A comparative study has been published in the journal Sustainability exploring different online algorithms which estimate the state of charge (SOC) in lithium-ion batteries. Scientists from Germany, India, Malaysia, Australia, and Saudi Arabia have contributed to the research.

Study: A Comparative Study on Different Online State of Charge Estimation Algorithms for Lithium-Ion Batteries. Image Credit: Lightboxx/Shutterstock.com
SOC Estimations
Although technology is expanding in electric vehicle development, it is still challenging to manufacture safe and efficient energy storage components. Lithium-ion batteries possess beneficial properties such as high energy density, low self-discharge, and long cycle lives.
SOC estimations are extremely important for lithium-ion batteries. Accurately evaluating a device’s SOC prevents critical issues such as overcharges, thermal runaways, and deep discharges. Lithium-ion batteries have become a key technology in the shift from internal combustion energies in the automotive industry to address climate change issues, and evaluating SOC is critical for safety and performance.
Battery management systems are recommended for ensuring that batteries operate in a safe range. These are electronic chips that are fast becoming an industry standard. Battery state, such as SOC, needs to be accurately monitored to ensure the proper functionality of battery management systems.
SOC is a ratio of the battery’s remaining to actual capacity. Algorithms are employed in battery management systems to monitor SOC, as it cannot be directly measured.
Estimation Methods
SOC estimation methods are classified as both indirect and direct methods. Direct methods are the open-circuit voltage and ampere-counting methods. Whilst these are easy to implement in battery management systems, errors can occur that limit SOC estimation.
Non-direct methods are both data-driven and model-based methods. Several model-based methods have been developed, including ECMs, EMs, EECMs, and ECTMs. Various advanced algorithms have been developed in recent years to provide accurate SOC estimation capabilities, such as Kalman filters, particle filters, the Luenberger observer, and the sliding mode observer.
To further enhance model-based estimation methods, modified algorithms have become a focus of research, including extended KF, unscented KF, and modified LO. Data-driven methods commonly use machine learning algorithms such as feedforward neural networks and recurrent neural networks. A major limitation of these machine learning methods, however, is the quality and quantity of datasets.
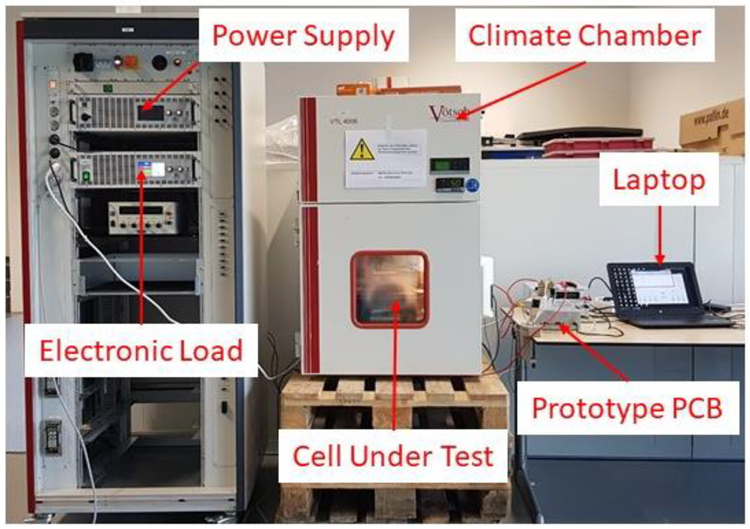
Experimental setup. Image Credit: Khan, Z.A et al., Sustainability
The Study
The new paper in Sustainability has reviewed the current state-of-the-art in this research field. Over the past decade, studies related to SOC estimations have doubled to just over a thousand papers published in 2021. This confirms that the research area is receiving growing attention from scientists.
Many researchers have focused on model-based SOC estimation due to the accuracy of the methods. In respect to adaptive algorithms, Kalman filters have been researched more frequently than other types. Data-driven methods have also received increased research attention due to recent developments in machine learning.
The authors have noted that to provide detailed comparative assessments the same test environment must be considered for SOC estimation and algorithms. This is because estimation may vary depending upon real-world operating conditions.
Seven different algorithms have been evaluated in the study based on recent research trends. These are LO, EKF, SMO, AEKF, UKF, BPNN, and LO. Each algorithm was evaluated under the same operating conditions to provide an accurate comparison in terms of complexity and SOC estimation error.
The authors have also analyzed the effects of temperature on model parameters for two RC batteries, utilizing the information for model-based SOC estimation methods. Experimental datasets from tests on commercial lithium-ion-based prismatic cells were used for SOC estimation. Different algorithms were applied to the datasets. Additionally, the authors compared computational burden and estimated state-of-charge accuracy.
Study Findings
The study’s findings can be used to provide future recommendations for SOC estimation. Furthermore, these findings can be utilized for the study of other operational and device states and parameters.
Comparing estimation results, the authors recommended that, for real-time battery management implementation, SMO and EKF are the most suitable algorithms. The lowest estimation errors and the least computation time were achieved using EKF, whereas SME possesses the highest computation time but the lowest estimation errors. Furthermore, the authors confirmed the superior accuracy of the 2-RC battery model.
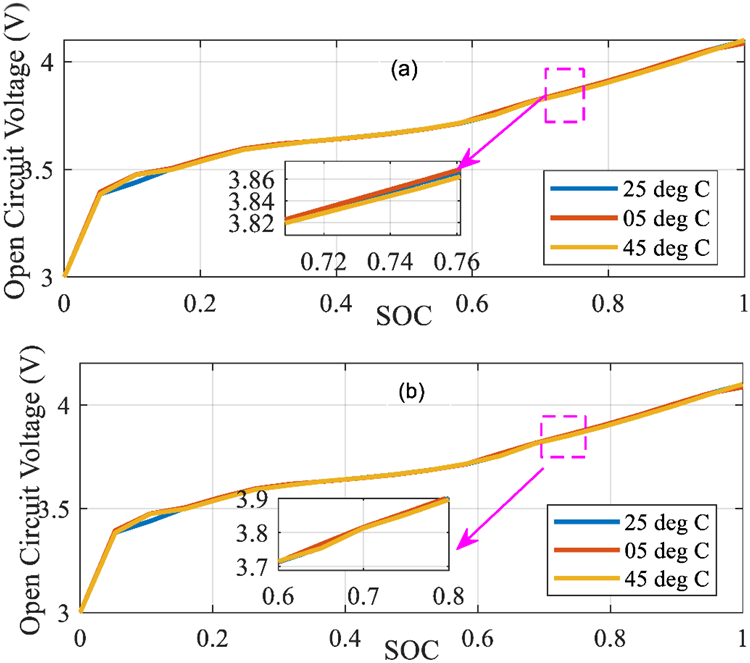
Obtained OCV vs. SOC relationship under three different temperatures: (a) for charging (b) for discharging. Image Credit: Khan, Z.A et al., Sustainability
Future Perspectives
Several recommendations were made in the study for future research directions. These include using model-based methods to improve SOC estimation accuracy by simultaneously estimating parameters, reducing the computational burden and improving accuracy, combining data-driven and model-based methods, and creating hybrid models to enhance the accuracy of online estimation methods.
Other recommendations include developing digital twins and cloud-based battery management systems. Whilst there are still some challenges to be addressed with online SOC estimation, the field is a promising one that can improve the commercial viability, safety, and performance of lithium-ion batteries for sectors such as the automotive industry.
Further Reading
Khan, Z.A et al. (2022) A Comparative Study on Different Online State of Charge Estimation Algorithms for Lithium-Ion Batteries Sustainability 14(12) 7412 [online] mdpi.com. Available at: https://www.mdpi.com/2071-1050/14/12/7412
Disclaimer: The views expressed here are those of the author expressed in their private capacity and do not necessarily represent the views of AZoM.com Limited T/A AZoNetwork the owner and operator of this website. This disclaimer forms part of the Terms and conditions of use of this website.