By Surbhi JainReviewed by Susha Cheriyedath, M.Sc.Apr 5 2022
In an article recently published in the Journal of Manufacturing Systems, researchers discussed the case study of a battery module assembly system for electric vehicles using an end-to-end big data analytics platform for Internet-of-Things (IoT)-enabled smart factories.
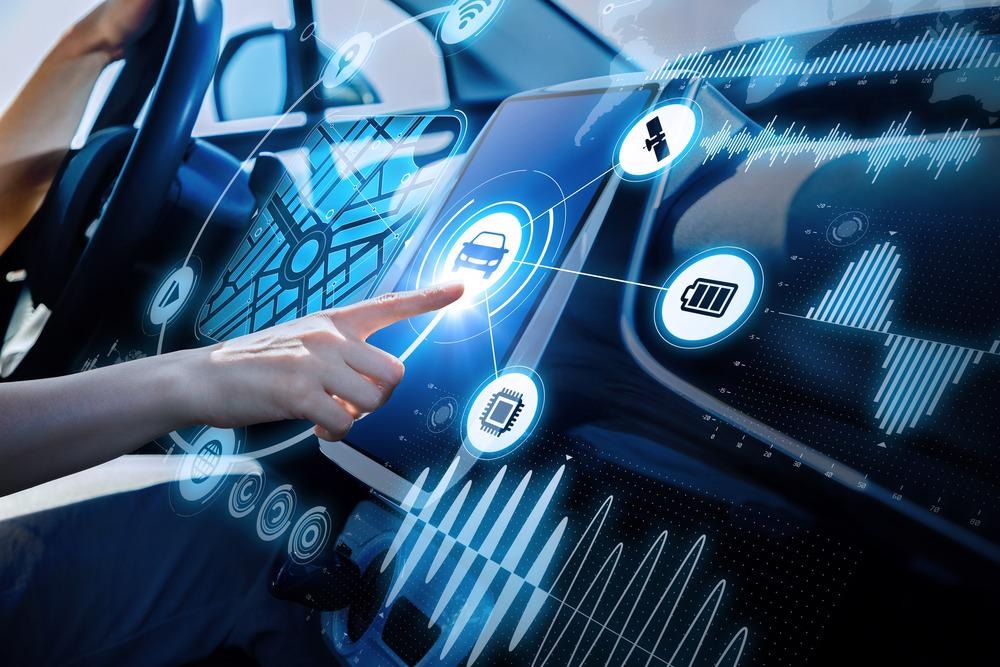
Study: An end-to-end big data analytics platform for IoT-enabled smart factories: A case study of battery module assembly system for electric vehicles. Image Credit: metamorworks/Shutterstock.com
Background
The necessity to turn existing production systems into self-learning and self-aware systems in order to add control and capacity to foresee failures, maintenance planning, and modify systems to unanticipated changes and new requirements gave rise to the notion of Industry 4.0.
The evolution of smart factories follows a number of key design concepts as part of the industry 4.0 paradigm. Big data analytics is critical in smart factories because it improves productivity, process safety, and product quality, as well as the economic and environmental resilience of manufacturing systems.
IoT enables the deployment of smart sensors that capture real-time production data, cloud computing enables networked data collection, off-site analytics, and data handling, and artificial intelligence (AI)/ machine learning (ML) enables decision-making flexibility and supports autonomous decisions in big data analytics systems. Despite its importance, the research has paid little attention to the practical applications of IoT-enabled industrial large data analytics systems.
High-level architecture of the proposed IoT-based end-to-end big data analytics platform. Image Credit: Kahveci, S et al., Shutterstock.com
About the Study
In this study, the authors described an end-to-end IoT-based big data analytics platform, which included five interconnected levels and a number of components for data gathering, storage, analytics, integration, and visualization. The platform architecture utilized the advantage of cutting-edge technologies and integrated them in a compatible and logical manner with clear data flows.
The team used the created platform in an electric car battery module assembly automation system developed by the University of Warwick's Automation Systems Group. The built proof-of-concept solution demonstrated how a wide range of tools and methodologies may be coordinated to work together in smart manufacturing environments to enhance decision-making and improve both process and product quality.
The researchers proposed and showed a five-layer, multi-component IoT-enabled big data analytics platform. The proposed solution allowed for an integrated system that facilitated decision-making at multiple levels of the management process.
A strong emphasis was made on using widely accessible industry standards for open-source technologies and interoperability to design and construct cost-effective enterprise systems for industrial applications. It covered strategies for analytics of streaming machine data and multi-tier processing in order to provide real-time actionable insights. The present options for big data analytics in smart industrial settings were reviewed. The architecture of an end-to-end IoT-enabled large data analytics platform was presented.
The authors also showed how the proposed reference design was implemented on an electric vehicle battery-operated module assembly automation system. The difficulties encountered throughout the platform's development and deployment were examined, as well as prospective alternatives were discussed.
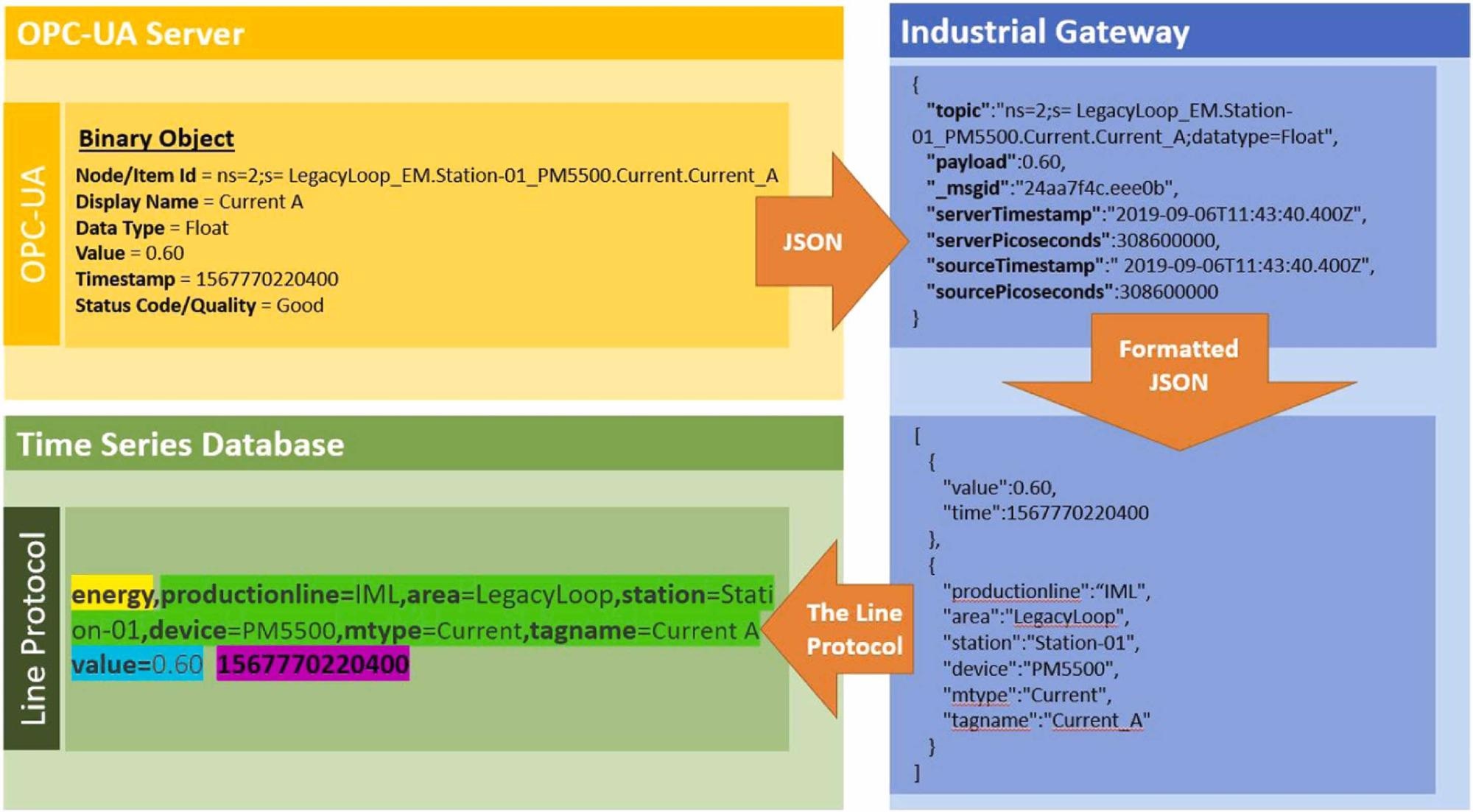
Representation of data transformation in the data integration layer. Image Credit: Kahveci, S et al., Shutterstock.com
Observations
Using open-source web-based data visualization technologies to build the presentation layer cut development time in half. The solution effectively supplied multi-platform creative user experiences for different jobs with different levels of information by fully utilizing interactive and responsive web user interfaces.
A reference design for a big-data analytics platform was demonstrated which was interoperable, resilient, end-to-end secure, and scalable. It was observed that utilizing a well-defined multi-tier platform aided in the cost-effective adoption of smart factory design concepts for Industry 4.0. The proposed solution resulted in an industry-ready, integrated system that allowed for decision-making at multiple levels to improve production line performance, identify bottlenecks, improve product quality, and lower production costs
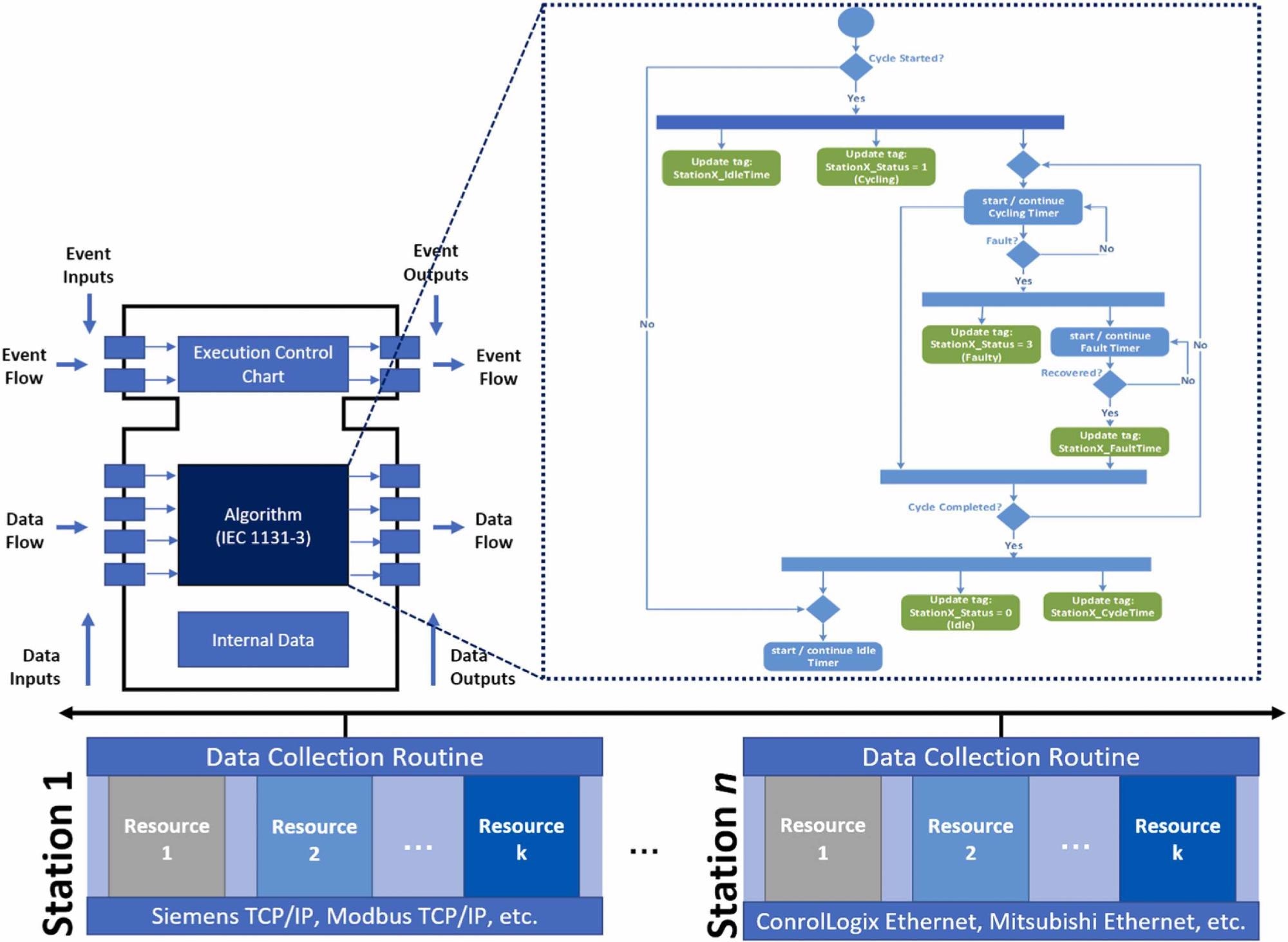
Example custom developed function block for a data collection routine. Image Credit: Kahveci, S et al., Shutterstock.com
Conclusions
In conclusion, this study elucidated the implementation of the proposed solution implemented in an electric vehicle battery module assembly system. Specific layers of the proposed platform, including their individual components, as well as data models and information flows from data collection through batch and real-time data analytics and visualization were defined in this implementation as a prime example of smart manufacturing systems in order to provide actionable insights.
The authors believe that in various respects, this work adds to the scholarly literature and industrial practice. They emphasized that it provides a reference architecture for IoT-based end-to-end big data analytics platforms in cyber-physical production systems that is safe, scalable, interoperable, and resilient.
They also mentioned that future work will involve adding more data points to incorporate decision support systems and improve key performance indicators (KPIs), investigating cloud-based or hybrid deployments, and implementing data preservation policies and data down-sampling methods for improved data maintenance.
Disclaimer: The views expressed here are those of the author expressed in their private capacity and do not necessarily represent the views of AZoM.com Limited T/A AZoNetwork the owner and operator of this website. This disclaimer forms part of the Terms and conditions of use of this website.
Source:
Kahveci, S., Alkan, B., Ahmad, M. K., et al. An end-to-end big data analytics platform for IoT-enabled smart factories: A case study of battery module assembly system for electric vehicles. Journal of Manufacturing Systems 63 214-223 (2022). https://www.sciencedirect.com/science/article/pii/S0278612522000450