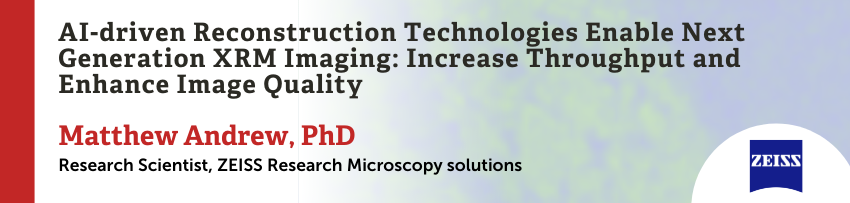
Watch this webinar titled, AI-driven Reconstruction Technologies Enable Next Generation XRM Imaging: Increase Throughput and Enhance Image Quality on-demand now.
About this webinar
In this webinar, you will learn how some of the biggest challenges associated with X-ray microscopy are tackled with the help of Artificial Intelligence (AI)-driven algorithms.
The first technology which enables a completely automated training of deep learning networks for reconstruction, ZEISS DeepRecon Pro, allows for images to be reconstructed at greatly reduced artefact and noise levels relative to traditional analytical reconstruction techniques. This can be used to either improve image throughput up to 10X or image quality while keeping throughput constant.
A second new technology allows for the quantitative removal of propagation phase contrast artefacts from X-ray microscopy images. ZEISS PhaseEvolve reveals the inherent material contrast of the image, otherwise overprinted by phase effects. And it allows for more accurate quantitative segmentation and analysis.
The application of these techniques on a range of samples from a range of application fields will be discussed, including rock cores, batteries, electronic devices and pharmaceutical samples.
Learn how:
- The easy-to-use solutions allow for models to be trained on X-ray datasets with a single click, without the need for a deep level of expertise in Deep Learning technologies
- Throughput is improved by up to 10x
- Image quality is greatly enhanced
- Phase effects are removed, allowing for much easier and more accurate segmentation
About the event speaker
This web seminar will be led by Matthew Andrew, Ph.D., a scientist currently working with ZEISS Microscopy who is passionate about developing new technologies and workflows within microscopy. Although this involves utilizing and integrating a wide range of different technologies, his current research is focused on the development and application of new reconstruction technologies for X-ray microscopy, particularly using deep learning.
Dr. Andrew received his Ph.D. from the Department of Earth Science and Engineering at Imperial College, London. He has published extensively on these topics, including his Ph.D. dissertation, ''Reservoir-Condition Pore-Scale Imaging of Multiphase Flow''.