In a paper recently published in the open-access journal Energies, researchers reviewed and summarized published articles to determine the most promising approach for artificial intelligence (AI) applications in environmental and energy engineering systems.
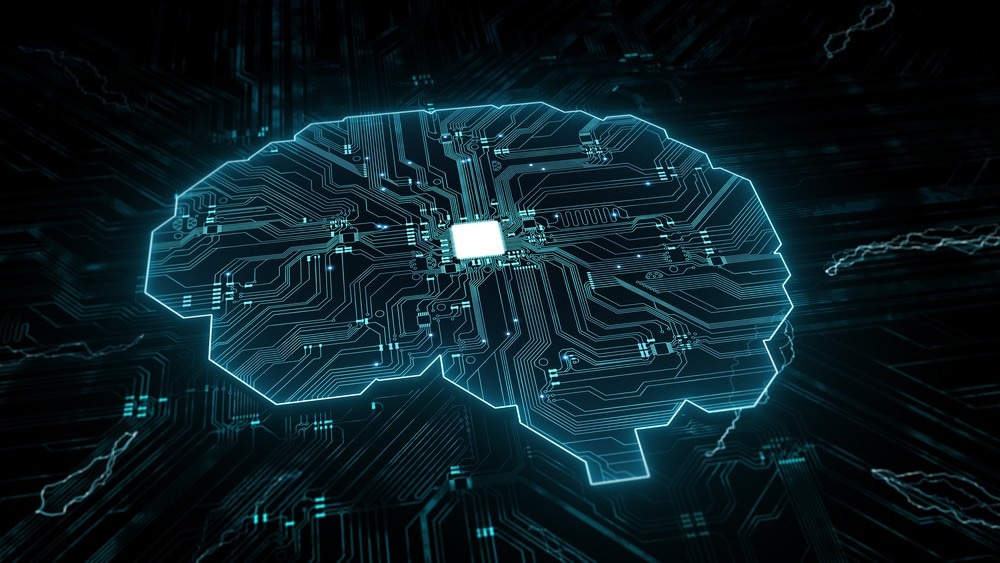
Study: Advanced AI Applications in Energy and Environmental Engineering Systems. Image Credit: cono0430/Shutterstock.com
AI and its Applications
AI is a computer science tool that works on creating intelligent devices, machines, and systems that carry out operations akin to human decision-making and learning. It can comprehend external data and learn from it, and adapt to its learning with practice.
Combining AI with the internet of things (IoT) technologies could be another promising approach as this combination can harvest large amounts of data, and the AI can study data patterns to enable task automation for several business benefits. Numerous uses of IoT systems in the energy sector pertain to sensor and communication technologies involving light sensors, temperature, humidity, speed, proximity, and passive infrared.
AI has been widely utilized in agriculture, focusing particularly on rice diseases, crop and pest management, product monitoring, and yield prediction. Medical and healthcare applications of AI include the understanding of diseases such as cancer as well as brain and heart disorders.
The Need for AI in Renewable Energy Sources (RES)
Although there have been numerous advancements in the use of RES, there is a considerable amount of scope regarding the use of intelligent and smart systems such as AI. This application can provide solutions to the existing and evolving difficulties and opportunities in the maintenance, power management, and control of RES.
Recently, RES has been receiving integration of AI and IoT, which has helped technologies such as wind turbines, solar photovoltaics (PVs), and panels become more efficient and cost-effective. AI technologies are currently being used in all RES including wind, ocean, hydro, and solar PVs, thus providing the potential to overcome the dependence on power generation based on fossil fuels.
AI Techniques in Energy Systems
The different AI techniques implemented in RES include artificial neural network (ANN), adaptive neuro-fuzzy inference system (ANFIS), genetic algorithm (GA), and automated machine learning (AutoML).
Among the above techniques, ANN is advantageous owing to its higher accuracy, generalization capabilities, and short calculation time. A study by Ashraf et al. outlined an advanced method for using actual operational data and implementing ANN methodologies in a supercritical coal-fired plant with a capacity of 660 MWe. Other ANN applications were depicted in a study by Skrobek et al., which consisted of sorption modeling through the deep learning (DL) method.
Furthermore, ANFIS has been successful as a data-driven, adaptive computational technique that is capable of plotting multifaceted and non-linear data. This has enabled the implementation of ANFIS in exergy and energy analyses as well as thermodynamic parameter predictions. On the other hand, GA is an appealing approach to optimization and modeling of environmental and energy systems, as seen by the use of this model in gene expressions programming (GEP). GEP can devise a mathematical expression among the independent and dependent variables that functions correctly for all cases regarded as an adaptive algorithm. GEP is also established as a capable system useful in detecting incipient defects inside power transformers.
To summarize, the authors considered all approaches and determined that AutoML could be a capable method for environmental and energy engineering system applications. Since the accessibility of machine learning is made possible by AutoML, it may become a potential alternative for several other data management techniques.
References
Krzywanski, J. Advanced AI Applications in Energy and Environmental Engineering Systems. Energies 2022, 15, 5621. https://doi.org/10.3390/en15155621
Dellosa, J.T.; Palconit, E.C. Artificial Intelligence (AI) in Renewable Energy Systems: A Condensed Review of Its Applications and Techniques. In Proceedings of the 2021 IEEE International Conference on Environment and Electrical Engineering and 2021 IEEE Industrial and Commercial Power Systems Europe (EEEIC/I&CPS Europe), Bari, Italy, 7–10 September 2021; pp. 1–6. https://www.academia.edu/62001720/Artificial_Intelligence_AI_in_Renewable_Energy_Systems_A_Condensed_Review_of_its_Applications_and_Techniques?auto=citations&from=cover_page
Ashraf, W.M. et al., Optimization of a 660 MWe Supercritical Power Plant Performance,a Case of Industry 4.0 in the Data-Driven Operational Management Part 1. Thermal Efficiency. Energies 2020, 13, 5592. https://doi.org/10.3390/en13215592
Skrobek, D, et al., Prediction of Sorption Processes Using the Deep Learning Methods (Long Short-Term Memory). Energies 2020, 13, 6601. https://doi.org/10.3390/en13246601
Disclaimer: The views expressed here are those of the author expressed in their private capacity and do not necessarily represent the views of AZoM.com Limited T/A AZoNetwork the owner and operator of this website. This disclaimer forms part of the Terms and conditions of use of this website.