Throughput integrated with image quality is considered one of the biggest difficulties linked to X-Ray microscopy. Obtaining high-resolution images—especially within huge samples—needs long acquisition times.
Such long acquisition times might restrict the technology’s application to industrial processes, where time per sample is crucial, or when executing in situ experiments where high temporal resolution is needed.
ZEISS DeepRecon increases image quality and efficient acquisition time via the integration of neural networks into the reconstruction process and image acquisition. Through working in close association with customers, networks and workflows can be tailored to answer particular difficulties linked to repetitive samples.
Using this technology could enhance throughput by up to a factor of 10, thereby significantly enhancing image quality and decreasing the effect of imaging artifacts that normally tend to impact quick acquisition workflows.
Highlights
Area of Research:
- Geoscience
- Semiconductor
- Manufacturing
Sample Types:
- Electronics
- Geological samples
- Semiconductor packages
Related Solutions
ZEISS Phase Contrast Enhancer
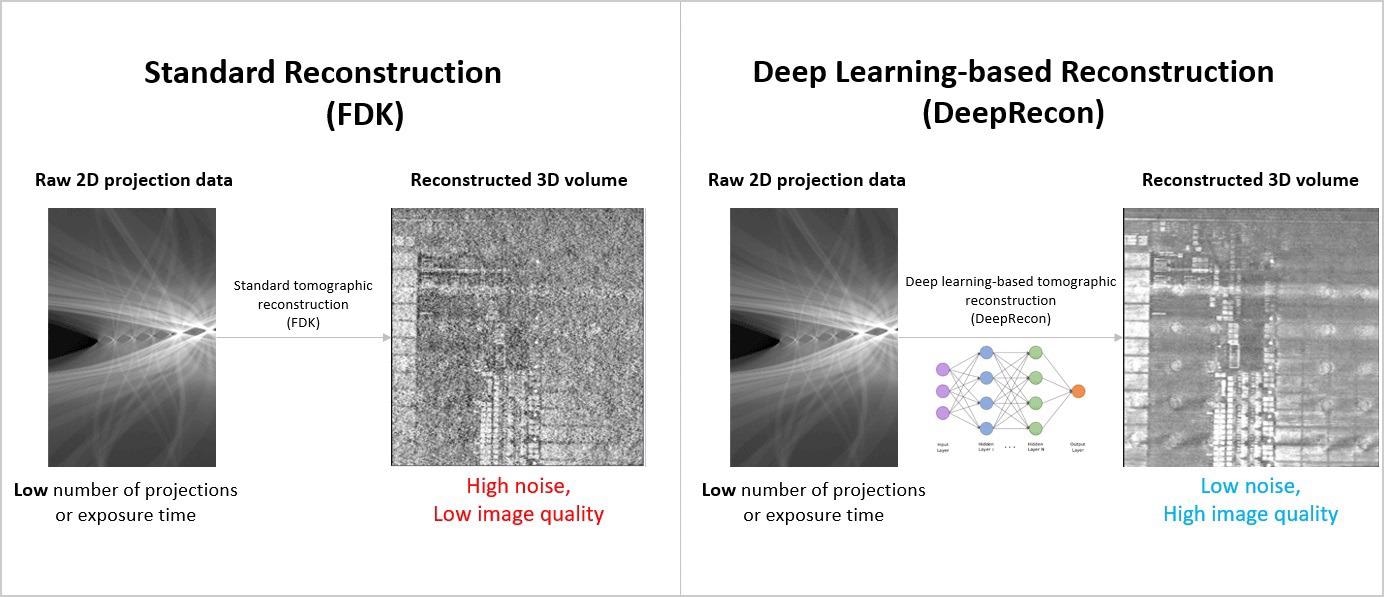
Deep learning-based image quality improvement with higher throughput for Xradia X-ray microscopes. Image Credit: Carl Zeiss Microscopy GmbH
The neural network-based image quality improvement structure has been obtained by training networks to recover image data degraded via noise, limited projection numbers, or samples outside of the field of view.
Workflow
The module workflow comprises a few steps:
- ZEISS DeepRecon is a Special Customer Solution (SCS), tailored to meet the particular needs of their use-case and sample class. Initially, engage with users’ local ZEISS representative (or fill out a form) to initiate the discussion regarding getting DeepRecon at the facility.
- Users can send data or a sample to the ZEISS XRM team.
- This data is utilized to make a tailored DeepRecon model, allowing excellence
- This model has been loaded into a tailored installation of ZEISS Reconstructor.
- Obtain data under the discussed conditions (up to 10X throughput boost comparative to standard reconstruction). The tailored model will be a selectable option at the time of sample reconstruction.
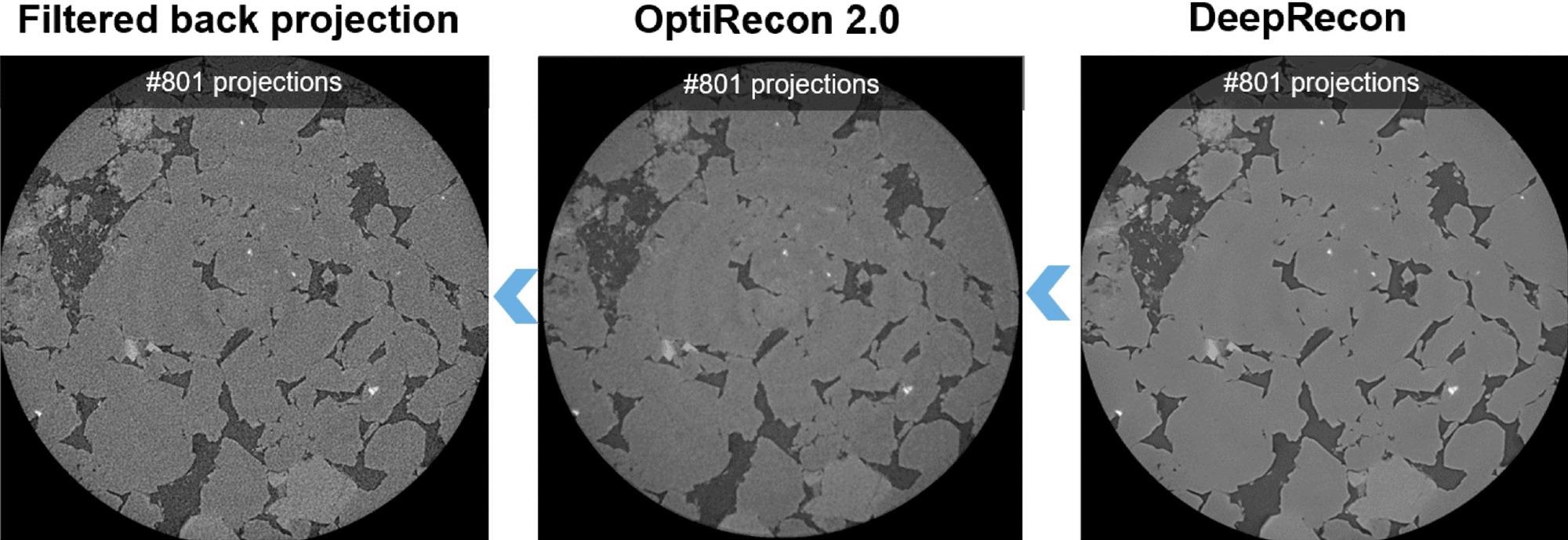
Progressive image quality improvement with various reconstruction techniques for Xradia X-ray microscopes. Image Credit: Carl Zeiss Microscopy GmbH
The effect of varying reconstruction methods on a sandstone sample, displaying gradual image quality improvement from standard reconstruction (filtered back projection) to OptiRecon 2.0 (iterative reconstruction) and DeepRecon (neural network-based reconstruction).