The introduction of silicon drift detector (SDD) technology enables rapid performance of energy dispersive spectrometry (EDS) using micro-X-ray fluorescence spectrometry (Micro-XRF) and scanning electron microscopes (SEM). The combination of benchtop Micro-XRF and SEM-EDS ensures an advanced workflow for characterizing the ore, as shown in the Table 1.
Table 1. Workflow for the characterization of ore samples
Task |
Method |
Option |
Resolution |
Time / min |
1. Detect |
Benchtop Micro-XRF |
Spectrum imaging |
~25 µm |
30 – 120 |
2. Locate |
High kV SEM-EDS |
Automated feature analysis |
~3 µm |
60 – 120 |
3. Analyze |
Low kV SEM-EDS |
Spectrum imaging |
~50 nm |
~20 |
This article describes the analysis of ore-thick sections from an offset dike of the Sudbury Igneous Complex (SIC), using Micro-XRF and SEM-EDS.
The analysis involves detecting the presence of high demand elements such as Co, Ni, Cu, Pt, Pd, As, and Te using Micro-XRF to determine the ore values. Following this, mineral phases of economic interest are located.
The mineral phases were then analyzed at high spatial resolution with the help of low accelerating voltages.
Experimental
Micro-XRF is used for determining the distribution of elements, with Z > 10 and spatial resolution over 25µm. The Bruker M4 TORNADO Micro-XRF spectrometer can be used to map the samples with sizes up to 20 x 16cm2 in 4h to identify the regions of interest for high-resolution SEM studies.
The QUANTAX EDS system is used to classify minerals in large areas using automated feature with SEM stage control, which is a combination of morphological classification with chemical analysis. The measurement and evaluation time is reduced through the analysis of features of interest upon selecting the corresponding threshold in the backscattered electron (BSE) micrograph.
Spectra were obtained either by scanning the complete grain, or through point measurements in the center of each grain. The grains can then be relocated for further analysis by saving stage coordinates in spectrum imaging techniques. Table 2 shows the optimal analytical conditions for automated feature analysis using SEM-EDS.
Table 2. Optimal analytical conditions for automated feature analysis using SEM- EDS
Parameter |
Conditions |
Remarks |
BSE threshold |
Multiple / single |
All / selected grains |
BSE pixel resolution |
~1 µm |
3 times better than smallest feature of interest |
HV |
25 kV |
Spatial resolution in the µm range |
Pulse processor setting / dead time |
130 kcps / 30% |
80 – 160 kcps input count rate, ~90 kcps output count rate |
|
0.5 – 1 s |
|
Results and Discussion
The Micro-XRF composite intensity map as shown in Figure 1a illustrates the mineral distributions, including that of chalcopyrite (copper iron sulfide), pentlandite (iron nickel sulfide), iron oxides and iron sulfides. The minerals of potential benefits like cobaltite-gersdorffite can also be detected using Micro-XRF, as shown in the Figure 1b.
The high sensitivity of Micro-XRF facilitates the display of cobalt enrichment in pentlandite as shown in the Figure 1b. This analysis cannot be performed using SEM-EDS owing to the low concentration of cobalt and the peak overlap of the cobalt K lines with the iron K lines.
.jpg)
.jpg)
Figure 1. Sample from the Sudbury Igneous Complex Parkin dike; (a) Composite Micro-XRF map of iron K, nickel K, copper K, and silicon K of a thick section (44 x 24 mm2, M4 TORNADO Micro-XRF spectrometer with 2 x 30mm2 XFlash® SDD, 50kV, 600µA, 310kcps, 2h, 20mbar chamber pressure, 2210 x 1185 pixels2, 20µm pixel size, and 2ms dwell time, 120min total measurement time). (b) Micro- XRF map of cobalt K. Note the association of cobalt and nickel with pentlandite (yellow arrow) and arsenide grains (red arrow).
Minerals shown in Table 4 were classified in the analyzed areas based on the parameters shown in Table 3. This is illustrated in the Figure 2. All the minerals can be classified using single thresholds by selecting the corresponding threshold in the BSE micrograph, as shown in the Figure 3.
Table 3. Analytical parameters used for the samples
Parameter |
Worthington dike |
Parkin dike |
Trill dike |
Measured area |
1.3 x 0.9 cm2 |
3.3 x 1.8 cm2 |
1.5 x 1.1 cm2 |
Measurement time |
292 min |
120 min |
35 min |
|
Multiple (all grains) |
Bright (As, Te) |
|
Table 4. Feature analysis results for all samples, presenting count and area fraction of every class determined
Class |
Worthington dike |
Parkin dike |
Trill dike |
Count |
Area /% |
Count |
Area /% |
Count |
Area /% |
Chalcopyrite |
607 |
3.0460 |
n.a. |
n.a. |
n.a. |
n.a. |
Pentlandite |
4126 |
3.4500 |
n.a. |
n.a. |
n.a. |
n.a. |
Iron sulfide |
1058 |
55.0754 |
n.a. |
n.a. |
n.a. |
n.a. |
Silicates |
324 |
0.0379 |
1 |
0.000003 |
n.a. |
n.a. |
Co-Ni-arsenide |
40 |
0.1140 |
9 |
0.000117 |
n.d. |
n.d. |
Pt-arsenide |
n.d. |
n.d. |
5 |
0.000045 |
n.d. |
n.d. |
Arsenide |
2 |
0.0006 |
n.d. |
n.d. |
n.d. |
n.d. |
Pd-Bi-telluride |
n.d. |
n.d. |
8 |
0.000294 |
12 |
0.00065 |
Pt-Bi-telluride |
n.d. |
n.d. |
n.d. |
n.d. |
1 |
0.00008 |
Bi-telluride |
n.d. |
n.d. |
2 |
0.000011 |
n.d. |
n.d. |
Sb-Pb-phase |
n.d. |
n.d. |
n.d. |
n.d. |
9 |
0.00049 |
Pb-phase |
2 |
0.0020 |
1 |
0.000053 |
30 |
0.00139 |
Ag-phase |
5 |
0.0004 |
1 |
0.000006 |
1 |
0.00001 |
Monazite |
n.d. |
n.d. |
19 |
0.000082 |
n.d. |
n.d. |
Th-phase |
1 |
0.0004 |
8 |
0.000034 |
n.d. |
n.d. |
Zr-phase |
10 |
0.0022 |
50 |
0.000389 |
n.d. |
n.d. |
Baryte |
n.d. |
n.d. |
1 |
0.000004 |
n.d. |
n.d. |
Sphalerite |
7 |
0.0007 |
n.a. |
n.a. |
n.a. |
n.a. |
Unclassified |
169 |
0.0322 |
n.d. |
n.d. |
n.d. |
n.d. |
|
6351 |
61.7618 |
105 |
0.001037 |
53 |
|
.jpg)
.jpg)
.jpg)
Figure 2. BSE mosaics of (a) Worthington dike (sample 804014, 15072 x 10061 pixels2, 90 fields). The highlighted area is a single field shown in detail below. (b) Trill dike (sample 703351/1, 12560 x 9420 pixels2, 256 fields). (c) Parkin dike (Milnet Mine, sample LH09-41, 27456 x 14713 pixels2, 875 fields)
.jpg)
Figure 3. Example of a single analysis field (highlighted in Fig. 2a) showing the distribution of iron sulfide (yellowish), chalcopyrite (blue), pentlandite (dark green with arrows) and cobalt nickel arsenic sulfide(red with arrows).
Minerals of economic interest, such as tellurides and arsenides, can be defined by choosing a bright threshold that reduces the measurement time. BSE pixel resolution of ~1µm can be used to detect grains of size greater than 3µm, as shown in the Figure 4.
.jpg)
Figure 4. Size (average diameter) of 13 Pd-Pt-bismuth telluride grains of the Trill dike
Conclusion
The combination of Micro-XRF and SEM-EDS helps improve data collection of samples. Micro-XRF helps resolve the differences between the highest spatial resolution of SEM-EDS and the macro sample, apart from providing the high sensitivity for trace elements.
The automated feature analysis enables easy localization of mineral grains of economic interest in a thin or thick section within 2h.
It can therefore be said that the recent advancements in software, pulse processor and detector technology have expanded the applications of Micro-XRF and SEM-EDS to applied and process mineralogy.
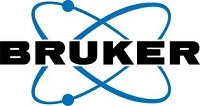
This information has been sourced, reviewed and adapted from materials provided by Bruker Nano Analytics.
For more information on this source, please visit Bruker Nano Analytics.