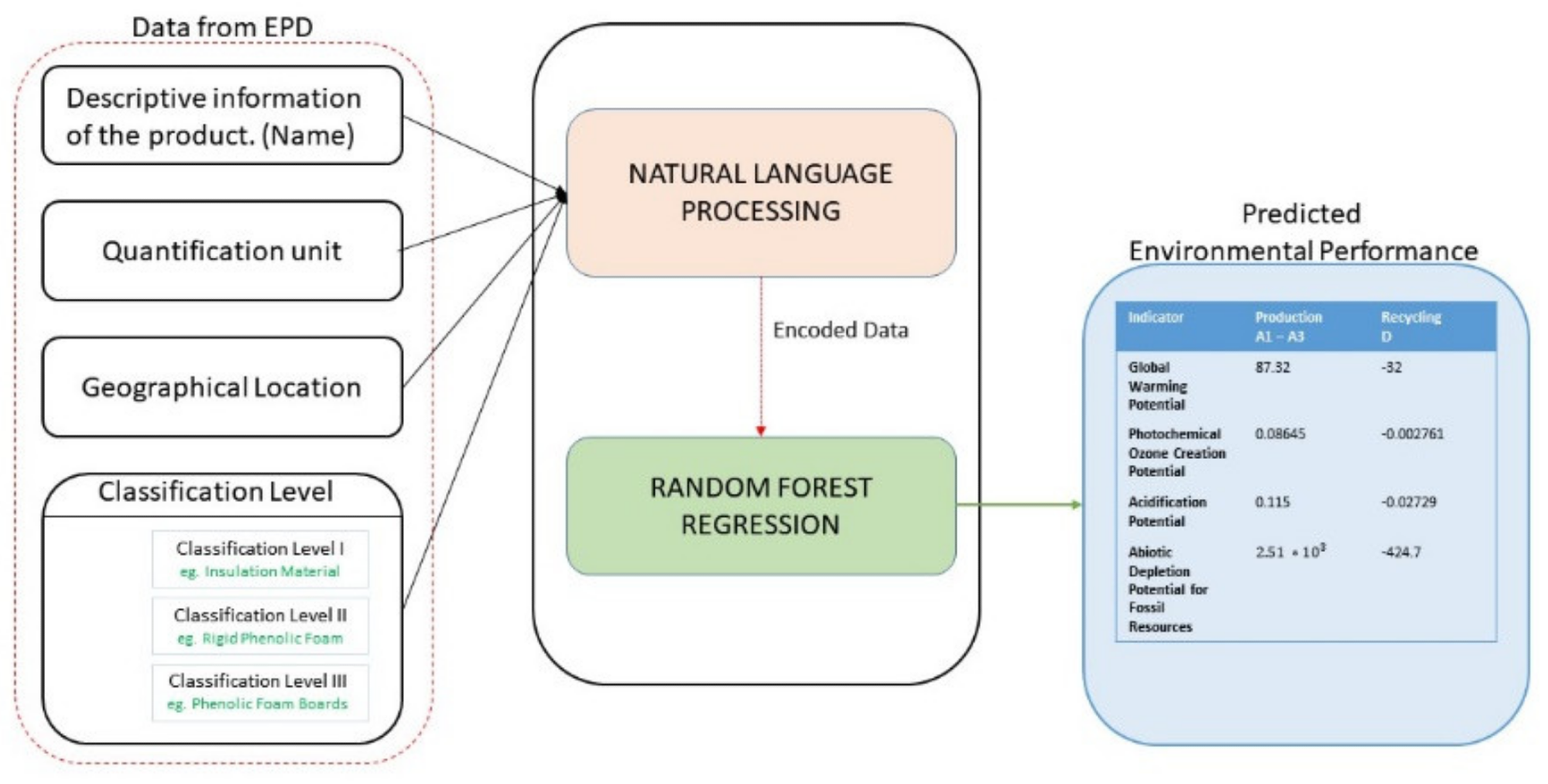
Inputs and outputs of the Machine Learning model. Image Credit: Koyamparambath, A et al., Sustainability
The Paper
The paper published in the journal Sustainability has aimed to explain and highlight a novel method for predicting a product or service’s environmental impact by learning from Environmental Product Declarations of products in the construction industry. Machine learning techniques used in the research were artificial neural networks random forest, and natural language processing.
The authors have stated that an artificial intelligence-based instant prediction model which requires minimal service or product data is missing from current research and most studies correspond to using artificial intelligence in the scope of Life Cycle Assessments. Current studies use inputs that are uncommon and unique and the methods developed in the research require additional data gathering.
The novel method developed by the authors is the first to attempt to estimate the values of four critical impact categories. These are the global warming potential, acidification potential, photochemical ozone creation potential, and abiotic depletion of fossil resources. The authors selected these impact categories for their different levels of robustness according to the EU’s Environmental Footprint database 3.0, part of the Single Market for Green Products Initiative.
The method presented in the research is not intended to replace a detailed Life Cycle Assessment study but is intended as a check for quickly predicting a product or service’s environmental impact. The novel trained model developed by the authors can predict the four impact categories with an R2 value of over 65%. Moreover, the authors demonstrated the model’s ability to use regression analysis to accurately predict environmental impact categories.
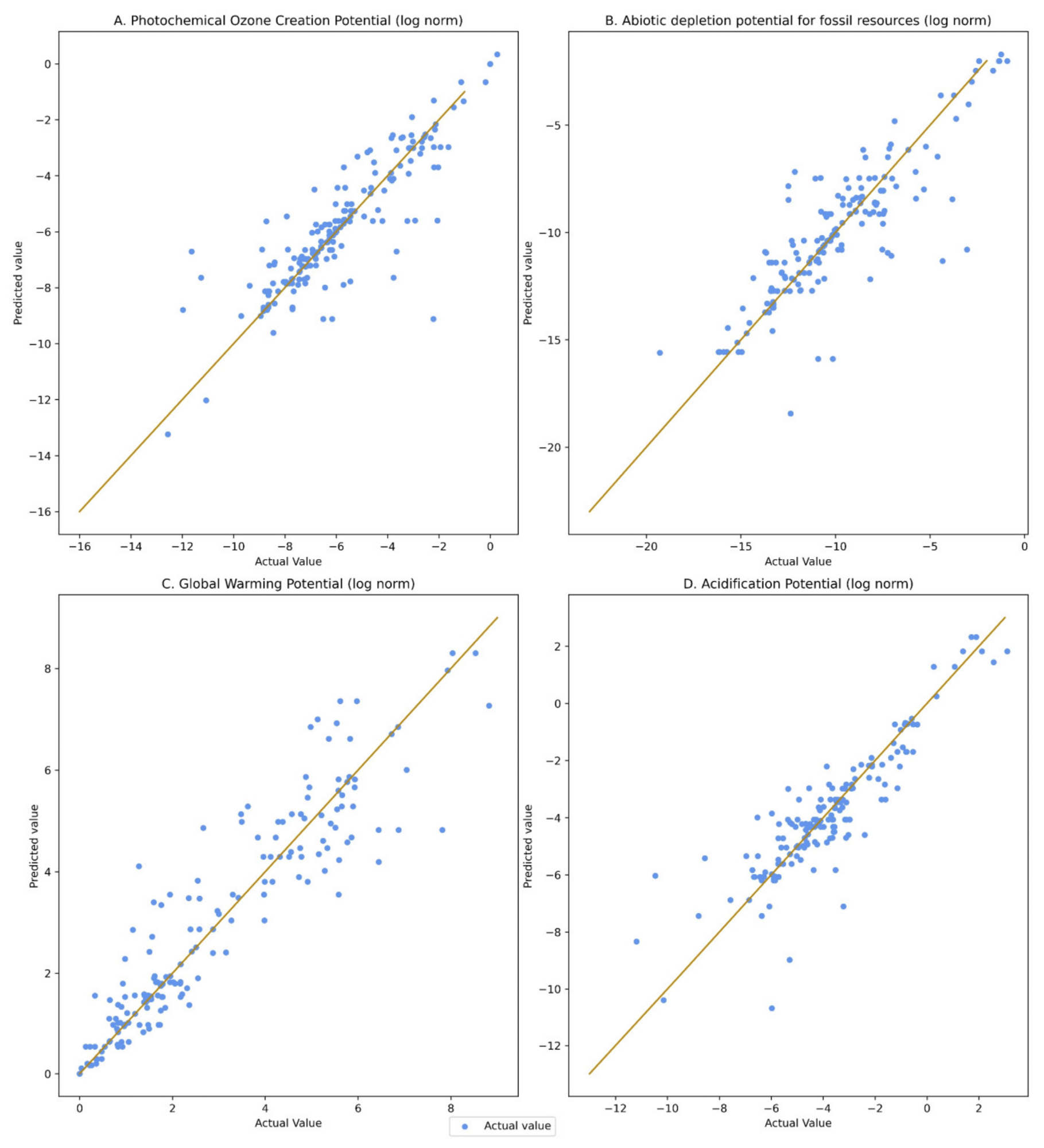
Visualization of prediction plot for the entire dataset for all the impact categories: (A) Photochemical Ozone Creation Potential, (B) Abiotic Depletion for Fossil Resources, (C) Global Warming Potential, (D) Acidification Potential. Image Credit: Koyamparambath, A et al., Sustainability
Future research perspectives stated by the authors include benchmarking the model’s performance with different machine learning methods and implementing the method to analyze other product groups and non-aggregated Life Cycle Assessment dataset results.
They have noted that the accuracy of results will be helped by the availability of more Environmental Product Declarations. For this reason, combining multiple databases for construction projects at the international level will be an exciting way forward for their research.
Further Reading
Koyamparambath, A et al. (2022) Implementing Artificial Intelligence Techniques to Predict Environmental Impacts: Case of Construction Products [online] Sustainability 14(6) 3699 | mdpi.com. Available at: https://www.mdpi.com/2071-1050/14/6/3699
Disclaimer: The views expressed here are those of the author expressed in their private capacity and do not necessarily represent the views of AZoM.com Limited T/A AZoNetwork the owner and operator of this website. This disclaimer forms part of the Terms and conditions of use of this website.