A paper recently published in the journal Electronics Letters demonstrated the feasibility of using a novel privacy-preserving blockchain-enabled framework for handover skipping in a high-mobility train network.

Study: Blockchain-enabled handover skipping for high mobility train passengers. Image Credit: CHEN MIN CHUN/Shutterstock.com
Background
The rising demand for data traffic due to the constant use of the mobile network has resulted in the development of small cells (SCs). SCs form a dense network that addresses the network capacity needs of daily train passengers in a high-mobility train network.
However, frequent and unnecessary handovers in such networks due to short journey times and reduction of cell sizes lead to loss of communication, which can adversely affect the rate of data transmissions and the overall quality of service.
In ultra-dense networks, tracking and monitoring the passenger trajectories to maintain a good quality of service with intelligent handover management, reduced costs, and enhanced throughput is considerably difficult as limited resources are available for fast-moving train passengers.
Passenger movements analyzed using conventional mobility prediction models are used as input in machine learning (ML) to establish the best-chosen daily routes. However, these models do not consider handover delay tolerance and last-hop signal strength.
Intelligent handover processes can ensure that passengers remain within the service footprints throughout their trajectories to prevent the wastage of resources. For instance, smart handover management can be implemented considering estimated user trajectories and topology awareness.
In smart handover management, the handover skipping decisions are made based on the cell sizes and cell locations to identify the closest handover and base station of the users. However, most of the handover skipping schemes require user data collection, which is increasingly becoming challenging due to the growing awareness of privacy among users.
Traditional user tracking applications, such as the Trace Together App and Google Apple Contact Tracing, have several drawbacks, including high risk to user privacy, due to high dependence on third-party and central servers for notifications and contact matching.
Thus, an innovative approach is required to ensure the secure and anonymous collection of data from users with their consent. Blockchain decentralized data management frameworks are considered to be promising alternatives as they are less vulnerable to failures, more transparent, anonymous, more secure, and do not depend on central servers.
The framework ensures user privacy using pseudonym addresses and data encryption methods.
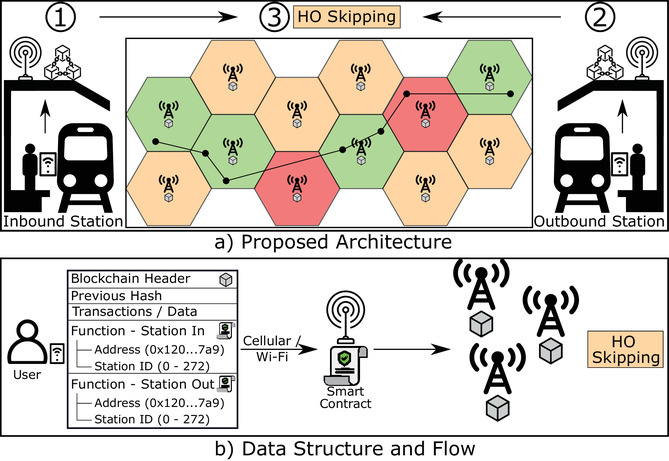
Proposed blockchain HO skipping architecture, data structure, and flow. A specific train line in Westbound direction is considered as a use-case. Image Credit: Hussain, S et al., Electronics Letters
The Study
In this study, researchers proposed a novel blockchain-enabled privacy-preserving handover skipping framework using the train mobility dataset from the city of London. The passenger traffic flows were modeled based on a complex dataset by averaging the station’s footfall numbers and different train lines using blockchain to ensure privacy. In the framework, pseudonym addresses were stored to track and monitor the user trajectory.
System Model
The proposed framework considered a downlink stream with several base stations dispersed over the London overground and underground (LOU) network. A specific train line in the westbound direction in a particular rectangle-shaped geographical area with L1 and L2 sides surrounding the LOU train network was considered as the use case.
Base stations were deployed uniformly with λ rate in the rectangular area as per Poisson point process (PPP) mobility. Every base station possessed a specific bandwidth and transmit power. Moreover, the framework was also composed of predefined train speed v and S stations, where users u can leave/board the train.
Passenger modeling was based on the distance of the passengers from the base stations surrounding the train network in the framework. All passengers, either alighting at stations or in train carriages, were represented as U = 1, 2, . . . , u with base stations as B = 1, 2, . . . , bk for a user association rule.
Reference signal received power (RSRP) measurements were performed by identifying the location of passengers in the train and their association to the nearest e-NodeB (eNB) base station.
Signal-to-interference and noise ratio (SINR) was used to measure the average RSRP sum of passengers to the average interference sum of noise. Users were allocated to the base station with the best SINR based on the SINR measurements.
Handover costs associated with handover skipping throughout the train route were calculated as the product of train speed, delay tolerance of every handover in seconds, and handover rate per unit of time. Researchers also measured the average passenger throughput considering the impact of handover skipping and handover rate.
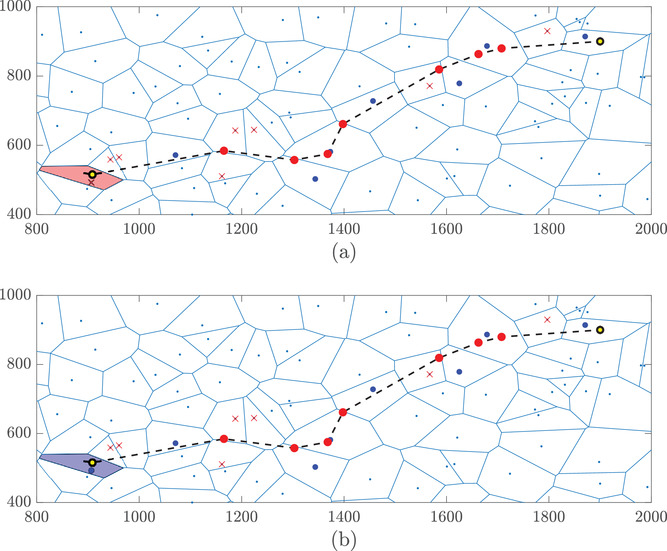
Simulated scenario shows the set of BSs skipped and connected for (a) the hybrid approach, and (b) the proposed approach. Image Credit: Hussain, S et al., Electronics Letters
Significance of the Study
The novel handover skipping framework effectively tracked the individual users through train stations in a high-mobility train network using the blockchain methodology. In this framework, the downloaded mobile application in the smartphone of passengers automatically interacted with the stations’ access point when they entered a train station and sent some basic information, including pseudonymous addresses of passengers that conceal their identities and their inbound/outbound stations.
Every base station was considered a blockchain node in the framework for information exchange and communication. The collected user information was readily available at all base stations after the blocks were validated by the blockchain, which was then used for individual handover skipping decisions for every passenger.

Estimated blockchain storage and transactions per second needed. Image Credit: Hussain, S et al., Electronics Letters
Thus, the blockchain provided end-to-end security throughout the passenger trajectory and was not utilized in all steps of the handover process. A step-by-step handover process was also executed with an additional blockchain security layer.
The proposed handover framework acted as a private and secure platform for train passengers while analyzing their future directions and mobility predictions by collecting their data with their consent throughout the entire journey. Specifically, the proposed framework ensured maximum privacy while observing the passenger trajectory considering the passenger locations and traveling paths as well as cell sizes.
User-specific handover skipping was achieved successfully based on individual user information due to the deployment of blockchain framework over the existing handover skipping, leading to a better trade-off in terms of last-hop signal quality, user quality of service, and network handover cost. The framework increased average throughput by 2% and last-hop signal quality by 100% and reduced the network handover costs by 50%.
References
Hussain, S., Asad, S. M., Imran, M. A., et al. (2022) Blockchain-enabled handover skipping for high mobility train passengers. Electronics Letters. https://ietresearch.onlinelibrary.wiley.com/doi/10.1049/ell2.12658
Disclaimer: The views expressed here are those of the author expressed in their private capacity and do not necessarily represent the views of AZoM.com Limited T/A AZoNetwork the owner and operator of this website. This disclaimer forms part of the Terms and conditions of use of this website.