The number of pharmaceuticals used worldwide is expected to increase to 4 billion doses by 2020 due to strong annual growth. A growing number and variety of trace substances are discovered in sewage and wastewater treatment plants as a result of drugs being metabolized by the human body and entering these facilities.
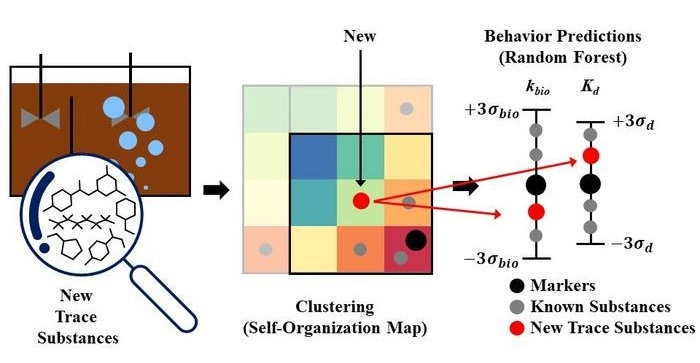
Machine learning approaches for predicting the behavior of new trace substances. Image Credit: Korea Institute of Science and Technology (KIST)
These trace materials can cause endocrine disruption and cancer when they get into rivers, seas, and other bodies of water. They can also have detrimental impacts on human health.
As a result, technologies are required to swiftly and effectively anticipate these trace substances’ features and behaviors; yet, evaluating unknown trace compounds is a labor-intensive process that takes a lot of time, expensive equipment, and experienced specialists.
The Korea Institute of Science and Technology (KIST) announced that a group headed by senior researcher Son Moon and Water Resources and Cycle Research Center head Hong Seok-won had created a system that uses artificial intelligence-based clustering and prediction to predict the concentrations of emerging trace materials based on their physicochemical characteristics.
The researchers classified 29 known trace substances, including pharmaceutical compounds and caffeine, using self-organizing maps, an AI technique that groups data into maps based on similarities. The information included in the maps included physicochemical properties, functional groups, and biological reaction mechanisms.
Then, further random forests were constructed to forecast the characteristics and concentration variations of novel trace materials. Random forests are a machine learning approach that divides data into subgroups. The characteristics of other chemicals in a cluster in the self-organizing map can be used to forecast how the properties and concentration of a newly discovered trace substance will vary.
The prediction accuracy of around 0.75 was achieved by applying this AI model (self-organizing map and random forest) for the grouping and prediction of 13 novel trace chemicals. This is significantly higher than the 0.40 prediction accuracy of current AI approaches that utilize biological information.
The data-driven analysis model developed by the KIST research team has an advantage over conventional formula-based prediction methods in that it only requires the physicochemical properties of trace substances.
By clustering substances with similar data, it can effectively predict how the concentration of new trace substances will change during the sewage treatment process. Furthermore, in the future, the data-driven AI model may be used to forecast the concentration of novel compounds, including pharmaceuticals that raise societal concerns.
Dr. Seokwon Hong and Dr Moon Son (co-corresponding authors) of KIST added, “It can be applied not only to actual wastewater treatment plants, but also to most water treatment-related facilities where new trace substances exist, and can provide quick and accurate data in the policy-making process for related regulations. Since it utilizes machine learning technology, the accuracy of the prediction will improve as relevant data is accumulated.”
Journal Reference
Lim, S.J., et. al. (2024) Clustering micropollutants and estimating rate constants of sorption and biodegradation using machine learning approaches. npj Clean Water. doi:10.1038/s41545-023-00282-6