A team of scientists from Drexel University in Philadelphia has published a new paper in Additive Manufacturing demonstrating the development of a practical framework that improves the prediction of conversion profiles of parts printed via vat photopolymerization techniques.
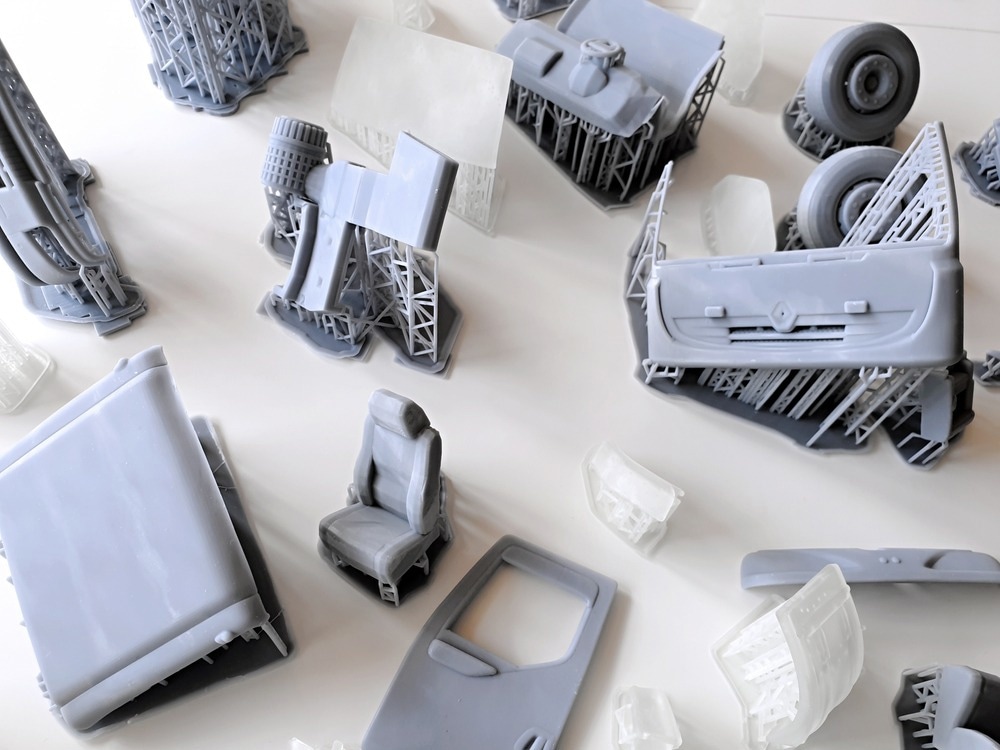
Study: A practical framework for predicting conversion profiles in vat photopolymerization. Image Credit: Archiwo/Shutterstock.com
Vat Photopolymerization
Vat photopolymerization is becoming a central additive manufacturing technology in several industries, producing products with extremely complex internal geometries and functional surface characteristics. This 3D-printing technique has been used to produce microfluidic devices, bespoke medical devices, tailored athletic equipment, and a range of tailored parts for the automotive and aerospace industries.
Developments in methods such as continuous liquid interface production and digital light processing have helped to advance the commercial viability of vat photopolymerization techniques. Several materials have been researched for printing via these methods, with acrylate resins displaying particular suitability as photocurable materials.
There are several critical material properties that affect the quality of products printed with photocurable acrylate resins. These include the material’s modulus, mechanical strength, density, and leachable components. Interaction between factors such as photo-initiator concentration, resin composition, oxygen exposure, and curing temperature plays a key role in controlling photopolymerization.
Conversion Gradients: A Critical Issue in Vat Polymerization
Some critical issues exist with printing these photocurable resins, with one of the fundamental issues being the formation of conversion gradients. This common problem is caused by light attenuation in the media, which forms gradients along the light path. Over the course of several printed layers, this can lead to residual stress, shape distortion, and consequent quality issues in the finished product.
Cracks and delamination can occur in printed parts, leading to pieces being rejected in manufacturing and the limited serviceable life of products. Another issue is light penetration into photocurable materials, leading to problems such as print-through errors. Accuracy along the printing axis is limited by uncontrolled light penetration into curing media.
Strategies to overcome these critical quality issues have been investigated in recent studies, with the addition of photo stabilizers during printing emerging as a forerunner. This strategy limits overcuring, decreases light penetration, and thereby enhances the accuracy of printing. However, this method faces challenges with slow printing speeds, steep conversion gradients between layers, and insufficient mechanical properties in the final printed construct.
The Paper
Conversion profiles are non-uniform and spatiotemporal in nature, making them difficult to predict. Accurately predicting conversion gradients and controlling them is crucial for ensuring the quality and performance of components produced using light-based additive manufacturing techniques. Overcoming this critical issue benefits multiple industrial sectors.
The research has investigated the development of a practical framework to meet this research challenge. The framework presented in the paper incorporates polymerization cure kinetics and accumulated dose profiles. 3D patterning history is computed to provide the dose profiles. Accumulated dose profiles are the distribution of irradiation energy density along the z direction of printing at given points.
The attractiveness of this method is its simplicity: with just two experiments (a cure kinetics study and a working curve experiment,) accurate conversion profile and gradient predictions can be made. A resin’s optical properties can be acquired and combined with 3D-printing parameters (which are user-defined) accumulated dose profiles can be calculated.
Additionally, photo-infrared and photo-calorimetry methods can be employed to obtain cure kinetics data. Spectroscopic absorbance decay and reaction heat release, which are experimental observables, can be analyzed to help obtain this data.
The accumulated dose profile can be obtained using mathematical modeling which includes the optical properties of the resin. These include exposure times, total layer numbers, layer thickness, and so forth. Printing parameters can be modified to tune these profiles. This helps to better control the curing process in each layer of the final product.
Research Conclusions
The authors printed six bar samples with varying layer thickness and exposure time to investigate the effects on conversion profiles. An IR microscope was employed to measure the samples’ conversion profiles along the z-print axis.
The study revealed that effective rather than accumulated dose governed the conversion profile of vat polymerization printed parts. This was due to the cure kinetics’ sub-linear dependence. The influence of layer thickness-resin penetration depth ratio was discussed by the authors. 25% of cure kinetics predictions under-estimated real conversion profiles, whereas over 35% of cure kinetics had accurate profile predictions.
Limitations in the method were identified, such as a low irradiance range, which negatively impacted the speed of reaction rates. Another critical limitation is the method’s assumption of isothermal reactions, which limits its applicability to printing methods. Further work will be performed on creating a more robust model.
There are opportunities with the method, however. Resins with higher depths of penetration can be used to improve print quality and speed, and the framework can be applied to grayscale vat polymerization methods, which can achieve higher print fidelity. This will enhance the commercial application of vat polymerization in several key industries.
More from AZoM: How Does Laser Cleaning Technology Function?
Further Reading
Tu, J., et al. (2022) A practical framework for predicting conversion profiles in vat photopolymerizations Additive Manufacturing 103102 [online, pre-proof] sciencedirect.com. Available at: https://doi.org/10.1016/j.addma.2022.103102
Disclaimer: The views expressed here are those of the author expressed in their private capacity and do not necessarily represent the views of AZoM.com Limited T/A AZoNetwork the owner and operator of this website. This disclaimer forms part of the Terms and conditions of use of this website.