Investigators rely on particle characterization methods to gain a deeper understanding of key properties that influence both the manufacturing process and the quality of the final product.
As development progresses, these characterization methods are typically integrated into the manufacturing workflow, where various parameters are controlled to ensure consistent manufacturability and product efficacy.
For decades, particle size has been a cornerstone of particle characterization. However, most established techniques for measuring particle size rely on indirect methods, which assume that all particles are round. Since most particles in industrial applications are not round, these techniques often fall short of providing sufficient characterization information, raising concerns about their reliability.
Particle size has been a fundamental characterization technique for many years. Most existing particle size characterization approaches are indirect measurement methods that portray particle size data, believing all measured particles are round. However, most industry particles are not round, casting doubt on size-only techniques to provide adequate characterization data.
Irregular particle shapes can significantly impact how particles interact, flow, and compact, ultimately influencing the performance of end products. To address these limitations, scientists began to adopt particle characterization based on both shape and size using direct measurement methods, such as microscopy. Although microscopy is slow and labor-intensive, it provides valuable qualitative insights into the properties of raw material particles.

Image Credit: Vision Analytical Inc.
Image Analysis as it applies to particle shape was first explored in 1963 when the "Krumbein Scale" was presented to geologists who wanted a standardized way to measure particle roundness and sphericity.
This scale was visual, with sample images on a sheet of paper to compare particles, and was subjective. However, this demonstrates that for many decades, the need to understand particle shape has been nearly as critical as particle size.
As time progressed, scientists used microscopes to view small sample populations to better understand the shape of their particles.
It was not until the early 1990s, with the advent of improved machine vision cameras and higher-performing computers, that particle shapes could be analyzed in a more standardized way with a much higher sampling of particles.
Image Analysis, whether the particles are static or dynamically moving, involves very fast image processing.
The Basis of Dynamic Image Analysis
This high-speed technique can analyze tens of thousands of particles within minutes or even seconds.
To achieve accurate results, the particles are suspended in a fluid, promoting a uniform and homogeneous dispersion. As the fluid flows through a specialized cell, a light source illuminates the particles from one side. On the opposite side, a lens and digital camera capture the particle silhouettes, converting them into grayscale images that are then processed by a computer.

Image Credit: Vision Analytical Inc.
The analysis software processes each image by converting it to a binary format. Rejection and thresholding parameters are applied, and the resulting binary, pixelized image is used to calculate over 30 shape parameters for each particle. Simultaneously, each particle is saved as a thumbnail, providing objective evidence of all measured particles.

Image Credit: Vision Analytical Inc.
More Information on Dynamic Image Analysis
Dynamic image analysis applies camera technology to capture images, so understanding some fundamental facts regarding image taking is crucial, the first of which is the field of view (FOV).
FOV refers to the area (x, y) within the imaging zone where particles are measured. The size of the FOV is determined by the lens magnification; higher magnification results in a smaller FOV. This is similar to zooming in with a camera to capture a detailed image of a small object. Increasing magnification makes smaller particles appear larger on the screen, which is critical for accurate analysis.
With higher magnification, more pixels are allocated to the edges of particles, improving the resolution and accuracy of shape determination since particle shape is calculated based on the pixels obscured by the particles. Conversely, decreasing magnification—or zooming out—expands the field of view, capturing a larger area but with reduced pixel detail for each particle.
Depth of field (DOF) is also crucial in dynamic image analysis. Like basic picture taking, the DOF relates to the regions nearest and farthest from the camera where all objects, or particles, are in focus. Dynamic image analysis will always reject out-of-focus particles.
The lens aperture diameter—the f-stop number—controls the DOF. Decreasing the aperture diameter (increasing the f-stop) expands the DOF because the light traveling at shallower angles passes through the aperture. Only light rays with shorter angles reach the image plane.
The equipment manufacturer will always aim to guarantee the greatest DOF for dynamic image analysis.

Image Credit: Vision Analytical Inc.
One final optical issue is perspective error and correction. Perspective error happens when a wider DOF allows closer particles to appear smaller due to their distance from the imaging source.
The image below, supplied by complements of Edmund Optics, demonstrates that one object looks smaller due to being further away but both objects are in focus within the DOF.
Many dynamic image analysis tools include telecentric optics to correct this error. Failing to correct this error will cause inaccurate data as the particles in the FOV and DOF that are further away can be reported as smaller than the same particles closer to the imaging source.

Image Credit: Vision Analytical Inc.
Benefits of Dynamic Image Analysis
Understanding how dynamic image analysis works provides end users with tangible results.
It is crucial to remember that the most widely used method for particle characterization is particle size measurement, with the most common techniques assuming that all particles are round. However, dynamic image analysis, being a number-based technique, provides much more detailed information. One key advantage is its ability to deliver both volume-weighted and number-weighted particle size distributions.
Number and Volume Weighting
Dynamic imaging, as a counting-based measurement technique, provides statistical size data in both number-weighted and volume-weighted distributions.
Number-weighted distributions are crucial for identifying and visualizing fine particles within a sample, while volume-weighted distributions are essential for detecting small amounts of larger particles, such as agglomerates. Both types of distributions are always calculated and reported.
Additionally, dynamic imaging offers the option to present results as a sieve correlation, allowing for a seamless comparison between traditional sieving methods and automated dynamic imaging, which simplifies method transfer.

Image Credit: Vision Analytical Inc.
The above image shows the results of an abrasive particle’s number-weighted and volume-weighted particle size distribution. This particular shape model estimates particles as if they were round and is commonly used by customers to compare dynamic image analysis results with other size-only techniques.
As shown, the two histograms differ significantly. The number-weighted histogram highlights all the fine particles in the sample, which can be critical depending on the application. For instance, in filtration processes, a high number of fine particles can quickly clog a filter, necessitating close monitoring.
On the other hand, the volume-weighted histogram can be valuable when large particles or agglomerates are a concern.
Counting and Concentration

Image Credit: Vision Analytical Inc.
Dynamic image analysis provides particle counting and concentration measurements by analyzing each individual particle. Concentration values are displayed in real-time as particles per milliliter, while the total count is provided at the end of the analysis. This count can represent all particles in the sample or be specific to a particular class or type of particle.
Such measurements are critical when dealing with samples containing rare events or when understanding concentration levels within any population or sub-population is necessary.
Counting instruments, including coulter counters and dynamic imaging systems, also account for dilution factors, automatically adjusting for any pre-dilution of the sample to ensure accurate results.
Viewing Questionable Statistics
A key advantage of dynamic image analysis is the ability to visually inspect the particles that contribute to a questionable statistical result.
In the example below, size-only instruments might produce statistical histograms with data that cannot be verified. Dynamic imaging, however, enables users to view the individual particles corresponding to any area of a size or shape distribution. This provides objective evidence and an added layer of confidence for end users—something unique to dynamic image analysis.

Image Credit: Vision Analytical Inc.
Comparisons
Dynamic imaging enables end users to compare different sample lots or analysis runs based on both size and shape. This is particularly valuable because samples may not exhibit noticeable differences in size but can show significant variations in shape.

Image Credit: Vision Analytical Inc.
Correlation Plots
Correlation plots enable users to observe all the analyzed particles to find rare events. Without this correlation function, detecting a particle or a set of particles within an analysis of tens of thousands of particles would be nearly impossible. Correlation plots can be constructed with any two available shape measures.

Image Credit: Vision Analytical Inc.
Classification
Having more than 32 shape measurements for each particle provides a significant advantage by making it easier for the software to differentiate between particles. In a multi-component sample, the extensive shape data allows for particle classification, a feature that enables the software to separate and quantify subpopulations of particles within the sample.

Image Credit: Vision Analytical Inc.
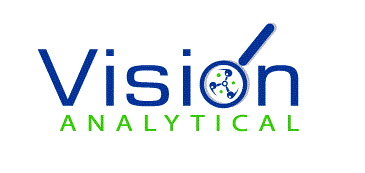
This information has been sourced, reviewed and adapted from materials provided by Vision Analytical Inc.
For more information on this source, please visit Vision Analytical Inc.