An international team of scientists from Greece, Spain, Switzerland, France, the USA, and The Netherlands have collaborated on a new paper investigating the application of solar irradiance nowcast algorithms to the field of solar forecasting. Their research has been published in the journal Energies.
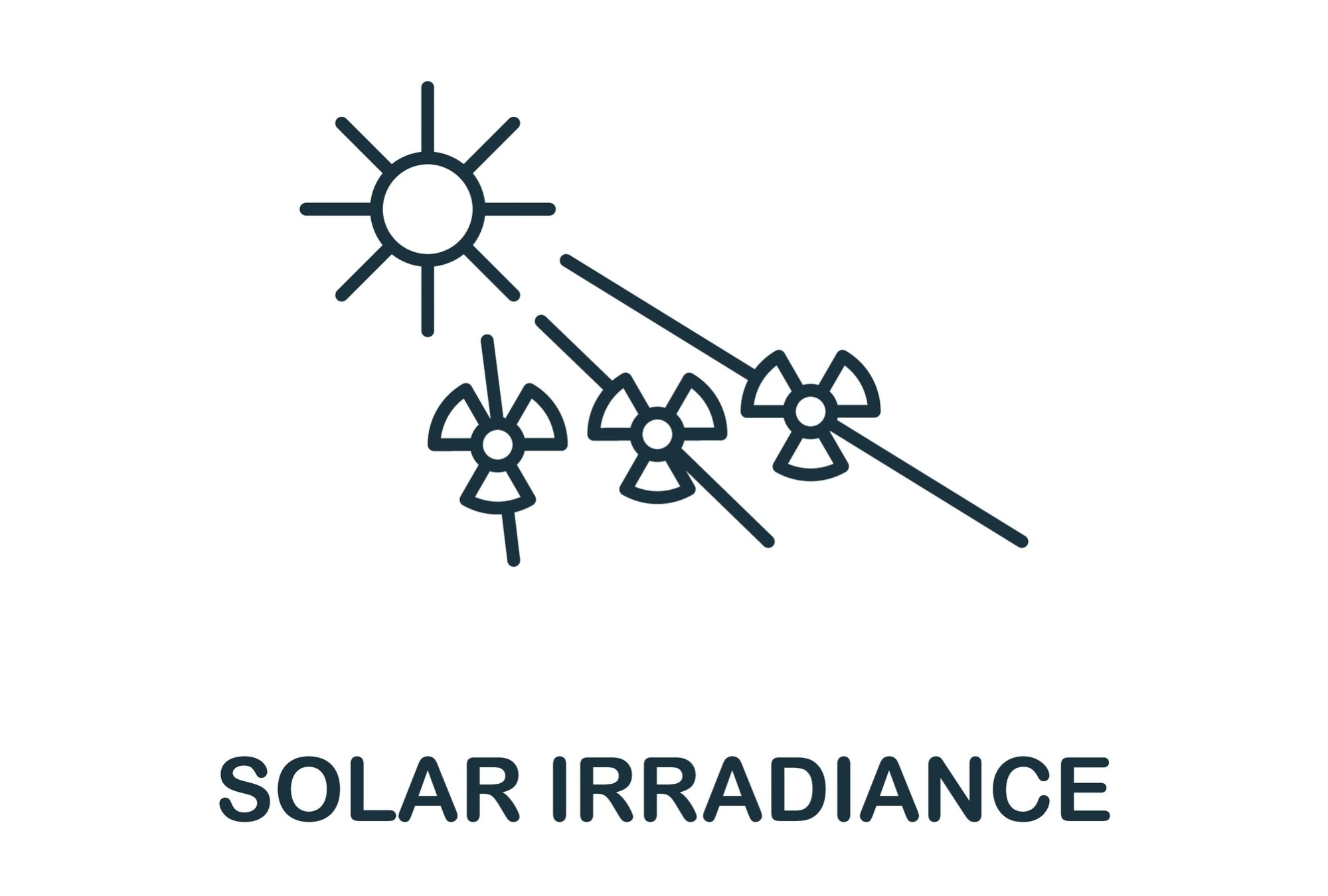
Study: Solar Irradiance Ramp Forecasting Based on All-Sky Imagers. Image Credit: Simakova Mariia/Shutterstock.com
Solar Forecasting and Ramp Events
Solar forecasting is an indispensable tool for the solar energy sector. The efficiency of photovoltaic technologies can be significantly impacted by environmental factors such as cloud cover, meaning that accurate forecasting of solar irradiance is crucial for ensuring that solar power technologies perform optimally. Consequently, accurate and efficient solar forecasting can markedly improve grid stability.
Forecasting is a complex undertaking that can be impacted by sudden changes in solar irradiance in a short time period. These sudden changes are known as ramp events. Currently, there is no formal consensus on the definition of a ramp event in the literature. Accurate definitions largely depend on the user and application.
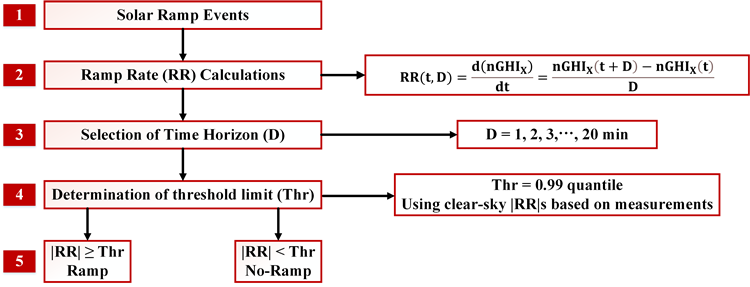
Flowchart of the applied methodology. Image Credit: Logothetis, S-A et al., Energies
A ramp event can be identified by calculating absolute power differences between the start and end point over a period of time. However, because solar irradiance is a dynamic and changeable factor, it is not always possible to accurately identify and forecast a ramp event. Strategies can be employed to overcome these issues but rely on user-defined thresholds for discriminating ramp events from non-ramp events.
Two main categories of ramp events have been defined in the literature: solar irradiance and solar power ramp events. Solar power installations can handle small amounts of uncertainty and variability, but there is a pressing need for the accurate detection of extreme events that installations may not be able to cope with.
Several ramp event detection strategies have been introduced in recent studies, with algorithms featuring heavily in these methods. Swinging door algorithms have been investigated extensively by more than one study, and another strategy is the use of all-sky imagers (ASIs) to provide measurements for deep-learning methods.
One study has attempted to correlate cloud types retrieved from satellite images with the variance in solar radiation. Another study has combined cloud information with neural networks, employing nowcast algorithms. Whilst there is a pressing need to provide accurate solar forecasting capabilities for the solar power sector, this area has been largely unstudied.
The Paper
This study is a benchmarking exercise within the IEA’s Photovoltaic Power Systems Program’s framework. The aim of this international framework is to enhance collaboration in the field of solar power energy to investigate the key role of photovoltaic technologies in the green economy.
The first benchmarking exercise in the study focuses on all-sky irradiance nowcasting. The exercise utilized data from a 2019 campaign in Spain which incorporated a bouquet of all-sky irradiance-based methodologies. Specifically, four systems were used in the project. Validation data revealed the efficacy of this approach irrespective of cloud cover conditions.
The second benchmarking exercise aim is to investigate the feasibility of using all-sky irradiance nowcasts for ramp event forecasting. Brief descriptions of methodologies and applied data are provided in the study, along with the methodology of solar irradiance ramp event detection. The main aim of the study is to evaluate the efficacy of all-sky irradiance nowcasts for the prediction of solar irradiance ramp events.
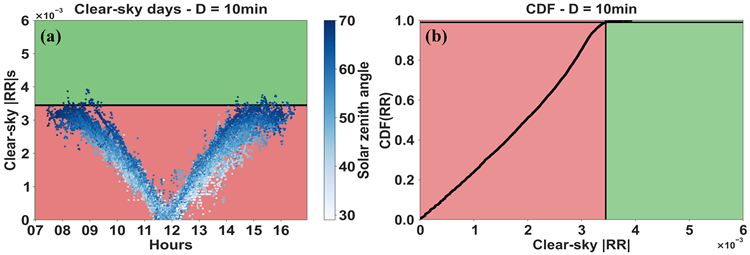
(a) Scatter plot and (b) CDF function of ramp rates (RRs) for clear-sky conditions at time horizon D=10 min. The solid black lines indicate the threshold limit (99th quantile) that classifies the no-ramp (red area) and ramp (green area) events. The blueish color bar refers to the solar zenith angle. Image Credit: Logothetis, S-A et al., Energies
Study Conclusions
The study presents a novel approach to detecting and predicting solar irradiance ramp events using all-sky irradiance nowcast algorithms. Some important conclusions were drawn in the paper.
The connection between detected ramp events and all-sky irradiance-based nowcast algorithms was revealed in the paper. The algorithms can detect 55% to almost 100% of ramp events. However, it has been noted in the study that ASI-2 systems, which predict 65% of ramp events, produce spatial GHI forecasts and were confined to narrow areas in the benchmark, penalizing physical approaches, especially for cloud geolocalization.
To overcome this issue, it is recommended to incorporate wider GHI forecast areas, which consequently enhances ramp event detection. Furthermore, ASIs 3-5 suffer from false predictions that are not encountered using ASI 1 and 2 systems. Increasing the forecasting horizon decreases the accuracy of ASI-based systems. On average, the forecasting accuracy for all systems is approximately 80%.
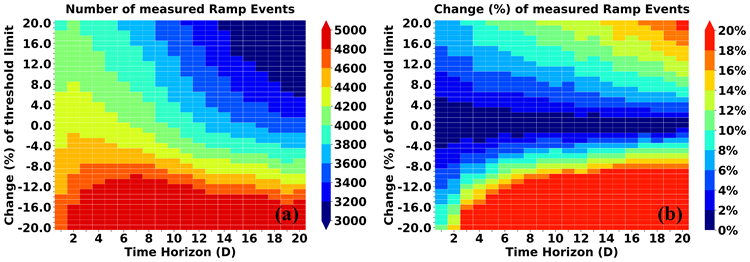
(a) Total number of measured ramp events and (b) the change of this number caused by varying the threshold limit (Thr) at each time horizon (D). The vertical axis shows the change of the threshold limit, whereas the horizontal axis presents D. The color bar displays the number of ramp events (a) and the change of the number of ramp events (b). Image Credit: Logothetis, S-A et al., Energies
The relationship between forecast accuracy and the underlying cloud conditions was also revealed in the study. Different cloud conditions were analyzed, with ASI 3-5, which are deep-learning algorithms, outperforming other algorithms on days with overcast or scattered cloud conditions. Algorithms that incorporate physical steps perform best on days with scattered clouds during half the day and cloudlessness during the other half.
ASI-based nowcast algorithms were also compared to other conventional forecasting strategies such as MAD and RMSD, demonstrating the enhanced performance of the forecasting strategy investigated in the study. ASI-based nowcast algorithm selection depends on the application. Furthermore, the authors have recommended that a combined approach would be beneficial for ramp event prediction accuracy.
Overall, the paper has demonstrated the suitability of all-sky irradiance-based nowcast algorithms as an integrated tool for solar irradiance ramp event forecasting prediction. This will improve the performance of solar power installations during extreme power fluctuation events, improving efficiency and reducing costs.
Further Reading
Logothetis, S-A et al. (2022) Solar Irradiance Ramp Forecasting Based on All-Sky Imagers Energies 15(17) 6191 [online] mdpi.com. Available at: https://www.mdpi.com/1996-1073/15/17/6191
Disclaimer: The views expressed here are those of the author expressed in their private capacity and do not necessarily represent the views of AZoM.com Limited T/A AZoNetwork the owner and operator of this website. This disclaimer forms part of the Terms and conditions of use of this website.