This article discusses the role of chemometrics in gas analysis and recent developments in this field.
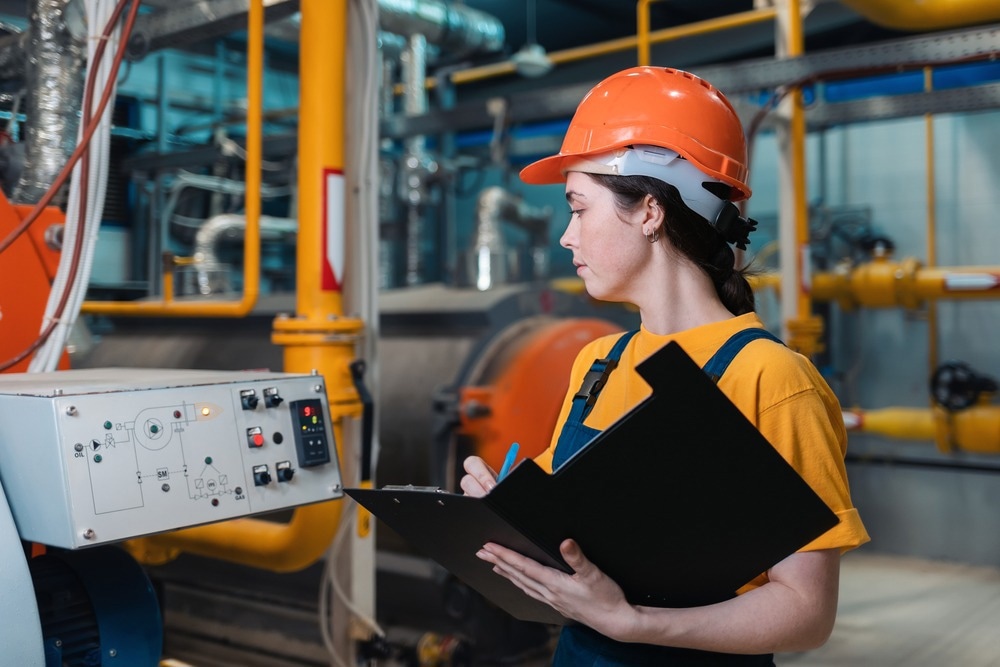
Image Credit: STEKLO/Shutterstock.com
Chemometrics-based Gas Chromatography (GC) Analysis
GC is primarily based on the principle of differential distribution/partition of a volatile compound/gas between a gaseous mobile phase and a liquid/solid stationary phase in a fixed set of parameters. GC-mass spectrometry (GC-MS), a technique that uses a mass spectrometer as a GC system detector, is used extensively for modern GC as it can effectively identify compounds in mixtures and detect and quantitate volatile trace components.
GC/GC-MS is performed to analyze flavors and fragrances, pharmaceutical drugs, environmental pollutants, and compounds in beverages, foods, and agricultural products. GC/GC-MS also plays a crucial role in the analysis of biological samples, such as different xenobiotics and volatiles emitted from bodily fluids/skin.
The use of GC-MS to investigate different complex samples generates a substantial amount of analytical information, which is decoded using chemometrics-based approaches.
Chemometrics is a chemical discipline that employs statistical, mathematical, or other methods based on formal logic to select/design optimal measurement experiments and procedures and provide maximum chemical information through chemical data analysis.
Chemometrics methods play a critical role as a highly-efficient data optimization and analysis strategy by revealing the hidden chemical knowledge/information in high-dimensional datasets obtained from multidimensional separations. The GC analysis dimensionality is demonstrated by the application of comprehensive two-dimensional (2D) GC (GC × GC) and GC-MS.
In GC analysis, the application of chemometrics can be categorized into data analysis and data preprocessing. Data preprocessing strategies render the GC data ready for precise quantification and identification.
These strategies include background correction, which involves smoothing and denoising to remove the high-frequency signals irrespective of their amplitude and low-amplitude signals irrespective of their frequency, retention time alignment to correct the inter-run retention time variation for similar samples, and drift correction to subtract the baseline shape from a measurement.
A series of steps is performed after data preprocessing to translate complex data into meaningful information for a sample. These steps include peak detection, which involves identifying true signals in chromatograms, information extraction by applying data dimension reduction, and classification to distinguish between sample classes with various chemical characteristics.
Common Chemometric Tools for GC Data Analysis
Chemometric tools used for baseline correction during data preprocessing include Savitzky-Golay, asymmetric least squares, polynomial fitting, penalized least squares, moving window minimum value, and local minimum values coupled with robust statistical analysis.
Automatic peak detection and background drift correction, local minimum value, and correlation-optimized warping are the chemometric tools employed for retention time alignment. In data analysis, multiple chemometric tools are used for unsupervised pattern recognition and supervised pattern recognition during classification.
These include principal component analysis (PCA), hierarchical cluster analysis (HCA), and cluster analysis (CA) for unsupervised pattern recognition and partial least squares discriminant analysis (PLS-DA), support vector machine (SVM), soft independent modeling of class analogy (SIMCA), random forest (RF), k-nearest neighbor (KNN), quadratic discriminant analysis (QDA), and linear discriminant analysis (LDA) for supervised pattern recognition.
Additionally, chemometric tools utilized for quantification include partial least squares regression (PLSR), artificial neural networks (ANN), multivariate curve resolution-alternating least squares (MCR-ALS), parallel factor analysis (PARAFAC), and generalized rank annihilation (GRAM).
PLSR is used extensively to calculate and correlate the sensory and gas chromatographic data models as the method can determine the covariance of two matrices and analyze data with missing values and multicolinearity.
However, PLSR methods can only model linear relations, which is a major disadvantage as the relation between sensory perception and concentration of volatiles is non-linear. Thus, future studies must develop robust non-linear methods to more effectively analyze the relations between volatiles and sensory data.
In a paper published in the Journal of Chromatography A, researchers used GC-MS to characterize the Iranian rosemary essential oil components. The multivariate curve resolution (MCR) method was utilized to address the problem of baseline offset, background, and embedded/overlapping peaks in GC-MS.
The GC-MS data analysis demonstrated the presence of 68 components in the rosemary essential oil. However, the application of MCR increased the number of components with over 0.01% concentration to 99, which accounted for 98.23% of the total relative rosemary essential oil content.
Recent Developments and Challenges
In a recent study published in the journal Molecules, researchers evaluated the feasibility of using a proposed non-targeted approach based on the combination of chemometrics and headspace flash gas-chromatography with flame ionization detection (HS-GC-FID) to detect adulteration of saffron with two plant-derived adulterants, including marigold and turmeric.
PLS-DA and LDA were employed to perform chemometric modeling from the GC data. The LDA and PLS-DA chemometric models were validated by external validation and cross-validation. Both models displayed satisfactory results with functional external and internal prediction ability, which indicated the effectiveness of the proposed approach as a low-cost rapid screening method for saffron authentication.
Although the chemometric approaches allow the use of correlations among variables, the constants in chemometric models do not possess physical relevance. Moreover, determining the effective chemometric methods for GC analysis is extremely difficult as most chemometric approaches have been used to address a specific challenge within a dataset and were not frequently compared with other approaches.
Thus, more research is required using different types of data and chemometric tools to thoroughly assess the advantages and disadvantages of every chemometric tool and determine the most effective tool that can deliver the best results for GC analysis.
More from AZoM: Gas Analysis in Photovoltaic Cell Production
References and Further Reading
Jalali-Heravi, M., Moazeni, R. S., Sereshti, H. (2011). Analysis of Iranian rosemary essential oil: Application of gas chromatography–mass spectrometry combined with chemometrics. Journal of Chromatography A, 1218(18), 2569-2576. https://doi.org/10.1016/j.chroma.2011.02.048
Seisonen, S., Vene, K., Koppel, K. (2016). The current practice in the application of chemometrics for correlation of sensory and gas chromatographic data. Food Chemistry, 210, 530-540. https://doi.org/10.1016/j.foodchem.2016.04.134
Morozzi, P., Zappi, A., Gottardi, F., Locatelli, M., Melucci, D. (2019). A Quick and Efficient Non-Targeted Screening Test for Saffron Authentication: Application of Chemometrics to Gas-Chromatographic Data. Molecules, 24(14), 2602. https://doi.org/10.3390/molecules24142602
Hoang, V. D., David, V., Moldoveanu, S. C. (2023). Introductory Chapter: Novel Aspects in Gas Chromatography and Chemometrics. IntechOpen. https://doi.org/10.5772/intechopen.109943
Disclaimer: The views expressed here are those of the author expressed in their private capacity and do not necessarily represent the views of AZoM.com Limited T/A AZoNetwork the owner and operator of this website. This disclaimer forms part of the Terms and conditions of use of this website.