Writing in the journal Materials, a team of scientists from China has reported the use of neural networks for modeling hyperelastic material structures to identify material parameters. The authors have stated that their proposed approach is universal and has high precision.
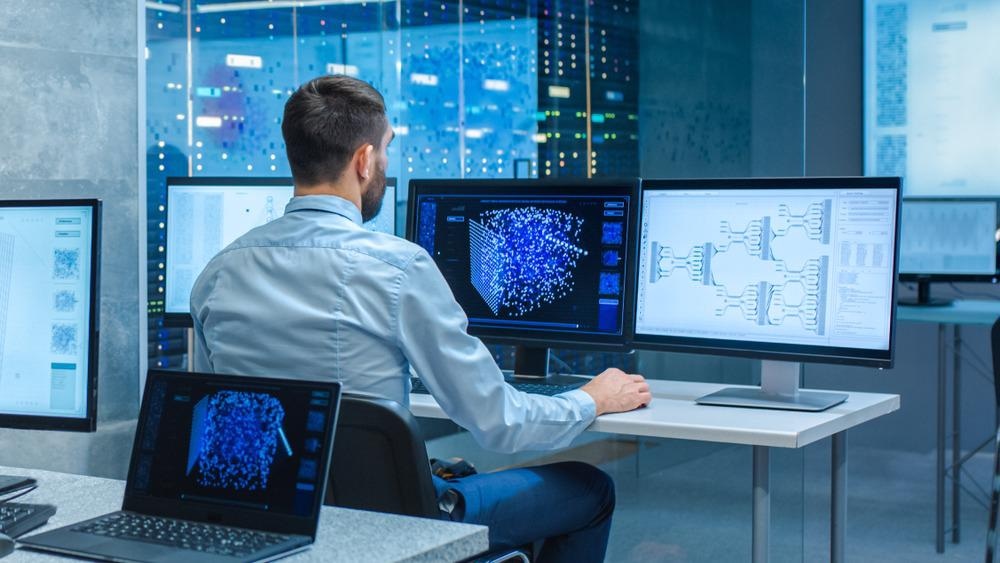
Study: Parameters Identification of Rubber-like Hyperelastic Material Based on General Regression Neural Network. Image Credit: Gorodenkoff/Shutterstock.com
Background to the Research
Hyperelastic materials possess several excellent qualities such as wearability, shock resistance, and elasticity, and can undergo extreme deformation, making them exceedingly durable. Many hyperelastic materials, including gels and rubbers, have found commercial use in engineering applications such as insulation materials.
In recent years, researchers have explored the use of simulations and numerical models to predict and describe experimental phenomena in these materials. Establishing a constitutive model has become a key research concern for the design of advanced hyperelastic materials.
Studies have demonstrated that hyperelastic materials have nonlinear characteristics, and their properties depend on their strain energy function. Several models such as the Gent model, Ogden model, Mooney-Rivlin model, and Gent-Thomas model have been developed to provide suitable strain energy density for these materials.
The specimen and its geometry employed in a uniaxial tensile test: (a) The image of silicone rubber specimen with bonded aluminum sheets; (b) The dimensions of silicone rubber specimen and aluminum sheets. Image Credit: Hou, J et al., Materials
Incompressible models have become a key model variant due to their ability to simplify theoretical work and, consequently, provide crucial information on the properties of hyperelastic materials. There has been an increased focus on the investigation of hyperelastic models to aid the further application of these commercially important materials.
Common approaches to determine the parameters of these materials include experiments, numerical calculations, and the use of artificial intelligence. Artificial intelligence has received increased research attention in recent years due to its ability to predict parameters and material behavior, which experiments and simulations find challenging.
Artificial intelligence methods can use existing data to establish the relationship between variables. This gives these methods a significant advantage over traditional analysis. AI has been successfully employed to optimize mechanical models, predict mechanical parameters, and monitor the health of materials and components along with several other aspects.
The Study
The study has investigated the use of neural networks to improve the modeling of hyperelastic material mechanical parameters. The authors have used an approach based on the General Regression Neural Network (GRNN,) a type of radial basis function neural network.
GRNN has been widely applied in many fields and has several advantages over conventional neural networks. It possesses a simpler network structure than feedforward neural networks. Model training is straightforward and simple – as soon as learning samples pass through the model’s hidden layer, training is complete. This reduces both training time and costs.
In addition, GRNN calculations have better global convergence capabilities than feedforward networks, which usually only achieve local convergence.
The aim of the paper is to create a simple, effective, and convenient GRNN-based approach. The purpose of the proposed neural network-based approach is to accurately identify the model parameters of hyperelastic materials. The approach combines experimental analysis, numerical modeling, simulations, and GRNN learning.
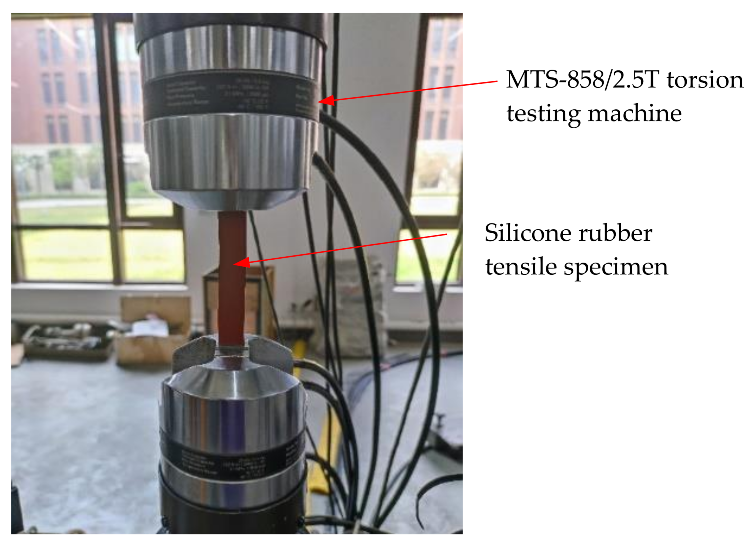
The experimental device of a uniaxial tensile test. Image Credit: Hou, J et al., Materials
The theoretical basis of GRNN and its architecture has been discussed in-depth in the study, along with the application of this approach for determining material parameters. A brief introduction of the hyperelastic model is provided by the researchers, along with prediction schemes based on GRNN, finite element analysis, and uniaxial testing.
In addition, the paper has provided a validation example using silicone rubber. Uniaxial tensile tests were used to measure the material’s force-displacement curve. Experimental results demonstrated nonlinear characteristics of the material. A numerical model based on finite element simulation was employed to obtain learning samples for the neural network to identify parameters.
Results and Conclusions
The authors designed a simple, convenient, and efficient method for identifying parameters in hyperelastic models. The approach combined experimental observations, numerical modeling, and GRNN. Target values were provided by experiments, with the numerical models being used to provide learning samples for the neural network. GRNN was used to identify the parameters.
The GRNN-based approach improved the accuracy of parameter prediction for three commonly used models: the M-R model, polynomial model, and Ogden model. However, whilst the results of all three hyperelastic models met accuracy requirements, the Ogden model produced a force-displacement curve that was closest to experimental data.
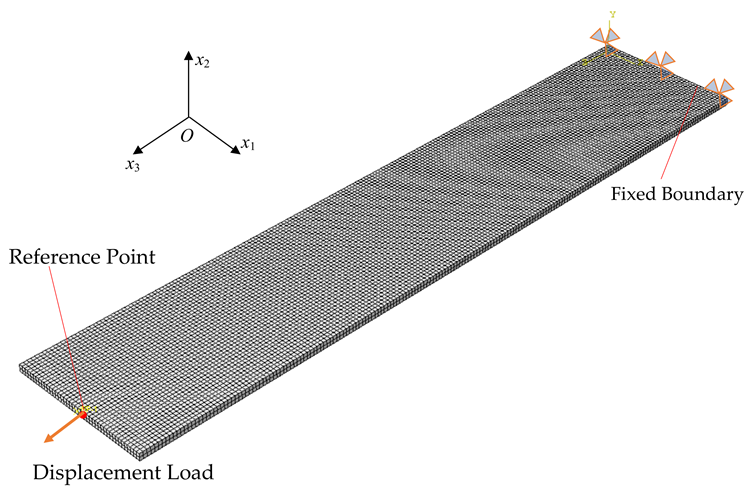
Finite element mesh and boundary conditions of silicone rubber specimen. Image Credit: Hou, J et al., Materials
Based on this, the authors concluded that the Ogden model was most suitable for characterizing the silicone rubber’s mechanical properties. The authors have stated that this GRNN-based approach could be considered a universal approach for addressing problems when identifying multiple parameters. GRNN is, therefore, a powerful forecasting tool that can automatically optimize material parameters.
Further Reading
Hou, J et al. (2022) Parameters Identification of Rubber-like Hyperelastic Material Based on General Regression Neural Network Materials 15(11) 3776 [online] mdpi.com. Available at: https://www.mdpi.com/1996-1944/15/11/3776
Disclaimer: The views expressed here are those of the author expressed in their private capacity and do not necessarily represent the views of AZoM.com Limited T/A AZoNetwork the owner and operator of this website. This disclaimer forms part of the Terms and conditions of use of this website.