In cold regions, ice accumulation is a major issue that hinders the efficient operation of wind turbine blades. A new paper has been published in the journal Energies with the aim of evaluating a novel method for detecting blade icing. The research has been conducted by scientists from the School of Electrical Engineering at Yanshan University in China.
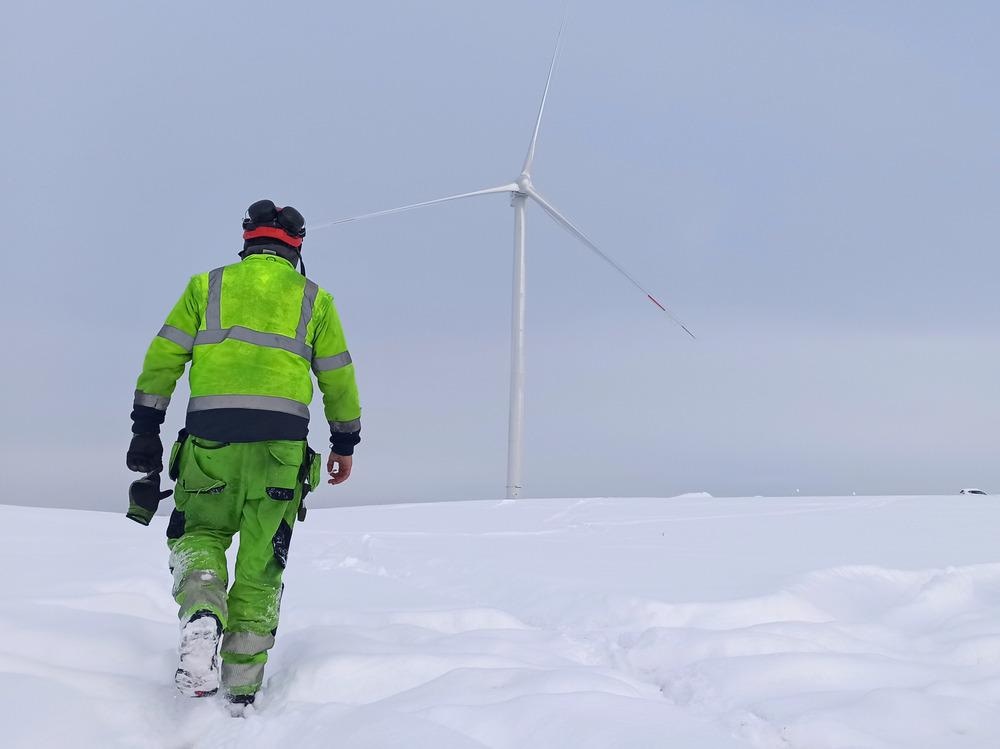
Study: Detecting Wind Turbine Blade Icing with a Multiscale Long Short-Term Memory Network. Image Credit: ka100500/Shutterstock.com
The Dangers of Blade Icing
Wind turbines have become a key renewable energy technology in the push toward a carbon-neutral economy. To maximize their energy generation capabilities, many onshore wind turbines are placed in areas with high elevations, such as the northern regions of China. However, installing wind turbines in these areas exposes them to freezing conditions, increasing the risk of ice formation on turbine blades.
Ice formation can lead to damage of turbine components if ice is not removed promptly. Structural stress causes mass and aerodynamic imbalance, which leads to lower wind power generation and shortened blade lifespan. Critical failures in turbine components such as bearings can lead to major accidents. Therefore, early, accurate detection of icing incidents is essential and helps to trigger the turbine’s ice removal systems.
Conventional Icing Detection Methods
Currently, there are several methods employed to detect blade icing and trigger timely de-icing interventions to avoid damage to blades and turbine components. Commonly employed conventional methods include telescope detection, infrared scanning, and UAVs. Vibration, wave propagation, and acoustic emission methods are used to monitor the health of blades.
![Schematic diagram of an LSTM cell at time step t [23]. Note that the output yt equals to the hidden state output ht.](https://d12oja0ew7x0i8.cloudfront.net/images/news/ImageForNews_58845_16503591176698396.jpg)
Schematic diagram of an LSTM cell at time step t. Note that the output yt equals to the hidden state output ht. Image Credit: Wang, X et al., Energies
There are drawbacks to these techniques, however. They cannot be carried out during periods of operation, and additional equipment such as sensors and cameras are needed, increasing costs. Moreover, equipment problems such as sensor degradation can impact detection accuracy, thereby reducing the effectiveness of detection methods. Reliable and inexpensive methods which provide early detection of icing events are needed by the wind turbine industry.
SCADA methods have emerged recently as a low-cost and reliable alternative to conventional ice detection techniques. These methods collect status and operational data, which include information on critical mechanical, electrical, and environmental parameters that relate to turbine health status. SCADA methods have been used to monitor component conditions, and they are increasingly becoming a standard configuration in large-scale wind turbines.
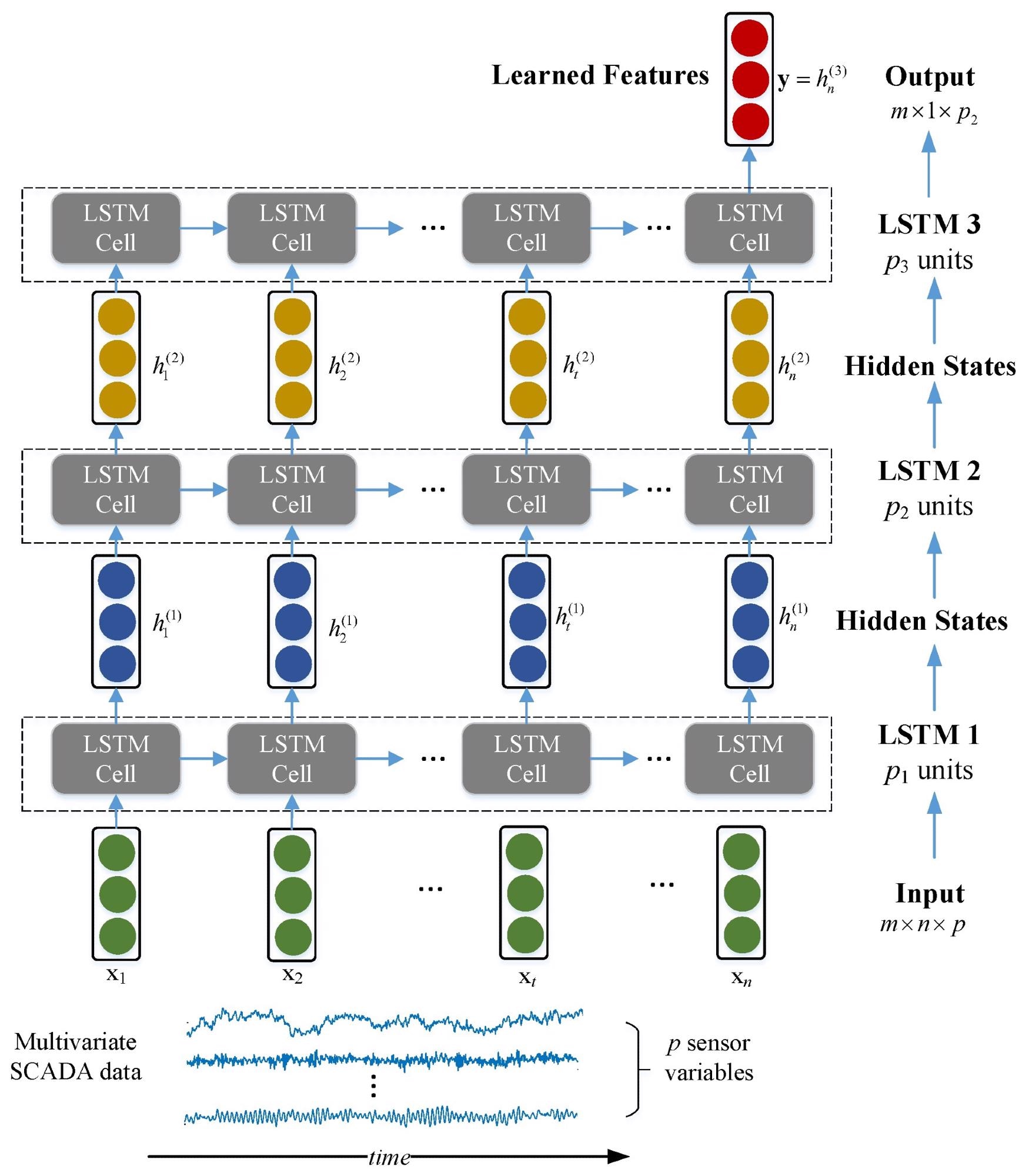
A three-layer deep LSTM architecture for temporal feature learning with multivariate time series. Here, p1, p2, p3 denote the number of units for three LSTM layers. Image Credit: Wang, X et al., Energies
Several studies have explored the potential of SCADA as a method for accurate and reliable low-cost detection of icing events. Whilst more reliable, there are limitations to these methods. Data gathered using SCADA methods lack measurements such as mechanical loads and blade vibrations, which are essential for monitoring the health status of turbine blades. Moreover, features still have to be extracted manually, and methods lack mature machine learning techniques. Limited modeling abilities hinder the satisfactory performance of these methods.
The Paper
The new research has investigated the development of an economical and robust system for detecting blade icing. The novel model designed in the research is based upon SCADA methods. To overcome the issues with traditional SCADA methods, the team has investigated incorporating deep learning models into the proposed system.
Deep learning and neural networks have already been widely studied in the wind turbine industry, with different papers applying techniques and models for fault diagnosis and monitoring. The gathering of data using these methods is strongly dependent on the temporal nature of sensory inputs, and external environmental factors can impact the data. Furthermore, data gathered are non-stationary due to the movement of turbines by the wind. Noise and disturbances are common features of data gathered using SCADA.
The authors have developed a new system which has been termed WaveletLSTM. Incorporating wavelets into the system gives it a multiscale learning ability. The system has a high level of discriminability and possesses the ability to analyze information from both local and global data and learn from it. Real-world SCADA data was used to evaluate the proposed model and demonstrated the superior fault-detection ability of WaveletLSTM.
The proposed model performed better than several other machine learning techniques. Moreover, expert knowledge is not required by the design. The authors have stated that this novel icing event detection model has potential as a system for detecting faults that can be used in multiple industrial applications. Further training and adjustment in the cloud could be used for further practical wind-farm applications.
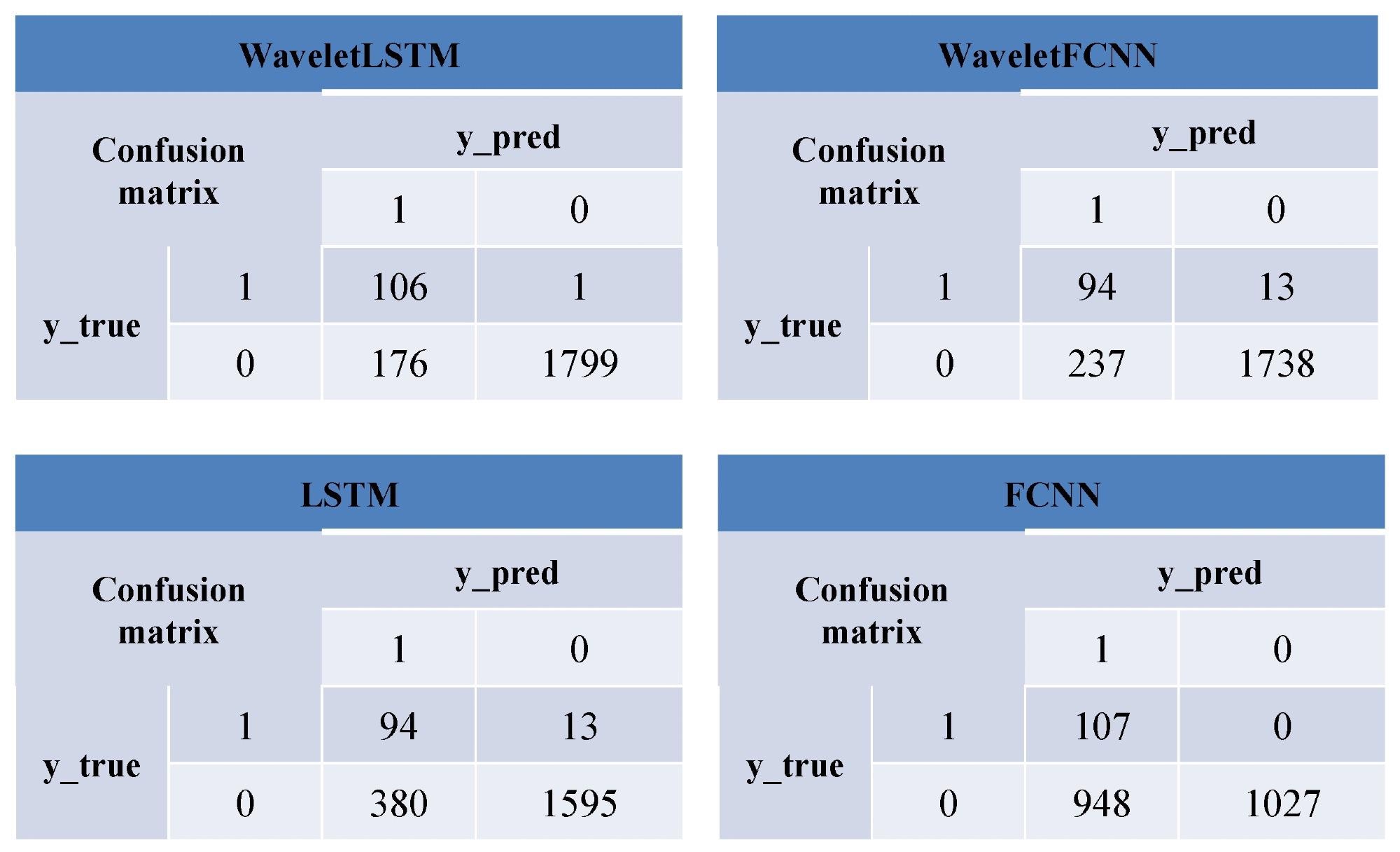
Confusion matrix of different models. Image Credit: Wang, X et al., Energies
The focus of future work by the authors will include the investigation of advanced imbalanced learning methods to develop more robust and effective fault detection systems. It has been noted that a drawback to the model is its inability to detect icing in advance; therefore, another focus will be on exploring the potential for timely interventions for early fault detection.
Further Reading
Wang, X et al. (2022) Detecting Wind Turbine Blade Icing with a Multiscale Long Short-Term Memory Network [online] Energies 15(8) 2864 | mdpi.com. Available at: https://www.mdpi.com/1996-1073/15/8/2864
Disclaimer: The views expressed here are those of the author expressed in their private capacity and do not necessarily represent the views of AZoM.com Limited T/A AZoNetwork the owner and operator of this website. This disclaimer forms part of the Terms and conditions of use of this website.